Month: September 2021
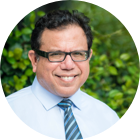
MMS • RSS
Posted on nosqlgooglealerts. Visit nosqlgooglealerts
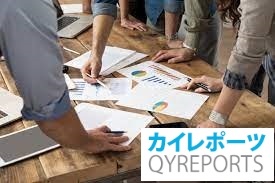
Global NoSQL Databases Market Report is a believable source for gaining the market research that will exponentially accelerate your business. SWOT and Porter’s five analysis are also effectively discussed to analyse informative data such as cost, prices, revenue, and end-users. The research report has been evaluated on the basis of various attributes such as manufacturing base, products or services and raw material to understand the requirements of the businesses. The report uses effective graphical presentation techniques, such as graphs, charts, tables as well as pictures for better understanding.
Key Players Profiled in the NoSQL Databases Market Report are as follows: Accumulo, Aerospike, Amazon SimpleDB, Azure Table, Cassandra, BigTable, Couchbase Server, CouchDB, Dynamo DB, Elasticsearch, Flink, HBase, HPCC Systems, Hypertable, MongoDB, NeDB, Oracle NoSQL, Riak, Redis,Key Product Type, Key-Value Stores, Wide-Column Stores, Document Databases, Graph Databases
Download PDF Sample Copy of this Report: https://www.qyreports.com/request-sample/?report-id=324488
The Global NoSQL Databases Market report inspects the financial standing of the leading companies, which includes gross profit, revenue generation, volume, sales revenue, individual growth rate and other financial ratios. It explains different verticals which are examined for a better understanding of the market clearly. The report is supported by significant economic facts. To present the data accurately, the study also makes use of effective graphical presentation techniques such as tables, charts, graphs, and pictures. The report further highlights recent trends, tools and technology platforms that contribute to enhance the performance of the companies.
This report is a believable source for gaining the market research that will exponentially accelerate your business. Additionally, it presents new task SWOT examination, speculation attainability investigation, and venture return investigation. The research report thoroughly explains each and every aspect related to the Global NoSQL Databases Market, which facilitates the report’s reader to study and evaluate the upcoming market trend and execute the analytical data to promote the business. The study has been conducted for 5 geographic regions: North America, Latin America, Europe, Middle East and Africa (MEA), and Asia-Pacific.
NoSQL Databases Market Segmentation:
The NoSQL Databases market report has classified the market into segments including product type, and application. Every segment is evaluated based on share and growth rate. Besides, the analysts have studied the potential regions that may prove rewarding for the manufacturers in the coming years. The regional analysis includes reliable predictions on value and volume, thereby helping market players to gain deep insights into the overall industry.
Ask for Discount on this Premium Report: https://www.qyreports.com/ask-for-discount/?report-id=324488
Reasons to buy this Report:
- We share detailed and exact information about the market forecast.
- Our reports have been examined by professional experts of the industry, which makes them beneficial for the company to maximize their return on investment.
- The analysis acknowledges that the sector players & key drivers of both conflicts and growth assess the impact of limitations as well as the opportunities on the sector.
- Data regarding the industry share by every item fragment, alongside their reasonable worth, have been served in the report.
- We provide statistic information, strategic and analysis tool results to provide a sophisticated landscape and target key market players. This will help the company to increase its efficiency.
- Our report helps readers decipher the current and future constraints of the market and optimal business strategies to enhance market development.
Highlights of NoSQL Databases Market Report:
- All-inclusive evaluation of the parent market.
- Evolution of significant market aspects.
- Industry-wide investigation of market segments.
- Assessment of market value and volume in past, present, and forecast years.
- Evaluation of market share.
- Tactical approaches of market leaders.
- Lucrative strategies to help companies strengthen their position in the market.
Global NoSQL Databases Market Report Summary:
The report studies the contemporary market to forecast the growth prospects, challenges, opportunities, risks, threats, and the trends observed in the market that can either drive or restrain the growth rate of the industry. The market factors impacting the global sector also include provincial trade policies, international trade disputes, entry barriers, and other regulatory restrictions.
Enquiry before Buying this premium Report: https://www.qyreports.com/enquiry-before-buying/?report-id=324488
Customization of This Report:
We appreciate your reading the article in its entirety. If you would like to know more about the NoSQL Databases market, looking for customization, contact us. To achieve a full market reach of NoSQL Databases, or explore more about opportunities reach our research analyst. Our team is available 24/7 to assist and support our customers through reliable research.
Seeking to initiate a fruitful business relationship with you!
Contact Us:
Name: Jones John
Contact number: +1-510-560-6005
204, Professional Center,
7950 NW 53rd Street, Miami, Florida 33166
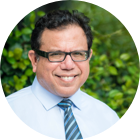
MMS • RSS
Posted on mongodb google news. Visit mongodb google news
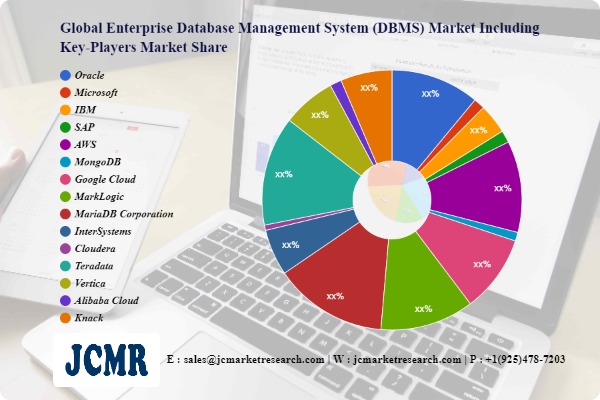
JCMR evaluating the Enterprise Database Management System (DBMS) market, highlighting opportunities, risk side analysis, and leveraged with strategic and tactical decision-making support. The Enterprise Database Management System (DBMS) study provides information on market trends and development, drivers, capacities, technologies, and on the changing dynamics of Global Enterprise Database Management System (DBMS) Market. Top companies are: Oracle, Microsoft, IBM, SAP, AWS, MongoDB, Google Cloud, MarkLogic, MariaDB Corporation, InterSystems, Cloudera, Teradata, Vertica, Alibaba Cloud, Knack, TeamDesk by ForeSoft
In the global version of Enterprise Database Management System (DBMS) report following regions and country would be covered
• Enterprise Database Management System (DBMS) North America (United States, Canada and Mexico)
• Enterprise Database Management System (DBMS) Europe (Germany, UK, France, Italy, Russia and Turkey etc.)
• Enterprise Database Management System (DBMS) Asia-Pacific (China, Japan, Korea, India, Australia and Southeast Asia (Indonesia, Thailand, Philippines, Malaysia and Vietnam))
• Enterprise Database Management System (DBMS) South America (Brazil etc.) & Middle East and Africa (North Africa and GCC Countries)
Get the inside scoop of the Sample Enterprise Database Management System (DBMS) report @: jcmarketresearch.com/report-details/1448902/sample
Research Methodology while conducting the study of “Global Enterprise Database Management System (DBMS) Market Data Survey Report 2029”
Secondary research
Our Enterprise Database Management System (DBMS) industry analyst refer a broad array of industry sources for our secondary, which typically include; however, not limited to: Company SEC filings, annual reports, company websites, broker & financial reports and investor presentations for competitive scenario and shape of the Enterprise Database Management System (DBMS) industry
• Patent and regulatory databases for understanding of technical & legal developments into Enterprise Database Management System (DBMS) industry
• Scientific and technical writings for product information and related pre-emption’s for Enterprise Database Management System (DBMS) industry
• Enterprise Database Management System (DBMS) Regional government and statistical databases for macro analysis
• Authentic new articles, web-casts and other related releases for Enterprise Database Management System (DBMS) market evaluation
• Internal and external proprietary databases, key market indicators and relevant press releases for Enterprise Database Management System (DBMS) market estimates and forecast
Complete report on Enterprise Database Management System (DBMS) Market report spread across 250+ pages, list of tables & figures, profiling 10+ companies. Read Detailed Index of full Research Study at @ jcmarketresearch.com/checkout/1448902
Primary research
Our primary research efforts include reaching out participants through mail, tele-conversations, referrals, professional networks and face-to-face interactions. We are also in professional corporate relations with various companies discussions, fulfilling following functions:
That allow us greater flexibility for reaching out Enterprise Database Management System (DBMS) industry participants and commentators for interviews and
• Validates and improves the data quality and strengthens Enterprise Database Management System (DBMS) research proceeds
• Further develops analyst team’s market understanding and expertise for Enterprise Database Management System (DBMS) industry
• Supplies authentic information about Enterprise Database Management System (DBMS) market size, share, growth and forecasts
Our primary research interview and discussion panels are typically composed of most experienced industry members. These participants include; however, not limited to:
• Chief executives and VPs of leading corporations specific to Enterprise Database Management System (DBMS) industry
• Enterprise Database Management System (DBMS) industry related Product and sales managers or country heads; channel partners and top level distributors; banking, investments and valuation experts Key opinion leaders (KOLs)
Thanks for reading this article; you can also get individual chapter wise section or region wise report version like Enterprise Database Management System (DBMS) North America, Europe or Asia.
Check for discount @ jcmarketresearch.com/report-details/1448902/discount
Key questions answered in this report – Global Enterprise Database Management System (DBMS) Market Data Survey Report 2029
What will be the market size and the growth rate in 2021?
What are the key factors driving the Global Enterprise Database Management System (DBMS) market?
Who are the key market players and what are their strategies in the Global Enterprise Database Management System (DBMS) market?
What are the key market trends impacting the growth of the Global Enterprise Database Management System (DBMS)market?
What trends, challenges and barriers are influencing its growth into Enterprise Database Management System (DBMS) industry?
What are the market opportunities and threats faced by the vendors in the Global Enterprise Database Management System (DBMS) market?
What are the key outcomes of the five forces analysis of the Enterprise Database Management System (DBMS) market?
Major Highlights of TOC:
Chapter One: Global Enterprise Database Management System (DBMS) Market Industry Overview
1.1 Enterprise Database Management System (DBMS) Industry
1.1.1 Overview
1.1.2 Products of Major Companies
1.2 Enterprise Database Management System (DBMS) Market Segment
1.2.1 Industry Chain
1.2.2 Consumer Distribution
1.3 Price & Cost Overview
Chapter Two & Three: Global Enterprise Database Management System (DBMS) Market Demand & Types
2.1 Enterprise Database Management System (DBMS) Segment Overview
Enterprise Database Management System (DBMS) Market, By Type, 2016-2021, 2022-2027 ($ Millions){linebreak}Enterprise Database Management System (DBMS) Market Segment Percentages, By Type, 2020 (%){linebreak}- Relational Database{linebreak}- Nonrelational Database{linebreak}{linebreak}Enterprise Database Management System (DBMS) Market, By Application, 2016-2021, 2022-2027 ($ Millions){linebreak}Enterprise Database Management System (DBMS) Market Segment Percentages, By Application, 2020 (%){linebreak}- SMEs{linebreak}- Large Enterprise
3.1 Global Enterprise Database Management System (DBMS) Market Size by Demand
3.2 Global Enterprise Database Management System (DBMS) Market Forecast by Demand
3.3 Enterprise Database Management System (DBMS) Market Size by Type
3.4 Enterprise Database Management System (DBMS) Market Forecast by Type
Chapter Four: Major Region of Enterprise Database Management System (DBMS) Market
4.1 Global Enterprise Database Management System (DBMS) Sales
4.2 Global Enterprise Database Management System (DBMS) Revenue & market share
Chapter Five: Enterprise Database Management System (DBMS) Major Companies List:- Oracle, Microsoft, IBM, SAP, AWS, MongoDB, Google Cloud, MarkLogic, MariaDB Corporation, InterSystems, Cloudera, Teradata, Vertica, Alibaba Cloud, Knack, TeamDesk by ForeSoft
Chapter Six: Conclusion
Contacts US:
https://jcmarketresearch.com/contact-us
JCMARKETRESEARCH
Mark Baxter (Head of Business Development)
Phone: +1 (925) 478-7203
Email: sales@jcmarketresearch.com
Connect with us at – LinkedIn
Article originally posted on mongodb google news. Visit mongodb google news
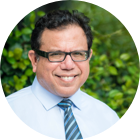
MMS • RSS
Posted on mongodb google news. Visit mongodb google news
The latest report titled Global Database Engines Market 2021 by Company, Regions, Type and Application, Forecast to 2026 is designed to follow a chapter-specific research presentation, finding out measures on demand and supply feasibilities, prevalent across the market landscape. This in-depth research report is a systematic in-depth analytical review. Details on graphical analysis and core insights have also been followed to understand the primary attractions of the market, thus serving as an investment guide for all the players comprising established and emerging players planning to establish a market stance in the global Database Engines market.
The report shows how the competition in the global Database Engines market is growing or decreasing based on a deep analysis of market concentrate rate, competitive situations, and trends, expansions, mergers, and acquisition deals. This section of the report gives statistical as well as other types of analysis of leading manufacturers in the market.
This study covers the following key players:
Google, Oracle, Microsoft, MongoDB, IBM, Redis Labs, Percona, Facebook,
DOWNLOAD FREE SAMPLE REPORT: https://www.marketsandresearch.biz/sample-request/186335
NOTE: Consumer behaviour has changed within all sectors of the society amid the COVID-19 pandemic. Industries on the other hand will have to restructure their strategies in order to adjust with the changing market requirements. This report offers you an analysis of the COVID-19 impact on the Database Engines market and will help you in strategising your business as per the new industry norms.
Then, external and internal factors that are likely to have a positive or detrimental effect on the industry have been analyzed in this report. By analyzing market segments and calculating the global Database Engines market size, analysis assists in comprehending the dynamics of the market. This report is in place to guide and influence core developments in the global Database Engines market globally. The study guides investors into an insightful representation of end-users competitive review, product portfolio, pricing, financial position, development strategies, and regional presence in the industry.
Based on product type, the market has been segmented into
Storge Engine, Query Engine,
Based on end-users/application, the market has been segmented into:
Large Enterprises, Small and Medium Sized Enterprises, Private, Others,
Advance Information On The Market:
- The report highlights novel industry trends as well as the growth steering potential of various trends
- The report also highlights factors, deterrents, as well as opportunities that direct massive growth implications in the global Database Engines market.
- Technological aids and milestones that replicate growth prognosis in the market.
- Elaborate analysis on market statistics, historical and current growth conditions have also been discussed in the report to enable futuristic growth forecasts.
- A clear analytical review of the competitive landscape, as well as highlights on core capabilities and growth objectives of the profiled players, have all been discussed in the report.
ACCESS FULL REPORT: https://www.marketsandresearch.biz/report/186335/global-database-engines-market-2021-by-company-regions-type-and-application-forecast-to-2026
The following is a complete run-down of geography-based analysis of the market:
North America (United States, Canada and Mexico), Europe (Germany, France, United Kingdom, Russia, Italy, and Rest of Europe), Asia-Pacific (China, Japan, Korea, India, Southeast Asia, and Australia), South America (Brazil, Argentina, Colombia, and Rest of South America), Middle East & Africa (Saudi Arabia, UAE, Egypt, South Africa, and Rest of Middle East & Africa)
Market size and dimensions are estimated, as well as risk management and probability and range of driver influencers in mediating growth prognosis have been included as vital research contents. The report analysts and advisors generate productive results through the use of industry tools and real customer insight. Leading market players in the global Database Engines market are covered along with production value, and growth rate.
Customization of the Report:
This report can be customized to meet the client’s requirements. Please connect with our sales team ([email protected]), who will ensure that you get a report that suits your needs. You can also get in touch with our executives on +1-201-465-4211 to share your research requirements.
Contact Us
Mark Stone
Head of Business Development
Phone: +1-201-465-4211
Email: [email protected]
Web: www.marketsandresearch.biz
View Related Report:
Global Low Performance SOC in IOT Market 2021 Research by Business Analysis, Growth Strategy and Industry Development to 2027
Article originally posted on mongodb google news. Visit mongodb google news
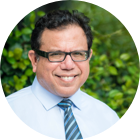
MMS • RSS
Article originally posted on Data Science Central. Visit Data Science Central
Announcements
![]() How Climate Change and Supply Chain Issues May Derail AI AdoptionThis week, the Chinese government made the announcement that several key manufacturing hubs critical to chip production will be shut down periodically in order to reduce the high demand for power (driven mostly by goal production), with companies including Apple, Nvidia, and Intel all making announcements that this would have a direct impact upon their own supply chain availability. This announcement came at a particularly bad time for the beleaguered computer chip industry. A fire at a major chip fabrication plant in Japan earlier this year has already impacted automobile production in the United States and elsewhere, as many of the chips produced there were specifically for the increasingly complex machine learning components going into contemporary vehicles. Additionally, the emergence of the Delta variant of the Covid-19 virus is ramping up as much of the Northern Hemisphere moves into the Fall and Winter months, which had both forced many companies that were just beginning to emerge from the earlier strains of the virus to once again put plans on hold, quite probably into late Spring. This is also putting strain on chip production, especially specialized GPUs that are at the core of the AI revolution. This has pushed the cost of electronics and related goods up significantly as inflation, which has been fairly dormant for the last twenty years, is now beginning to heat up. From an enterprise standpoint, this is generally bad news, though in the longer term one effect of this is likely to be that more countries will start to invest once again into their own chip production facilities (as Japan, South Korea, and the United States have all recently announced they are ramping up to do). However, in the interim this deficit is likely to hit companies that have been buying these specialized high-performance chips for building out machine learning and AI-based pipelines, as well as those involved in media production. It’s also possible, though by no means certain, that this could have the effect of reducing the hiring of data scientists and machine learning specialists, at least in the short term, until new capability comes online. Whether this spills out into the broader economy remains to be seen, but the increasing stress on global supply chains, coupled with the increased environmental and energy costs of advanced computing, makes this worth keeping an eye on. In media res, Kurt Cagle To subscribe to the DSC Newsletter, go to Data Science Central and become a member today. It’s free! Data Science Central Editorial CalendarDSC is looking for editorial content specifically in these areas for September, with these topics having higher priority than other incoming articles.
DSC Featured ArticlesPicture of the Week
To make sure you keep getting these emails, please add mail@newsletter.datasciencecentral.com to your browser’s address book.
Join Data Science Central | Comprehensive Repository of Data Science and ML Resources
Follow us on Twitter: @DataScienceCtrl | @AnalyticBridge This email, and all related content, is published by Data Science Central, a division of TechTarget, Inc.
275 Grove Street, Newton, Massachusetts, 02466 US
|
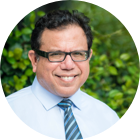
MMS • RSS
Posted on mongodb google news. Visit mongodb google news
(VIANEWS) – Shares of MongoDB (NASDAQ Composite: MDB) slid 5.13% to $463.57 at 20:04 EST on Tuesday, after three consecutive sessions in a row of gains. NASDAQ Composite is dropping 1.35% to $14,546.68, after five consecutive sessions in a row of losses. This seems, at the moment, an all-around negative trend exchanging session today.
MongoDB’s last close was $507.41, 1.47% below its 52-week high of $515.00.
Volume
Today’s last reported volume for MongoDB is 794901 which is 0.73% below its average volume of 800793.
The company’s growth estimates for the present quarter and the next is a negative 19.4% and a negative 3%, respectively.
MongoDB’s Revenue
Year-on-year quarterly revenue growth grew by 39.4%, now sitting on 641.7M for the twelve trailing months.
MongoDB’s Stock Yearly Top and Bottom Value
MongoDB’s stock is valued at $463.57 at 20:04 EST, under its 52-week high of $515.00 and way higher than its 52-week low of $211.29.
MongoDB’s Moving Average
MongoDB’s worth is way above its 50-day moving average of $417.21 and way above its 200-day moving average of $341.87.
More news about MongoDB (MDB).
Article originally posted on mongodb google news. Visit mongodb google news
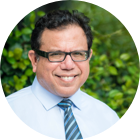
MMS • RSS
Posted on mongodb google news. Visit mongodb google news
What happened
Many tech stocks got a haircut on Tuesday as Wall Street turned away from seemingly risky investments in favor of more sensible value plays. Any ticker with a recent history of market-beating returns was fair game for a sharp correction, including many popular investments in the technology sector.
As of 2:35 p.m, EDT, database specialist MongoDB (NASDAQ:MDB) had fallen as much as 4.8%. E-commerce technologist Shopify (NYSE:SHOP) dipped 5.9% lower at most. Data-warehousing expert Snowflake (NYSE:SNOW) bounced back from a 5.5% nadir, and media-streaming veteran Roku (NASDAQ:ROKU) found support after a 4.5% fall. Even mighty Apple (NASDAQ:AAPL) suffered a maximum drop of 2.5%.
Together, the lowest points for this group of five stocks added up to $80.6 billion of lost market value today. The funny thing is, none of these fantastic companies did anything wrong. They were punished as a group for the debatable offense of delivering strong returns to shareholders in recent months.
Image source: Getty Images.
So what
The specific details may differ, but the themes are all the same. Here’s what these stocks looked like on Monday evening:
- MongoDB had gained 133% over the previous 52 weeks, trading at 42 times trailing sales with negative earnings.
- Roku looked back at a one-year gain of 88%. The stock traded at 196 times trailing earnings and 19 times sales.
- Shopify had amassed a 54% return over the same period. Shares were changing hands at 392 times earnings and 47 times sales.
- Snowflake’s annual gain stopped at 48%. Like MongoDB, this company is not reporting positive bottom-line earnings. The stock was soaring at 95 times trailing sales.
- Apple’s full-year returns were actually slightly below the S&P 500‘s 35% gain, but the iPhone maker had tripled the broader market’s 5% returns since early June. At 28.5 times earnings and 7 times sales, Apple’s shares straddled the line between growth stocks and value investments.
These combinations of recent gains and soaring valuation ratios set our example tickers up for painful corrections today. To be clear, none of the companies listed above had any significant news of their own today.
Now what
The driving force behind Tuesday’s flight from riskier stocks was a surging bond market, where the yield on 10-year Treasury notes rose to a three-month high of 1.53%. The stock market can be sensitive to large moves in these ultra-safe investment returns. Higher bond yields give value investors a more sensible safe haven in times of turmoil.
In turn, the rising bond rates followed from the idea that the Federal Reserve looks ready to stop plowing billions of dollars into the bond market someday soon. The rising yields are still lower than the long-term annual inflation target of 2%, which limits the power of the bond market as a whole. Tuesday’s anti-risk corrections will look tame by comparison if that yield-to-inflation relationship flips around, which might actually happen when the Fed’s quantitative easing program finally runs its course.
I’m not saying it’s time to sell every high-risk stock and reinvest the cash in bonds and gold, but investors should be ready for a more dramatic drop in the relatively near future. If anything, I might increase my investments in MongoDB and Roku if their stock prices tumble for purely economy-based reasons.
After all, these great companies are poised for long-term success in their chosen sectors regardless of bond yields and inflation rates. In the long run, stock prices are based on business results, not on the availability or lack of investment alternatives.
This article represents the opinion of the writer, who may disagree with the “official” recommendation position of a Motley Fool premium advisory service. We’re motley! Questioning an investing thesis — even one of our own — helps us all think critically about investing and make decisions that help us become smarter, happier, and richer.
Article originally posted on mongodb google news. Visit mongodb google news
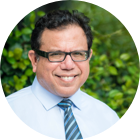
MMS • Sergio De Simone
Article originally posted on InfoQ. Visit InfoQ
The latest release of Apple’s language, Swift 5.5, introduces new features aimed at making it easier for developers to write asynchronous code as well as several extensions to the language and compiler, not the least CGFloat and Double interchangeability.
By Sergio De Simone
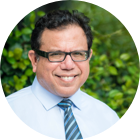
MMS • RSS
Article originally posted on Data Science Central. Visit Data Science Central
Summary
Regression analysis is one of the methods supplied “built-in” with SAP BW Data Mining. Based on this method regression models can be created and configured to satisfy specific analysis requirements (e.g., choice between linear or non-linear approximation, etc.). The method includes regression-specific reporting that allows analysis of the modeling results. In this paper we are suggesting a number of ways to extend this reporting in order to improve insight into the results of regression modeling. The mentioned extensions are implemented via the following analytics:
- Dashboard – SAP BW Data Mining Regression Reporting
Business Requirements
We will set our focus on the method-specific (not problem-specific) indicators that appear on regression reporting in BW Data Mining. That means that we will not be considering the part of the standard BW Data Mining reporting that visualizes regression scores (predicted values) neither regression coefficients for particular regression models. Instead, we will concentrate on the indicators that provide insight as to the volume and quality of models’ input data, as well as the quality of approximation achieved via the models.
The abovementioned method-specific indicators can be viewed via either the basic statistics of models involved in analysis processes (transaction RSANWB, display the analysis process, right-click on the model and select to display basic statistics) or via the general statistics in a model’s results (transaction RSDMWB, display the model, choose the modeling results button in the model’s toolbar, choose the general statistics button in the toolbar of the main results graph).
An example of visualization available via the basic statistics of an analysis process is provided in the below screenshot:
An example of visualization available via general statistics in a regression model’s results is provided in the below screenshot:
The above visualization functionalities cover well the basic needs of a user that would like to obtain insight in the results of regression modeling. Based on our practical experience with regression modeling in SAP BW Data Mining the following additional business requirements could be suggested:
- Ability to browse specific regression models to visualize their most important details without clicking into those models and studying them via a Display/Edit mode
- Ability to visualize the method-specific indicators of regression models without going into either basic statistics or general statistics of individual processes and models
- Ability to visualize the method-specific indicators per model variable with sufficient precision (i.e. not rounded to an integer or to only three digits after the decimal point)
- Ability to select and visualize in a graphical mode only the comparable method-specific indicators (in order to avoid difficulties with their visualization among the other indicators, usually with greater values)
- Ability to select and visualize only the details (indicators and data properties) of specific model variables
Analytics
The implementation of the above business requirements in the “SAP BW Data Mining Regression Reporting” dashboard is based on combining the functionality of the “SAP BW Data Mining Model Reporting” dashboard (find more details on this dashboard in SAP BW Data Mining Analytics: Model Reporting) with insight that is specific for SAP_REGRESSION and SAP_SCORING_WT_TABLE methods.
At startup, the “SAP BW Data Mining Regression Reporting” dashboard displays three tabs:
- Model Master: contains the overall list of SAP BW Data Mining models defined in our system with their most important data properties, plus a set of controls allowing to browse and filter that list
- Regression/Scoring Models – Table: contains the list of models based on SAP_REGRESSION and SAP_SCORING_WT_TABLE methods with their variables and method-specific indicators, plus a set of controls allowing to browse and filter that list
- Regression/Scoring Models – Graphs: contains the bar chart to visualize the method-specific indicators for the models and variables chosen using the two other tabs, plus a set of controls to select for visualization specific indicators
- The following columns have been enabled in the list at the Model Master tab (see the screenshot below):
- Model ID – a unique identifier, the “technical name” of the model in the overall model list
- Model Field Name – an identifier of a variable (field) of the model in the overall model list
- Modeling Method – the modeling method on which the model is based
- Version – the version of the model
- Field Data Type – the type of the data contained in a model field
- Field Content Type – the role that a model field plays in the model
- Field Is Predictable – contains “X” if the field is a predictable field, i.e. the one that if filled with prediction results
- Character Field Length – the total length of a character field
- Numeric Field Length – the total length of a numeric field
- Numeric Field Precision – the number of digits after the decimal point in a numeric field
The following columns have been enabled in the list at the Regression/Scoring Models – Table tab (see the screenshot below):
- Model ID – a unique identifier, the “technical name” of the model in the regression-specific model list
- Row Number – an identifier of a variable (field) of the model in the regression-specific model list
- Goodness Indicator I – an indicator of goodness of approximation
- Goodness Indicator R – an indicator of goodness of approximation
- Total of Absolute Differences – the sum of absolute predicted/observed differences
- Total of Predicted Values – the sum of predicted values
- Total of Observed Values – the sum of observed values
- Number of Data Records – the number of data points loaded into a regression model during its training
The selectors of the dashboard match the columns of the lists and allow limiting the models and variables visualized via the lists to specific criteria. Each time a specific value is selected, the respective selector’s status indicator turns green.
In the below screenshot, the PIO_INV_RG_L value is selected via the Model ID selector. The lists of variables in the Model Master and Regression/Scoring Models – Table tabs are immediately refreshed to display only the records corresponding to the model with the technical name PIO_INV_RG_L:
In order to visualize only the details of a specific variable, we must select this variable using the Model Field Name and Row Number selectors. The Model Field Name selector applies to the Model Master tab:
The Row Number selector applies to the Regression/Scoring Models – Table tab:
The necessity to indicate two different identifiers for the same variable is due to the fact that the variables are coded differently in the SAP BW Data Mining’s internal tables – the overall model master table uses the model field names indicated in the model definitions, while the regression-specific model master table uses the numbers of the rows occupied by the respective variables in the regression model definitions. We are working on the link among the two sets of identifiers and will implement it in the further versions of this dashboard. For the time being, a reasonable workaround is based on the fact that the order in which the model field names and of the row numbers for a selected model appear on the dropdown lists is the same – which allows us to simply count the same number of labels from the top of the dropdown lists in the Model Field Name and Row Number selectors in order to make sure that the same variable is selected in both of the respective tabs.
Finally, we may need to obtain graphical visualization of the values of the method-specific indicators displayed in the Regression/Scoring Models – Table tab. Such visualization is implemented in the Regression/Scoring Models – Graphs tab. In the below screenshot we can see that all of the six method-specific indicators for the variable PIOINV08 (corresponds to the row number 3, which is displayed next to the graph’s Y-axis) are displayed, but because the values for the two goodness-of-fit indicators, as well as for the total of absolute differences indicator, are so small compared to the values of the other indicators that we can only see three bars in the bar chart (with the bars corresponding to the indicators with smaller values “molded” into the Y-axis):
A similar inconvenience, as it was mentioned before, could be found with the standard SAP BW Data Mining reporting related to regression modeling results. Our dashboard proposes and efficient workaround – we can uncheck directly in the graph’s legend the indicators with greater values and to have the indicators with smaller values visualized along the automatically rescaled X-axis:
Typical Use Cases
The following could be examples of the typical use cases in which the usage of the SAP BW Data Mining Regression Reporting dashboard could bring benefits:
1) A data mining specialist would like to visualize the models with Y as predictable variable and to study method-specific indicators of those of them that are based on the SAP_REGRESSION method.
Use scenario: in the Model Master tab, select the records that correspond to the SAP_REGRESSION method using the Modeling Method selector, then limit further your selection by choosing Y via the Model Field Name selector and X in the Field Is Predictable selector. The dropdown list of the Model ID selector will contain the technical names of the models we are interested in. Choose those models one by one in the Model ID selector and study their method-specific indicators in the Regression/Scoring Models – Table and Regression/Scoring Models – Graphs tabs.
2) A data mining specialist would like to visualize the models based on the SAP_REGRESSION method that contain at least 10 variables with the predictable variable having exactly N digits after the decimal point.
Use scenario: in the Model Master tab, select the records that correspond to the SAP_REGRESSION method using the Modeling Method selector, and then limit further your selection by choosing X in the Field Is Predictable selector and the value closest to or exactly matching N in the Num. Field Precision selector. The model list in the Model Master tab will display the technical names of the models matching all of the above criteria except for having at least 10 variables. To apply this last criterion, switch to the Regression/Scoring Models – Table tab and choose 10 in the Row Number selector (if 10 is not available, there are no models that match this criterion). Choose one by one the model technical names displayed in the Model ID selector in the Regression/Scoring Models – Table tab to verify whether the models selected with the help of selectors in the Model Master tab are compatible with having at least 10 variables criterion. If on selection of a model technical name via the Model ID selector the model list in the Regression/Scoring Models – Table tab displays at least one row, then the respective model satisfies all the criteria. If the list is empty, the respective model has less than 10 rows.
3) A data mining specialist would like to visualize models based on the SAP_REGRESSION method that contain variable Y as predictable variable and to find out which of those models provides the highest value of the goodness indicator R.
Use scenario: in the Model Master tab, select the records that correspond to the SAP_REGRESSION method using the Modeling Method selector, then limit further your selection by choosing Y in the Model Field Name and X in the Field Is Predictable selectors. This will leave in the dropdown list of the Model ID selector the models that satisfy the above criteria. Switch to the Regression/Scoring Models – Table tab and choose one by one the model technical names displayed in the Model ID selector to visualize the values of the goodness indicator R. Those values may differ across a model’s variables, and then to identify the highest value per model we could either apply sorting in the Goodness Indicator R column (by clicking on that column’s caption), or to switch to the Regression/Scoring Models – Graphs tab and to remove selection from all the options in the graph’s legend except for Goodness Indicator R. By choosing the model technical names available in the Model ID selector and observing the highest value of the goodness indicator R per model, we can identify the model which provides the highest value for this indicator.
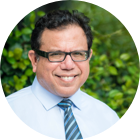
MMS • RSS
Article originally posted on Data Science Central. Visit Data Science Central
I am very fortunate that I get asked to present at several universities about what students (and all professionals) can do to “future proof” their careers. We live in a world of constant change driven by technology, economics, pandemics, environmental, political, and society forces. We live in a world where we need to build “transformational muscle” so that we not only can survive – but can actually thrive – in a world of constant disruption and transformation.
And I believe that a “future proof” foundation is built by blending the critical and empowering disciplines of data science, design thinking, and economics. When you meld those three together, you are certainly in a great position for whatever the future is going to throw at you (Figure 1).
Figure 1: Blending Data Science, Design Thinking and Economics to Future Proofing Your Career
Discipline #1: Data Science – the Language of AI / ML
Data Science is about identifying those variables and metrics that might be better predictors of behaviors and performance.
While not everyone will be required to code a Neural Network algorithm (thank God), it is critically important that everyone learns what can be done with advanced analytic capabilities like Machine Learning, Neural Networks, Reinforcement Learning, and Artificial Intelligence. While there seems to be multiple ways to define the “Advanced Analytics topology”, I use the following 3 levels to help explain the differences to my students (Figure 2):
Level 1 quantifies cause-and-effect (strength of relationships) and goodness of fit (model accuracy) using:
- Statistics are used to support hypothesis (decision) testing and provide credibility to model outcomes (confidence levels, p-values, goodness-of-fit)
- Predictive Analytics and Data Mining uncover statistically significant patterns, trends, and relationships buried in large data sets to quantify risks and opportunities
Level 2 predicts likely actions or outcomes in order to prescribe recommendations to improve human decision-making using:
- Deep Learning (Neural Networks) recognizes “things” – images, photos, voice, audio, video, text, handwriting – out of complex data formats
- Machine Learning identifies relationships and patterns in the data. Supervised Machine Learning identifies “known unknown” relationships and patterns from “labeled” outcomes (e.g., purchase, fraud, attrition, breakage) using algorithms such as linear regression, logistic regression, Naive Bayes, and Support Vector Machine (SVM). Unsupervised Machine Learning identifies “unknown unknown” relationships and patterns from data with no labeled outcomes using algorithms such as clustering, segmentation, and K- nearest neighbor.
Level 3 seeks to continuously-learn and adapt within a continuously changing environments with minimal human intervention (robots, vacuums, autonomous vehicles) using:
- Reinforcement Learning takes actions within a controlled environment to maximize rewards while minimizing costs. Reinforcement Learning uses trial-and-error to map situations to actions to maximize rewards (think of the kids’ game Hotter/Colder).
- Artificial Intelligence acquires knowledge about a specific environment, applies knowledge to successfully interact with environment, and continuously learns from interactions so that subsequent interactions get more effective with minimal human intervention.
Figure 2: Three Levels of Analytics Maturity
Discipline #2: Design Thinking – the Language of your Customer
“Design Thinking is a human-centered and collaborative approach to problem solving using a design mindset to solve wicked complex problems” – IDEO
Design Thinking is all about people…their points of view…and their stories. Design Thinking is about gaining an intimate understanding of your customers – what jobs they are seeking to do, and the gains (benefits) and pains (impediments) that they encounter on their personal journey (Figure 3).
Figure 3: Design Thinking: Speaking the Language of Your Customer
But the key to Design Thinking is the empowering mindset that it establishes across all the stakeholders. Design Thinking seeks to empower and democratize the ideation process by ensuring that all ideas, regardless from whom they originated, are worthy of consideration. That one can only have break-through moments if they are willing to fail and learn from those failures. To seek to unleash the greatness that is in every one of us. Design Thinking creates a culture of rapid exploration, rapid testing, failure-tolerant, continuous learning and adapting (Figure 4).
Figure 4: Design Thinking Users Empowerment to Democratize Ideation
Discipline #3: Economics – the Language of Business
Economics is the branch of knowledge concerned with the production, consumption, and transfer of wealth or value.
Data and analytics, in particular, possess unique economic characteristics that enable new opportunities to drive and derive new sources of customer, product, and operational value including:
Nanoeconomics is the economic theory of individual entity (human or device) predicted behavioral and performance propensities. We can apply Nanoeconomics to transition the organization from making decisions based upon overly generalized averages, to making decisions on individual human or device entity’s predicted behavioral and performance propensities. Organizations can leverage Nanoeconomics to transform their economic value curve – which measures the relationship between a dependent outcome and independent inputs required to achieve that outcome – to deliver more value (outputs) with less investments (inputs). See Figure 5.
Figure 5: The Economic Theory of Nanoeconomics
Schmarzo Economic Digital Asset Valuation Theorem. Economics is a powerful enabler, but when it comes to digital economic assets, the power is magnitudes greater because (Figure 6):
- Data is an asset that never depletes, never wears out, and can be used across unlimited use cases at zero marginal cost.
- Using AI, organizations can build analytic assets that appreciate, not depreciate, in value the more they are used.
- Data Economic Multiplier Effect measures the increase in aggregated value from the application and reuse of the organization’s data and analytic assets against the organization’s use cases at zero marginal cost.
- Marginal Propensity to Reuse (MPR) states that an increase in the reuse of a digital asset across multiple use cases drives an increase in the attributable value of that digital asset at zero marginal cost.
Figure 6: Schmarzo Economic Digital Asset Valuation Theorem
Future Proofing Your Career Summary
In a world of constant disruption and transformation, everyone needs to embrace a mindset of lifetime learning. And the best way to future proof your career, no matter your profession, is to blend the disciplines of data science, design thinking, and economics.
I can dream, right?
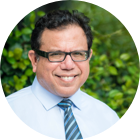
MMS • RSS
Posted on nosqlgooglealerts. Visit nosqlgooglealerts
<!–
Join us free for the Data Summit Connect 2021 virtual event!
We have a limited number of free passes for our White Paper readers.
Claim yours now when you register using code WP21.
–>
PostgreSQL is an incredibly reliable open-source database technology that continues to grow in popularity with its users whether its supporting enterprise-grade workloads and commercial databases. It’s flexible, you can use it for SQL and NoSQL workloads, and has high availability. On-premises PostgreSQL deployments make it difficult to harness the true potential of these databases.
Migrating PostgreSQL to a cloud platform like Amazon Aurora can deliver a host of benefits including increased flexibility, greater capacity, improved security and automation to start. It also requires a thorough understanding of your current on-premises databases, your application requirements, your database migration goals, and your technical resources.
Download Datavail’s white paper to discover why your PostgreSQL database should live on Amazon Aurora and learn:
- A brief overview of AWS & Amazon Aurora
- 15 benefits of an Amazon Aurora migration
- What should organizations know before migrating PostgreSQL to Amazon Aurora
- Best practices & use case scenarios for a migration