Month: November 2020
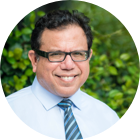
MMS • RSS
Article originally posted on Data Science Central. Visit Data Science Central
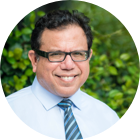
MMS • RSS
Article originally posted on Data Science Central. Visit Data Science Central
In my previous post, What’s Driving the Future of Work and Professions?, I mentioned PESTLE as a helpful framework for classifying external forces that act on all of us and the companies for which we work. In this article, I present a list of them for you to consider. It’s not encyclopedic, but it’s a good start.
As you do, ask yourself:
- What was the old norm?
- What is the new norm?
- What does a person need to thrive in the new norm?
To remind you PESTLE stands for Political, Economic, Social, Technological, Legal and Environmental. Each of these areas is addressed, below:
- Political
- COVID impacts, countries’ responses and the economic fallout
- Healthcare issues—costs, availability
- Wealth inequality and redistribution, differences in educational opportunities
- Cross-border trade and supply chains, protectionism vs. globalism
- Urban, suburban, rural differences and partisan polarization, adversarial government and the inability to push forward legislative action
- Climate change and denial, green initiatives, energy-related tax incentives and policies
- Immigration policy discord and changing demographics, mass migrations, differences in birth rates
- Overpopulation, impact on the ecosystem and strain on quality of lives
- Chinese, Russian, Iranian and Saudi aspirations
- New political systems, decline of Liberalism, nationalism, protectionism
- Asymmetric and Cyber warfare, Tech cold war, foreign social media influence and election tampering, data privacy and security
- Economic
- Productivity increases, quality of life improves, poverty decreases but inequality increases; perceived justice issues spur distrust and unrest
- Geo-economic issues, money flows, globalism, rise of the 3rd world
- National and individual debt
- Population growth, fewer opportunities, differences in birth rates, greying of populations
- Impacts of COVID, climate change
- Remote Work, decline of cities, strain on food, restaurant, sports, travel & entertainment industries; increasing gig and contracting work
- New jobs and forms of competition and cooperation will emerge, robotics displaces or permanently alters massive number of jobs; new entrepreneurial opportunities emerge
- Social
- Impact of social media, loss of social cohesion, disinformation runs rampant, social unrest; increasing numbers of people believe blatantly false information
- Resentment and anger, tension, differences in expectations, inequality of wealth and opportunities, politics of grievance
- Norms, such as religious beliefs, shatter; new sects emerge (e.g., QAnon)
- Loss of privacy, risks of mega companies
- COVID restrictions disrupt in-person events of all sorts, COVID deaths
- Work from anywhere, new forms of management, new definitions of work
- End of or disruption in careers, diminishing work opportunities, end of institutions, end of elites
- Education- higher education business model disrupted, companies will offer education opportunities as a perk or an inducement, lifelong learning will be mandatory
- Technology: SMACIT—social, mobile, analytics, cloud and Internet of Things, and others
- Social Media displaces face to face interactions, accelerates fads and trends; AR/VR will make its way into business and social interactions
- Mobile, anywhere, anytime connectivity pushes the merging of work and personal lives. Work from anywhere will create arbitration opportunities for hiring companies to drive down pay scales for contractors and employees
- AI drives decision-making and displaces what were formerly human interactions; it increasingly enables and augments on-line marketing and selling, among other things
- Cloud becomes the foundational infrastructure for everything and a delivery mechanism for services.
- IoT will flood the information ecosystem with real-time data. Things like healthcare and package delivery will evolve substantially.
- Robotics—Anything physical that can be automated will be automated. Manufacturing jobs will diminish, though a higher level of employee that can program, manage and service robots will emerge. 3D printing will displace manufacturing and assembly processes. Software robots will process an increasing percentage of day-to-day transactions. Increasingly smart AI will drive out the need for human decision-making for everyday things.
- Blockchain will become the foundational transaction processing substrate for interactions among governments, companies, NGOs and people.
- Software development—What’s now done by programmers will increasingly be generated autonomously.
- Apps for everything—interactions between customers and providers (companies, government, NGOs, others) will be displaced by apps.
- Legal
- Political polarities and power politics will impair the ability to evolve laws at the rate that society is changing, laws on privacy and data protection, in particular. All-or-nothing ethos will prevail.
- International laws will evolve unevenly, power will shift as alliances do
- Legal mechanisms are perceived as unfair, existing mechanisms for consensus cease working
- The filter for decision-making and problem solving will increasingly be risk.
- Rise and risks of global elites will provoke responses and engender legislation
- Ageism becomes an increasing problem as the population ages.
- Technology will increasingly be incorporated into legal interactions—blockchain, smart contracts and AI, in particular.
- Environmental
- Climate change has profound impacts, making some areas inhabitable while making others habitable. Food production may become impossible in many areas. The costs of some food products may soar. Others may simply disappear.
- Carbon-producing energy and power-consuming industries and equipment will get displaced over time—electric vehicles will displace internal combustion vehicles
- Dirty industries that pollute 3rd-world countries will be forced to curtail their activities to a large extent. Internet-enabled piece work alternatives to manual labor done by local populations may change the economic trade-offs on such operations.
- Provenance of goods will be much more widely and closely tracked and the information may impact a lot of international trade. Supply chain monitoring automation will proliferate.
Now, give some thought to the following questions about your chosen profession:
- What’s your reaction to this list? Is anything missing that is relevant to your profession?
- What drives the business model of your profession?
- What major changes have you seen over the past few years and do you see coming in the next few?
- Which of them do you think will be the most impactful? In the near-term? In the longer term? Which will be most difficult to navigate and why?
- How do you think your profession will have to change to adapt?
- What advice would you give to anyone contemplating entering or remaining in the profession?
We have enlisted the help of some noted practitioners in a number of professions and gotten their answers to these questions. We will be posting these interviews over the next several weeks.
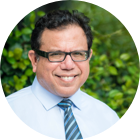
MMS • Ron Nevo
Article originally posted on InfoQ. Visit InfoQ
AIOps platforms empower IT teams to quickly find the root issues that originate in the network and disrupt running applications. AI/ML algorithms need access to high quality network data to determine what went wrong and where. Network visibility starts from TAPs around network equipment, and teams can add application instrumentation and logs as data sources for complete insights.
By Ron Nevo
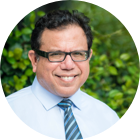
MMS • Bruno Couriol
Article originally posted on InfoQ. Visit InfoQ
The Electron team recently released the 11th iteration of the popular cross-platform native desktop application framework. Electron 11 introduces support for the new Apple silicon hardware (Darwin arm64). Electron 11 also updates the versions of Chromium, Node, and its JavaScript engine.
By Bruno Couriol
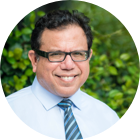
MMS • RSS
Article originally posted on Data Science Central. Visit Data Science Central
I’ve been reading the interesting and soul-searching (from a data scientist perspective) book from Cathy O’Neil titled “Weapons of Math Destruction”, or WMD as used in the book. The book provides several real-world examples of how Big Data and Data Science – when not properly structured – can lead to ethically-wrong unintended consequences.
Chapter 3 “Arms Race: Going to College” describes how the college ranking system developed by “US News & World Report” in 1983 has created its own self-fulfilling, mis-aligned ecosystem. Because of the influence the “US News & World Report” ranking has on the multi-billion-dollar college recruiting business, a few key metrics – SAT scores, student-teacher ratios, acceptance rates, alumni donations, freshman retention – get over-valued in college’s investment strategies.
The unintended consequences is that many colleges focus their investments on overly-opulent facilities and over-paid research faculty programs in the effort to increase their ranking, sometimes at the expense of a more holistic “quality education and enlightening personal experience” for the college students.
But the “US News & World Report” ranking is greatly flawed with the omission of several critical key metrics. For example, the ranking doesn’t considered price. If cost is not an issue for someone deciding to go to college, then that’s okay. But for the other 99% of us, cost is an important factor in determining a “quality” educational experience.
And that’s the challenge with AI model biases, if you don’t carefully consider the different variables and metrics against which you need to measure model progress and success, you may end up with AI models that deliver ethically-wrong unintended consequences.
So, how does one mitigate the negative impacts of models that are supposed to represent the real-world, but actually provide a dangerously biased and skewed perspective on that world? Here are a couple of things that every organization can do to reduce the ethically-wrong unintended consequences caused by AI models that turn into ““Weapons of Math Destruction”:
- Brainstorm a “diverse, sometimes conflicting set of metrics” across a diverse group of stakeholders that drives the AI Utility Function
- Thoroughly explore and quantify the AI model costs of False Positives and False Negatives
#1. Brainstorm Diverse Set of Metrics to Power AI Utility Function
One way to avoid AI models that deliver unintended consequences is to invest the time upfront to brainstorm a “diverse, sometimes conflicting set of metrics” against which the AI model will seek to optimize. This means embracing a diverse set of stakeholders (a stakeholder map can help to identify the different stakeholders who either impact or are impacted by the AI model) who can provide a diverse set of perspectives on how best to measure the AI model’s progress and success.
To understand why it’s important to capture a diverse and sometimes conflicting set of metrics against which the AI model must seek to optimize, one needs to understand how an AI model (AI Agent) works (see Figure 1):
- The AI model relies upon the creation of an “AI Agent” that interacts with the environment to learn, where learning is guided by the definition of the rewards and penaltiesassociated with actions taken by the AI Agent.
- The rewards and penalties against which the “AI Agent” seeks to take the “right” or optional actions are framed by the definition of valueas represented in the AI Utility Function.
- In order to create an “AI Agent” that makes the “right” decision, the AI Utility Functionmust be comprised of a holistic definition of “value” including financial/economic, operational, customer, society, environmental and spiritual value.
Figure 1: Role of AI Agents and Continuously-learning and Adapting
Bottom-line: the AI Agent determines or learns “right versus wrong” based upon the definition of value as articulated in the AI Utility Function. The AI Utility Function provides the metrics against which the AI model will learn the right actions to take in what situations (see Figure 2)
Figure 2: AI Utility Function
To avoid the unintended consequences of a poorly constructed AI Utility Function, collaboration with a diverse set of stakeholders is required to identify those short-term and long-term metrics and KPI’s against which AI model progress and success will be measured. The careful weighing of the short-term and long-term metrics associated with the financial/economic, operational, customer, society, environmental and spiritual dimensions must be taken into consideration if we are to make AI work to the benefit of all stakeholders (and maybe avoid those pesky Terminators in the process).
To help brainstorm these diverse set of metrics, embrace the “Thinking Like a Data Scientist” methodology which is designed to drive the cross-organizational collaboration necessary to root out and brainstorm these different metrics. The “Thinking Like a Data Scientist” process guides the identification of a “diverse, sometimes conflicting metrics” into the data science modeling work because the real world is full of “diverse, sometimes conflicting metrics” against which the world must try to optimize (see Figure 3).
Figure 3: The Art of Thinking Like a Data Scientist
A key deliverable from the “Thinking Like a Data Scientist” process is the Hypothesis Development Canvas. The Hypothesis Development Canvas helps in the identification of the variables and metrics against which one is going to measure the targeted use case’s progress and success. For example, increase financial value, while reducing operational costs and risks, while improving customer satisfaction and likelihood to recommend, while improving societal value and quality of life, while reducing environmental impact and carbon footprint (see Figure 4).
Figure 4: Hypothesis Development Canvas
The AI modeling requirements captured in the Hypothesis Development Canvas then need to be translated into the AI Utility Function that guides the metrics and variables against which the AI model will seek to optimize. Shortcutting the process to define the measures against which to monitor any complicated business initiative is naïve…and could ultimately be dangerous depending upon the costs associated with False Positives and False Negatives.
#2. Codifying the Costs Associated with False Positives and False Negatives
Unintended consequences can easily occur with the AI model if a thorough, comprehensive exploration of “what could go wrong” isn’t conducted prior to building the AI models, and then integrated those costs into the AI Utility Function. And that brings us into the realm of Type I and Type II errors, or False Positives and False Negatives.
- A Type I Error, or False Positive, occurs when asserting something as true when it is actually false. This false positive error is basically a “false alarm” – a result that indicates a given condition has been fulfilled when it actually has not been fulfilled (i.e., erroneously a positive result has been assumed).
- A Type II Error, or False Negative, occurs when a test result indicates that a condition has failed, when in reality the condition was successful. A Type II Error or False Negative occurs when we fail to believe something (like someone is sick, or a part is going to break) is a true condition.
I think most folks struggle to understand Type I (False Positive) and Type II (False Negative) errors, which is why I think Figure 5 summarizes Type I and Type II errors very nicely (he-he-he).
Figure 5: Understanding Type I (False Positive) and Type II (False Negative) Errors
In Figure 5, a Type I Error (False Positive) occurs when the doctor tells the man that he is pregnant, when obviously he can’t be. The Type II Error (False Negative) occurs when the doctor tells the women that she is NOT pregnant when visual inspection confirms that she is pregnant.
Let’s look at understanding the costs of False Positives and False Negative using a real-world COVID19 example. With respect to COVID19, when one has incomplete data and is trying to buy time in order to get more complete, accurate and trusted data through testing, then the best thing that one can do is to make decisions based upon the costs of the False Positives and False Negatives. In the case of the COVID19, that means:
- The cost of a False Positiveis that a healthy person will be quarantined and will be one of the first to receive the vaccine when it is available. The cost of being wrong in this case are the costs associated with being quarantined such as lost wages and the inconvenience associated with being quarantined. The cost of the False Positive in this case is very low.
- One the other hand, the cost of a False Negativeis that an infected person is classified as healthy and they continue to mingle in public infecting others and even potentially leading to the death of others. The cost of the False Negative in this case is very high.
See the blog “Using Confusion Matrices to Quantify the Cost of Being Wrong” for more homework on understanding the costs associated with False Positives and False Negatives. Maybe some of you can share this blog with some of our elected officials…
Summary
Any time you see a very complex, multi-faceted decision that has been boiled down to a single number…WATCH OUT! Creating a single number against which to monitor any complicated business initiative is naïve. Baseball, for example, leverages a bevy of numbers and metrics to determine the value of a particular player, and many of those numbers and metrics – such as Wins above Replacement, Offensive Wins above Replacement, Offensive Runs above Average and W-L Percentage of Offensive Wins above Average – are complex, composite metrics that are comprised of additional data and metrics.
In a world more and more driven by AI models, Data Scientists cannot effectively ascertain on their own the costs associated with the unintended consequences of False Positives and False Negatives. Mitigating unintended consequences requires the collaboration across a diverse set of stakeholders in order to identify the metrics against which the AI Utility Function will seek to optimize. And these metrics need to represent multiple, sometimes conflicting objectives including financial/economic, operational, customer, society, environmental and spiritual objectives. And again, the determination of the metrics that comprise the AI Utility Function is not a Data Scientist job, unless, of course, you don’t mind herds of Terminators roaming the local mall (I hear that they like sunglasses).
Podcast: Antoine Patton on Learning to Code While in Prison and Sharing That Knowledge
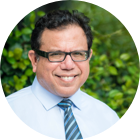
MMS • Antoine Patton
Article originally posted on InfoQ. Visit InfoQ
In this podcast recorded at QCon San Francisco 2019, Shane Hastie, Lead Editor for Culture & Methods, spoke to Antoine Patton on Holistic Ed-tech and Diversity, learning to code while in prison and founding a non-profit to teach people of color how to code.
By Antoine Patton
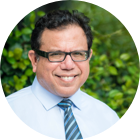
MMS • Hrishikesh Barua
Article originally posted on InfoQ. Visit InfoQ
The State of DevOps Report 2020 released by Puppet reveals that internal platforms for self-service and effective change management practices were key for organizations to move up the DevOps evolution ladder. Security integration in the software delivery pipeline also plays a key role.
By Hrishikesh Barua
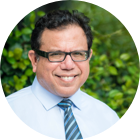
MMS • Steef-Jan Wiggers
Article originally posted on InfoQ. Visit InfoQ
Recently, Google announced a new serverless Database Migrates Service (DMS) in preview. The service supports migrations of self-hosted MySQL databases, either on-premises or in the cloud, and managed databases from other clouds, to Cloud SQL for MySQL.
By Steef-Jan Wiggers