Month: January 2022
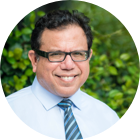
MMS • RSS
Posted on nosqlgooglealerts. Visit nosqlgooglealerts
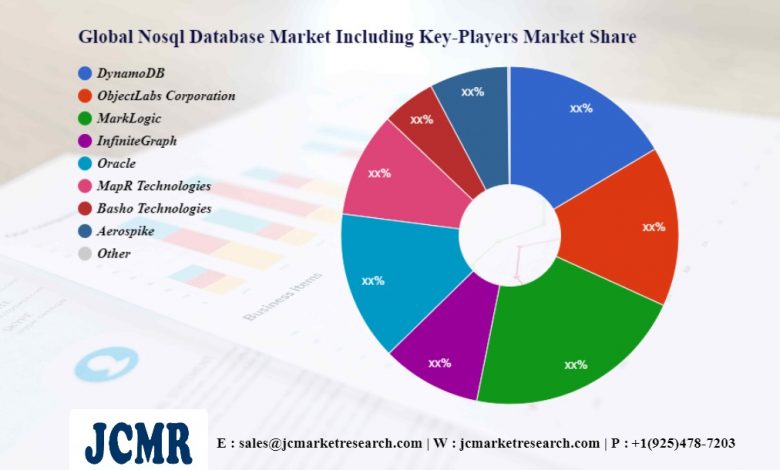
A new research study from JCMR with title Global Nosql Database Market Research Report 2021 provides an in-depth assessment of the Nosql Database including key market trends, upcoming technologies, industry drivers, challenges, regulatory policies & strategies. The research study provides forecasts for Nosql Database investments till 2029.
The report does include most recent post-pandemic market survey on Nosql Database Market.
Competition Analysis : DynamoDB, ObjectLabs Corporation, Skyll, MarkLogic, InfiniteGraph, Oracle, MapR Technologies, he Apache Software Foundation, Basho Technologies, Aerospike
Request Free PDF Sample Report @: jcmarketresearch.com/report-details/1114162/sample
Commonly Asked Questions:
- At what rate is the market projected to grow
The year-over-year growth for 2021 is estimated at XX% and the incremental growth of the Nosql Database market is anticipated to be $xxx million.
Get Up to 40 % Discount on Enterprise Copy & Customization Available for Following Regions & Country: North America, South & Central America, Middle East & Africa, Europe, Asia-Pacific
- Who are the top players in the Nosql Database market?
DynamoDB, ObjectLabs Corporation, Skyll, MarkLogic, InfiniteGraph, Oracle, MapR Technologies, he Apache Software Foundation, Basho Technologies, Aerospike
- What are the key Nosql Database market drivers and challenges?
The demand for strengthening ASW capabilities is one of the major factors driving the Nosql Database market.
- How big is the North America Nosql Database market?
The North America region will contribute XX% of the Nosql Database market share
Enquiry for Nosql Database segment [email protected] jcmarketresearch.com/report-details/1114162/enquiry
This customized Nosql Database report will also help clients keep up with new product launches in direct & indirect COVID-19 related markets, upcoming vaccines and pipeline analysis, and significant developments in vendor operations and government regulations
Nosql Database Geographical Analysis:
• Nosql Database industry North America: United States, Canada, and Mexico.
• Nosql Database industry South & Central America: Argentina, Chile, and Brazil.
• Nosql Database industry Middle East & Africa: Saudi Arabia, UAE, Turkey, Egypt and South Africa.
• Nosql Database industry Europe: UK, France, Italy, Germany, Spain, and Russia.
• Nosql Database industry Asia-Pacific: India, China, Japan, South Korea, Indonesia, Singapore, and Australia.
Market Analysis by Types & Market Analysis by Applications are as followed:
[Segments]
Some of the Points cover in Global Nosql Database Market Research Report is:
Chapter 1: Overview of Global Nosql Database Market (2013-2025)
• Nosql Database Definition
• Nosql Database Specifications
• Nosql Database Classification
• Nosql Database Applications
• Nosql Database Regions
Chapter 2: Nosql Database Market Competition by Players/Suppliers 2013 and 2018
• Nosql Database Manufacturing Cost Structure
• Nosql Database Raw Material and Suppliers
• Nosql Database Manufacturing Process
• Nosql Database Industry Chain Structure
Chapter 3: Nosql Database Sales (Volume) and Revenue (Value) by Region (2013-2021)
• Nosql Database Sales
• Nosql Database Revenue and market share
Chapter 4, 5 and 6: Global Nosql Database Market by Type, Application & Players/Suppliers Profiles (2013-2021)
• Nosql Database Market Share by Type & Application
• Nosql Database Growth Rate by Type & Application
• Nosql Database Drivers and Opportunities
• Nosql Database Company Basic Information
Continue……………
Note: Please Share Your Budget on Call/Mail We will try to Reach your Requirement @ Phone: +1 (925) 478-7203 / Email: [email protected]
Find more research reports on Nosql Database Industry. By JC Market Research.
Thanks for reading this article; you can also get individual chapter wise section or region wise report version like North America, Europe or Asia.
About Author:
JCMR global research and market intelligence consulting organization is uniquely positioned to not only identify growth opportunities but to also empower and inspire you to create visionary growth strategies for futures, enabled by our extraordinary depth and breadth of thought leadership, research, tools, events and experience that assist you for making goals into a reality. Our understanding of the interplay between industry convergence, Mega Trends, technologies and market trends provides our clients with new business models and expansion opportunities. We are focused on identifying the “Accurate Forecast” in every industry we cover so our clients can reap the benefits of being early market entrants and can accomplish their “Goals & Objectives”.
Contact Us: https://jcmarketresearch.com/contact-us
JCMARKETRESEARCH
Mark Baxter (Head of Business Development)
Phone: +1 (925) 478-7203
Email: [email protected]
Connect with us at – LinkedIn
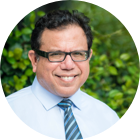
MMS • Jason Yee John Egan Ben Sigelman
Article originally posted on InfoQ. Visit InfoQ
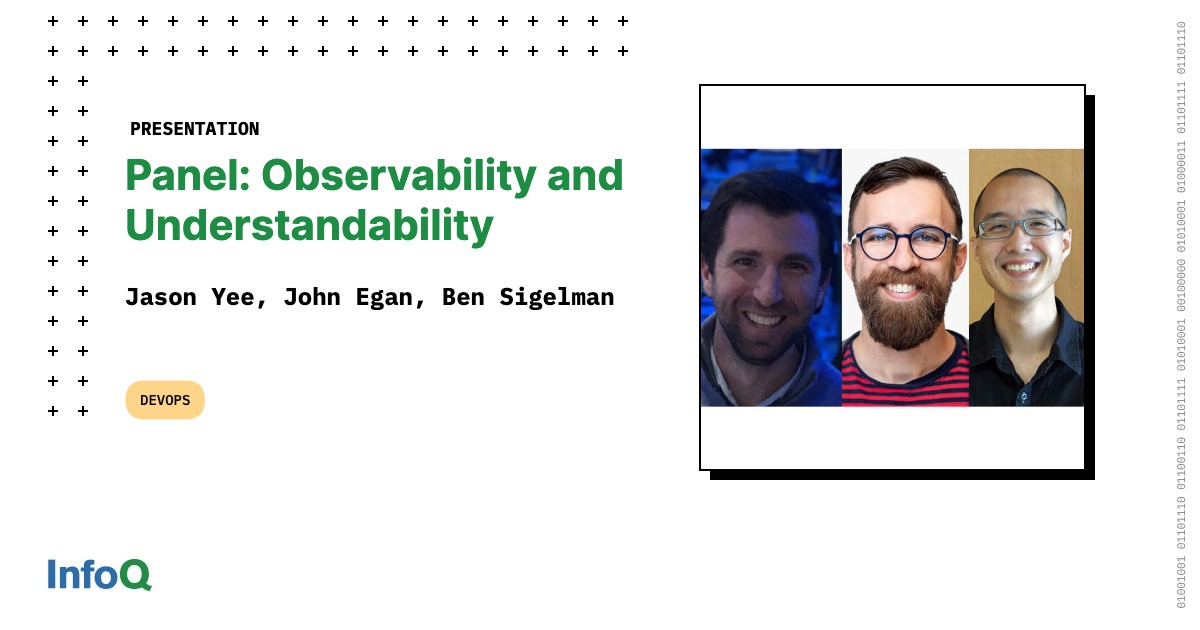
Transcript
Butow: This panel brings together innovative leaders from the world of incident management, OpenTelemetry, large scale distributed systems, to discuss and share their current thoughts on the state of observability and understandability.
You’ll just be hearing from our technical experts who’ve actually been in the trenches. They’ve achieved incredible results, and they’ve learned a ton along the way. The speakers on this panel didn’t just do the work, they also created entire movements to bring thousands of people along with them to achieve and celebrate success in these areas, too.
Introduction
Yee: I’m Jason Yee. I work at Gremlin as the Director of Advocacy, which means I get the amazing pleasure of working with Tammy on a day to day basis. I’ve given talks on everything from monitoring and observability, to chaos engineering, to innovation, which is my passion, is how do we help engineers be better and create the next big thing, or just improve their lives?
Sigelman: My name is Ben Sigelman. I’m one of the co-founders and the CEO at Lightstep.
Egan: I’m John Egan. I’m CEO and co-founder at Kintaba. We’re an incident management platform that try and help companies be able to implement best practices for incident management across the entire organization, as opposed to just within their SRE teams. I gave a talk about why it’s important for companies to increase the overall number of incidents that they’re tracking internally as a top level metric, as compared to maybe some of the more standard MTT* metrics.
How Different Companies Manage Incidents
Butow: Previously, when I worked at Dropbox, I worked with a lot of folks who were from Google, and YouTube. Then also a lot of folks who were from Facebook. It was really interesting to hear their different viewpoints of how they did things. Ben, like you came from Google. John, you came from Facebook. I thought it would be interesting, have you noticed that yourselves, that there’s a different view from Google to Facebook of how to, for example, manage incidents? You both talked about SLOs and SLIs. What are your thoughts there?
Egan: Having not worked at Google, I won’t try to speak directly to how things operate there. I’ll say what I thought worked really well at Facebook was its ability to ingest the incident process across the entire organization. Such that if you’re an employee at Facebook, and something’s happening deep within the engineering team, deep within the infrastructure team or otherwise, you always have immediate and open visibility into everything from the top level information on that incident, all the way down to the specific details of exactly what’s being done. It was this really powerful impact of everyone in the company knew what a SEV1 was. Everyone knew how to respect that and stay off people’s backs when things are being worked on as high priority. The cultural impact of that was really strong. It meant everyone from sales team out to PR team, out to otherwise, responded really well to the occurrence of these incidents. As a product manager there, where I primarily operated, that was really impactful for me, as a non-engineer, actually. In that the way that we responded to incidents started to look similar to the way that engineers and SREs, or groups of people who’ve been doing this for much longer than us would approach things and we would match that. I thought it was a really powerful indicator across the company of shared cultural values up from engineering. One of the reasons I pushed back on things like MTTR, and some of those areas is those top level cultural impacts, I thought long term actually had a more visible long term resilience effect on the organization versus just like the deep in incident response metrics themselves.
Butow: Yes, taking the bigger picture.
Sigelman: I never worked at Facebook, so I’m not going to speak for the Facebook side of it. I would agree, Tammy, there are some cultural differences between the way that SRE is practiced in those two organizations. For Google, it’s funny, they did a lot of things right. I think it was really cool and progressive of them to say, we’re not going to call this Ops, we’re going to call this site reliability engineering, which I think they coined that term. I believe they did anyway. It seemed very new when I heard it, and think about this as a real practice. It was, I think, really amazing that they did that. It was so amazing that they created their own organization for Site Reliability Engineering, that was parallel to the product and engineering organization. I’m not sure that was such a good idea. They ended up creating the org chart. When there’s 10 people, it’s fine. When you have 1000 people in the organization, it does inevitably become somewhat political. There just wasn’t that much political incentive to be reasonable.
I do remember a meeting I had once, where I was talking to a very senior leader in the SRE organization, about Monarch, which was our distributed, multi-tenant, time-series database, like a metrics system. We were trying to figure out what the SLA should be for Monarch. I just asked him like, “What availability requirement do you need?” He’s like, “I need 100% availability.” I was like, “That’s not reasonable. Can you tell me, like how many nines? Can you just work something, something not 100?” He’s like, “No, I need 100% reliability.” It wasn’t reasonable, but he had no reason to be reasonable politically. I think having SRE as a completely segregated organization allowed him to be completely unreasonable. He was.
As a result, you can go look at the Monarch paper, it’s awfully reliable. It also consumes, steady state, 250,000 VMs are dedicated to Monarch. Just think about how expensive that is. It is extremely expensive. That was not, in my mind, actually, the right decision. We probably should have, instead of shooting for four nines, should have shot for like three nines, and then had some other thing for the super high availability or whatever. It’s just the political situation as it wasn’t his P&L, and he didn’t care. He just asked for an unreasonable amount of reliability, and here we are. I think that there is a lesson there. SRE is an amazing thing, but I think there needs to be a check and a balance on reliability as a goal. It needs to be balanced with other product velocity concerns and cost. I don’t think that Google set things up to succeed in that regard. Not to say that everyone is political, of course, but it was an issue.
Butow: Yes, it’s definitely an interesting conversation. This came up also, in your talk, Ben, about cost, just like the cost associated with observability, being able to understand systems and what’s happening. Amazon’s famous for everyone saying that they’re very frugal. They’re trying to always reduce costs and keep costs down. It’s one of their cultural items that they really do focus on. It’s just interesting, different companies do things different ways and have different goals that they strive towards. I think that’s great to be able to learn from how people do things.
How Hobbies outside Work Help with Observing and Understanding Systems
Something else that I’m really interested in, is how have hobbies outside of work helped you observe and understand systems? I always think this is really interesting, because our job, day to day, isn’t the only thing that we learn from. What else do you learn from?
Yee: That’s one of the things that I love about being an engineer. I think the question was about learning. I think that’s a big reason why there’s such a movement for this idea of bringing your whole self to work, is we learn from all these other things that we do. As an example, what we deal with. We always talk about complex systems. You can’t look for single root causes, because we’re in a complex system. Everybody has this idea of like, this implicit understanding, yes, it’s a complex system. Then, oftentimes, we treat it like it’s not. One of my pandemic hobbies that I picked up was chocolate making, like bean to bar chocolate making. I buy raw cacao beans, from an importer who goes around the world getting cacao. I roast it in my oven, and I shell it, and then make chocolate. It is simultaneously one of the most fun, enjoyable things that I’ve done and learned how to do, and also extremely frustrating because when you make chocolate, there’s a process of tempering. The idea is that chocolate that you buy in the store, it doesn’t melt in your hands, because it’s tempered. It has this nice snap point when you break it. That’s a process of crystallization. It’s this extremely complex thing of like the smallest changes in humidity in your room. You can do almost everything the exact same and have something that completely fails, whereas you did it yesterday, and because that humidity was slightly off, it came out perfect.
I think that’s something that I’ve started to realize, as we deal with the systems we work on, is we inherently understand that it’s complex, but I don’t think we realize how complex when we’re talking about like, I automate deployments. We did two dozen deployments yesterday, and the idea of what worked in the morning may not work an hour from now, because you just had 10 different deployments going on. I love bringing in that stuff, because I think it helps us make better sense when we’re thinking about the stuff we work on.
Butow: Yes, definitely. It helps explain it to folks as well that don’t work in our world, if they ask you like, how come things just don’t work perfectly every single day? They worked great yesterday. You can really take those different ideas and share that with people to explain it.
Sigelman: Honestly, the only thing that’s really coming to mind, but it is coming to mind is parenting. I think that this has been like ultra-intense parenting year, and it is a learning thing. Like, how do you help your kids learn and experience the world when their world is so much narrower than it usually is? I think we’ve tried to do what we can. It’s interesting that finally things started opening up, and we took my 3-year-old to an aquarium basically, and we thought this could be so amazing. He used to love the aquarium in the before times or whatever. He had his mask on, and everything. He was initially excited to get there. Then after like 20 minutes, he’s like, I want to go home. It was like too much learning, like too much stimulus. It was very interesting. I’ve been thinking a lot about just in terms of learning about just the amount of stimulation and the type of stimulation that we receive. How if you suddenly make some giant change on that front, I think it can be very overwhelming for people. I don’t know if that makes any sense. It’s certainly not related to observability. It’s something I’ve been thinking about a lot this year, as everyone’s just been so narrowed in on whatever it is that we do. It’s much more focused than normal.
Butow: I think it definitely makes sense in the world of understandability as well, because sometimes just being overloaded with stimulation can be really confusing. It’s nice to have a simple way to be able to understand things, especially when you are working in very complicated distributed environments, not only systems but also people, it becomes very hard.
Egan: I think work just permeates everything that I do these days. In some way or another, I feel like I look at the entire world through the lens of incident response and incident management. The big thing that usually comes out for me around that is immediate prioritization of things and ways that my wife and my son definitely don’t like, where we’re interacting with an issue. You go, why is this really a SEV1? Let’s be careful here. I have a 3-year-old, and so, it’s similar in the parenting world, when we’re trying to deal with something, we’re trying to go out the door, and we really don’t want to put our mask on, or we really don’t want to put our shoes on. There’s just screaming that you could hear down the street and around the corner. It’s like, let’s take a moment, and let’s look at the data. Let’s figure out whether the impact here is actually justifying the degree of response that we’re putting into it. It’s really funny, because I think 5 or 10 years ago, a lot of people who were really big junkies into the task management space, had a very similar approach to the world. Where it was like, task management is really just to-do lists, and to-do lists work everywhere. I’m using Asana at home to run my family. I think a lot of people try to go out and do that for a little while.
I’ve ended up doing the same thing with incident management, where it’s like, mentally, in my head, I had this whole incident graph, and I want to put something up on the refrigerator that’s like, ok, how many SEV1s did we have last week? Let’s talk about them. Are we going to write anything up? Are we going to work on it? The reason I think it permeates similar to the task management space, is there’s some truth to it. You can apply a lot of the blameless approaches to dealing with major incidents in the workplace that we’ve absorbed into products and best practices, for things like incident management. You really can’t absorb those into your day to day life. You really realize how aggressively human nature fights against a lot of our learnings. We really don’t want to do ‘be blameless’ in our day to day lives. It’s very difficult to step back and say, these things probably went wrong, whatever they are, not having our shoes on, that’s if we’re going outside. All the way out to something bigger, like forgetting to pay a bill or something like that. The very natural and easy reaction is always to just say that’s your fault, or that’s my fault, or that’s this person’s fault.
I think the big learning that I’ve taken away from a lot of this, and working in this space more directly over the last couple of years, has really been this idea of look for the systemic cause. If you address that systemic cause you don’t alleviate yourself of accountability, you’re still accountable towards the thing that happened, but you’re actually fixing the thing that caused you to act in that way. Very much just like, I think Sidney Dekker, in one of his books, says, most people don’t come to work to do a bad job every day. If you go into every conversation about incidents with that in mind, you’ll come out stronger. I think it’s very much the same in personal life, parenting. I think it’s the same in cycling. I think it’s the same in all of these different hobbies and things that I do is, people in this room are doing what they’re doing because they want to do a bad job. Everyone’s here to try and do well and have a good time and live well. That’s the way it permeated inwards and helps me stay cognizant of focusing on that aspect of day to day life and hobbies.
Butow: It makes me think about my own life as well. My husband, he’s a game designer. Me coming from being on-call for many years, looking after distributed systems, making sure they’re up and running. He’s more about making sure that things are fun, and enjoyable, and really just awesome experience. That’s what he does. He’s been doing that for such a long time as well. For me, it’s funny because I’ll focus on what we need to prioritize and what we need to get done. Then he’ll balance it out and make sure that even if it is super high priority, we need to get it done within the next hour. It’s still fun and enjoyable. I think that’s cool how you can balance the two things together.
Systems Mirror the Organizations That Build Them
We do have a question about games as well, a little bit. In the board game space, there is an ongoing debate about balancing the setting of a game and the systems or mechanics implemented. Often, game systems set in a colonial period bring up conversations about conflicts between the observations of a system and mechanics, and the themes abstracted from the system setting. Are there problematic themes, assumptions birthed from the abstractions built with modern day tooling for services?
Yee: I don’t know that I would say that there are necessarily problems. I think that the way we think about breaking things into services largely affects what we build. You can think of Fred Brooks, and his whole concept that the systems that we build mirror the organizations that build them. If you decide that you’ve got a giant organization, and you’re going to break it into different teams, those teams suddenly become your services. The way that those services connect, they’re mirrors of the way that your teams connect. That can be problematic. I think the problems come when we presuppose that we’re going to be in a certain framework, and that things have to be a certain way. From my own experience, this often replicates in the fact that we have the database team. Your team doesn’t own the data. They always have to go talk to that database team, because we’ve naturally set it up that there’s a team that manages the database, and they’re the keepers of all the data. Naturally, then you start to have this abstraction of like, then I don’t control the data that my service needs. Now I have to think of an abstraction layer of, how do I talk with that database team? I think that, yes, the way that we set things up naturally impacts the way we think about things, and it can create some friction.
Sigelman: This is in some ways exactly what I’m getting at with this idea of resources versus transactions. We want to be able to talk about the health of individual transactions, that’s the point. That’s what our customers care about. You can almost see these movements occurring in our technology ecosystem, where we’re trying to make the resources align better to the transactions. In some ways, that’s what things like Kubernetes are all about, like the idea of a service, it can get more abstract. You’re talking about serverless, when it’s literally just a function call that we’re abstracting. The trouble is that you can’t get away from the fact that at the end of the day, there are physical resources being consumed, and those crop up in reality. It turns out that serverless is a wonderful abstraction, but the cost of making a network call is still way higher, probably a thousand to a million times higher, depending on what network you’re on, than accessing something on local disk, or something like that.
You run up against the reality that if you try to make the schema that you’re using to understand and abstract your system. As you try to make that as friendly as possible, you drift further from the physical reality of your resources. Those, unfortunately, dictate performance and the cost profile of your system. I think this push and pull has been happening for decades, and will continue for decades. It’s a great way to think about the talk I was giving around resources and how they relate to transactions in the first place.
Egan: I think a lot of the abstractions we see today, when we build abstractions out, we’re really trying to compartmentalize complexity. We’ve got complex systems, then we build another system above it that’s simpler, that then houses that complex system, and another system above that, that houses that complex system. There’s the law of stretched systems, which was Rasmussen back in the ’80s, was talking about this, where every time you do that, you’re just going to stretch the new system until it breaks. Then you’re going to build another abstraction, you’re going to stretch that system until it breaks, and you’re going to keep building up. I’ve always found that the biggest risk around those abstractions is you stop working to optimize the system that you abstracted in many cases. Because now you’re going to have to start focusing on the breaking points of the new system you’ve built, on top of that. I think this translates really cleanly to tools. Otherwise, in my field, in incident management, it really translates directly to the increase in this butterfly effect of, one small thing done now propagates across these abstractions and down, and actually increases in order of magnitude each time that it works its way down.
I think it was in the ’50s or so when some of this was being researched, it was the difference between the mistake of pushing a wrong key on a typewriter and pushing the wrong key on a computer. Your impact of a mistake at that typewriter key is very limited and very small because you’re in that base system. Now that you abstracted so many levels of software and tooling and otherwise all the way up in the computer world and you’ve clicked a key, you might have accidentally just brought down an entire data center, or otherwise. I was saying the other day, we need child locks on keyboards now that everyone’s working at home, because the risk is so high. I think there’s a huge risk there as we do tooling and as we continue to abstract out, where the difference between building software today, and trying to understand it is now equally as complex as the infrastructure that we’ve gone out and built up. Maybe that’s one of the reasons observability is such a big deal now. I just think it’s fascinating that that problem is just going to continue to propagate. I don’t think there’s an end to it.
How to Interview for Observability Skills
Butow: How do you think that we should interview for observability skills? What are your thoughts there?
Sigelman: As a practitioner, you mean, so interviewing someone who would lead like an observability team or something like that? Is that the idea?
Butow: Yes.
Sigelman: Of course, we interview people all the time at Lightstep. That’s the type of observability interview, but not the same type of observability interview. I think what I’d really want to see would be a focus on the outcomes of observability and the ability to articulate how those connect to the practice of it. In my head, there’s some hierarchy of observability. At the very bottom layer, you have code instrumentation. Then above that, you have telemetry egress, and then telemetry storage, and then data integration. Then, maybe UI/UX, and then above that, like automation. The conversation is way at the bottom of that stack right now, and it’s not valuable. Observability is only valuable if it accelerates your velocity, or improves your time with an SLO. Those are the only things that matter. If you can’t actually describe how all that stuff at the bottom actually affects the stuff at the top, I’m not that excited.
Of course, there’s no one true path. There’s a lot of open questions, and so on. I’d want to see someone be able to navigate that, not just to talk about the goals like, yes, we want faster velocity, but how do you do that with observability? To describe it in practical terms, and going all the way up and down that stack. That’s what I would really be looking for. I think you have people who talk in hyperbole about the top of the stack, and people who get lost in the weeds at the bottom of the stack, but it’s rare to find someone who can actually connect those dots in a really clear and coherent way.
Butow: Those are some great tips for folks who might be doing interviews around observability in the future. It makes me think just pulling up, if you’re able to draw a diagram as well of that pyramid, and talk through each of the items, as you’re doing your interview. It’s a really great way to explain to the person that you’re interviewing with, your thoughts and your frameworks that you use.
Interviewing for Incident Management Understandability
I’d love to ask the same question but about understandability in regards to incident management in general. What would you interview for there? What are you looking for?
Egan: I think when we’re talking to people about understandability with respect to this space, we’re really thinking a lot about translating active and emotional cases into practical systemic solutions. It’s a little bit of a EQ when you’re interviewing around that space. Because it’s an ability to walk that line between overly technical root cause analysis that results in potentially the system level task changes that need to go in to the organization to improve state versus the cultural and process changes that need to be implemented. The airline industry has gotten really wonderful at this, over the years in terms of, how do you train people into incident management regarding the response, but more importantly, the then learnings and understandability on the other end of it? In such a way that the learnings and reasonings propagate out into the industry and the organization in a practical manner. It’s the best way I can describe it. You’re really looking for an ability there to translate things into consumable and actionable results, not just to go and write novels like at the outcomes. I think we look really closely at that when we talk to people about how to hire into this space really, when you’re looking for your incident commanders, or IMOCs that we had at Facebook. There’s an interesting difference there, Facebook, Google, that I think you can dig really deeply into and have pretty deep conversations with folks. If you don’t have both, you won’t actually solve the long term resiliency challenges that you’re trying to work through.
Butow: I know a lot of folks have probably written up postmortems. The next big step is like, what are we going to change after this incident happened and we’ve written up this postmortem? What are our action items? How are we going to make sure those get done? What did we actually do? Then afterwards, did they actually help us improve? It’s really much more of a nicer circle instead of just ending it at the postmortem. That’s a great thing for folks to think about. I’m going to circle back as well to talk more about IMOCs in that incident manager role. I think that’s a really interesting conversation for us to have as well.
Interviewing for Someone Who Understands How to Observe and Understand Failure
You have a lot of experience in chaos engineering. What would you interview for when you’re looking for someone who understands how to observe and understand failure?
Yee: I think it’s a little combo of both what Ben and John said. Previously, when I was working at Datadog, and things, like observability in and of itself isn’t the end goal. I think one of the challenges when I’m looking for people to hire, is that understanding of having a clear idea of how you fit into this system. What’s the ultimate end goal? What’s your contribution to it? Because if you can’t define that, then you have no direction in what you’re doing. Having that overall understanding means that I can trust you, given whatever incident happens, that you’ll know what to prioritize, and you’ll know how to act on that. I think that’s a big thing, even when it comes to chaos engineering. We talk about reliability, but it’s a pretty big blanket. What do we make reliable? How do we focus in on the things we need to be improving, especially with chaos engineering? Because it’s this fun thing, but you can go around breaking anything, and having no impact on the system and improving nothing? You’ll have a lot of fun doing it. It comes down to that, like, where do I fit in the system? How do we look at a system and get the most benefit from what we’re doing? The rest of it, the chaos engineering and the tools, that’s pretty straightforward.
Butow: It actually makes me think of a saying that I think is from Facebook. I used to always hear, focus on impact. Is that a Facebook thing? Yes.
Egan: That was definitely a red letter poster, one of the big ones on the walls. Yes.
Butow: I used to always hear that at Dropbox, when we were talking about incidents, postmortems, like doing chaos engineering. It was always like, focus on impact. Make sure that what you’re doing is positively impactful in a good way. It’s going to actually help move the needle, we always would say that. I really like that idea.
Incident Manager On-Call (IMOC)
Now let’s circle back to the idea of an Incident Manager On-Call, an IMOC. That’s what we called it at Dropbox. I know at Amazon they’ve also called it a call leader. I didn’t actually know that IMOC originally comes from Facebook. What’s it called at Google? Is it the same or is it different?
Sigelman: Google, despite having many SREs, there were different terms in use in different teams. I also left the company in 2012. The term incident commander, I definitely heard in my time at Google, but I’m not sure if it’s something that was totally standardized. The Search SRE team behaved and operated in a totally different way than the Gmail SRE team. I can go into the whys of that. I think it was all for good reasons. I don’t think that was as standardized as it was at Facebook, in my experience anyway.
Butow: Would you like to tell us about that, what’s it like at Facebook, having an IMOC?
Egan: Just to set some context for folks listening, there’s a couple of different terms for what arguably could be called the leader during an incident, but has different terms as it’s happening. One of the more popular ones I think you see on a lot of organizations’ sites are the incident commander, which Google had in their book in 2016, on SRE, defined pretty directly. I’m not sure if that evolved over time, or if maybe that’s just the public version that they wanted to put out. At Facebook, we practiced a slightly different role that was called the IMOC, which was the Incident Manager On-Call, and who we internally joked was the responsible adult in the room. The core difference between those roles, at least as I saw it, was that the incident commander role was a very hierarchical and almost militaristic type role. If you go look at the definitions, and these vary on every company’s site, but for example, PagerDuty has a set of definitions of how an incident commander should run. They’re very aggressive. It’s very much, incident commander, ask engineer responder one, is problem solved? If engineer responder one does not respond with a yes or no, remove engineer number one from call, insert engineer number two. It’s a very aggressive approach to dealing with the micromanagement almost of an incident.
The IMOC, I always felt lended itself a little bit better to multiple leadership roles. Where you had an IMOC who was very well trained in incident management processes, and can help to make sure things like leveling of the server correct, can help to reach into the organization and across the organization to folks who maybe aren’t contactable. The advantage of that is it permits the incident itself to also have a separate role that you might call the owner. That owner doesn’t necessarily have to be someone who’s perfectly trained. It’s simply the person who’s currently responsible for the incident as it’s being resolved. It’s a little bit more of a team effort. In an ideal world, the IMOC might do nothing other than just be present and aware of the incident happening, while the owner is actually going out and doing the work. In a more complex world, the IMOC might be coming in and helping reach across the org, if it’s a PR impacting issue, or if it’s maybe dealing with a cross team, and it’s a new engineer who’s being the owner.
I’ve actually found running Kintaba, that the incident commander role is far more adopted, I think, across organizations today, if you were to go and take the entirety of organizations, I think in the Valley, and compare what they call that role. I think incident commander has taken root, much more aggressively. I wish it would start to swing the other way, because I find the roles to be more about distributing accountability across the company, to the various owners. Versus just saying, ok, everything comes back onto the incident commander’s shoulders. Not to phrase that as if that’s what happens at Google. That’s what I see it having translated into, in a lot of other parts of the industry.
Butow: I’ve definitely seen lots of companies taking up this role. I myself was an IMOC at Dropbox for a long time as well. Yes, you’re responsible for every single system. You have to know about everything. It’s a pretty big job. Also, it’s a 24/7 rotation, which is interesting too.
Fair Retention Time for Tracing
Butow: What is your thoughts on a fair retention time for tracing? Do you have a quick answer there?
Sigelman: The question itself said that the value of tracing data falls over time, since they’re less useful for identifying problems for possible improvements. Yes, it is true. I really want tracing sampling, which is what we’re talking about here, to be based less on properties of the request itself, which is how things are done right now, and more based on hypotheses that are being examined or other things that are of interest. Like an SLO, for instance. If a trace passes through an SLO, we need to be able to hydrate that SLO with enough traits and data to be able to do real comparisons between an incident that’s affecting the SLO and a baseline time when things are healthy. Even in healthy times, having a large volume of tracing data is quite useful for those sorts of comparisons. I want the sampling to be based on things like SLOs or CI/CD. Like we do a new release, we want to up this trace sampling rate. It’s not just about time, it’s about whether or not those traces are involved in some change, planned or unplanned, that’s of interest.
Something Everyone at Every Company Should Do
Butow: What’s something that you wish everyone did at all companies?
I actually wish every company paid people extra for on-call over the holidays, if they work that shift. Like if you work New Year’s Eve, you should get paid extra for doing that on-call shift.
Egan: I’ll be selfish in the incident space. I think companies should directly reward people who file the most incidents. I don’t care if it’s gamed, I still think it should be done.
Butow: I think that’s great. Reward and recognition is important.
Yee: Companies should spend more time and give employees time to actually explore. We think of chaos engineering largely as reliability, but I like to think of it as just exploring and learning. I think that that’s something that engineers need more of.
Sigelman: One of our values internally is being a multiplier, and it’s a difficult thing to reward people for. It can be measured in certain ways. I think if a service is written well and thoughtfully, and SLOs are determined well and thoughtfully, the service will contribute a lesser portion to the ultimate amount of user visible outages, and you’re being able to apply it for the product and for the rest of the organization. I would love to see people rewarded for doing more than their fair share in overall reliability of the system, because it’s a hard thing to notice when it’s happening. It’s very important, I think, overall.
See more presentations with transcripts
NoSQL: Market 2022 Trends, Innovation, Growth Opportunities, Demand, Application … – Industrial IT
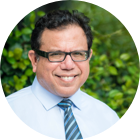
MMS • RSS
Posted on nosqlgooglealerts. Visit nosqlgooglealerts
Qurate’s most recent research study on NoSQL Market 2022 was performed by extremely experienced research professionals and business experts in order to offer an in-depth assessment on the NoSQL. The key sources of information consist of several NoSQL industry professionals, suppliers, manufacturers, associations along with business distributions. The research report defines exclusive benefits of the various market size, shares, and the patent industry. The key aim of the NoSQL research report is to offer the latest updated statistics such as the market share, size, trends, evolving markets, earnings, historic and forecast figures, and data on leading market players. The NoSQL research study provides significant and critical information which is necessary for strategic decisions and to have a competitive edge.
The key players included in the NoSQL research report includes –
SAP
MarkLogic
IBM Cloudant
MongoDB
Redis
MongoLab
Amazon Web Services
Basho Technologies
Google
MapR Technologies
Couchbase
AranogoDB
DataStax
Aerospike
Apache
CloudDB
MarkLogic
Oracle
RavenDB
Neo4j
Microsoft
For the detailed sample pages link please visit –
Sample pages are a PDF document covering the detailed Table of Contents along with the blueprint of charts, graphs, figures, and tables to give you a flavor of the final report. Please note that the sample pages may not comprise of actual figures.
In view with the ongoing pandemic our analysts have thoroughly scrutinized and presented the below parameters under the detailed Covid – 19 impact analysis in the NoSQL research report:
- Impact on Market Size
Analysis on the overall impact of Covid – 19 on the globe which will include quantitative data wherein we include the estimated gap in the market size (negative or positive) due to the pandemic.
- End-User Trend, Preferences, and Budget Impact
Qualitative data as to the trends in the end-user segment due to the imposed policies and safety guidelines are analyzed in the NoSQL research report. In addition, a detailed understanding on the preferences at the consumption end as to what type/technology the end-user adopts is also studied in the report. The additional funding provided by legal authorities in also included to provide information on a particular industry vertical to kickstart the economic development.
- Regulatory Framework/Government Policies
Detailed qualitative analysis on the government policies and safety guidelines followed by each country are studied to understand different authorities’ views and opinions used to regulate the impact caused by Covid – 19.
- Key Players Strategy to Tackle Negative Impact
The overall business strategies adopted by key companies in the Covid – 19 situations are analyzed and documented in our research studies. The information is presented in the either qualitative or quantitative format in the NoSQL research report.
- Opportunity Window
The opportunities that Covid – 19 presents to the NoSQL players and industry professionals are mentioned to give a detailed understanding on the next best possible profitable solutions.
Years Studied to Estimate the NoSQL Market Size are as under:
History Year: 2015-2019
Base Year: 2020
Estimated Year: 2021
Forecast Year: 2022-2026
The NoSQL research report also encompasses the conditions that impact the industry. It also consists of the growth drivers and difficulties faced by the NoSQL industry. The research report includes detailed segmentation analysis along with several sub-segments.
Segmentation of the NoSQL –
on the basis of types, the NoSQL market from 2015 to 2025 is primarily split into:
Key-Value Store
Document Databases
Column Based Stores
Graph Database
on the basis of applications, the NoSQL market from 2015 to 2025 covers:
Retail
Online gaming
IT
Social network development
Web applications management
Government
BFSI
Healthcare
Education
Others
Regions Covered under the NoSQL include:
Asia-Pacific (Vietnam, China, Malaysia, Japan, Philippines, Korea, Thailand, India, Indonesia, and Australia)
North America (The United States, Mexico, Canada, etc.)
South America (Brazil, Colombia, etc.)
Europe (France, Germany, Russia, UK, Italy, etc.)
Rest of the World (GCC and African Countries, Turkey, Egypt, etc.)
20% free customization – If you want us to cover analysis on a particular geography or segmentation which is not a part of the scope, kindly let us know here so that we can customized the report for you.
To Purchase the Full NoSQL Research Report with Detailed Market Analysis Along With Covid – 19 Impact Analysis Please Visit
Purchase FULL Report Now! https://www.qurateresearch.com/report/buy/ICT/global-nosql-market/QBI-MR-ICT-1082925
Key Questions Answered in the Report –
- Who are the global industry players of the NoSQL and what is their market share, net worth, sales, competitive landscape, SWOT analysis and post Covid – 19 strategies?
- What are the prime drivers, growth/decline factors and difficulties of the NoSQL?
- How is the NoSQL industry expected to emerge through the pandemic and through the forecasted period of 2022 – 2026?
- What are the supply patterns across the various regions mentioned in the NoSQL research report?
- Is there been a change in the regulatory policy framework after the Covid – 19 situations?
- Which are the prime areas of applications and product type that are going to expect a surge in the demand during the forecast period 2022 – 2026?
(*If you have any special requirements, please let us know and we will offer you the report as you want.)
Note – In order to provide more accurate market forecast, all our reports will be updated before delivery by considering the impact of COVID-19.
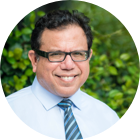
MMS • RSS
Posted on mongodb google news. Visit mongodb google news
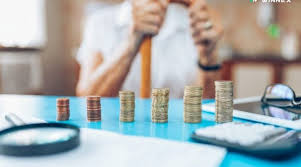
MongoDB Inc. (NASDAQ:MDB) shares, rose in value on Wednesday, 01/26/22, with the stock price down by -3.81% to the previous day’s close as strong demand from buyers drove the stock to $351.57.
Actively observing the price movement in the last trading, the stock closed the session at $365.48, falling within a range of $345.35 and $393.84. The value of beta (5-year monthly) was 0.65. Referring to stock’s 52-week performance, its high was $590.00, and the low was $238.01. On the whole, MDB has fluctuated by -37.13% over the past month.
3 Tiny Stocks Primed to Explode
The world’s greatest investor — Warren Buffett — has a simple formula for making big money in the markets. He buys up valuable assets when they are very cheap. For stock market investors that means buying up cheap small cap stocks like these with huge upside potential.
We’ve set up an alert service to help smart investors take full advantage of the small cap stocks primed for big returns.
Click here for full details and to join for free.
Sponsored
With the market capitalization of MongoDB Inc. currently standing at about $24.31 billion, investors are eagerly awaiting this quarter’s results, scheduled for Mar 07, 2022 – Mar 11, 2022. As a result, investors might want to see an improvement in the stock’s price before the company announces its earnings report. Analysts are projecting the company’s earnings per share (EPS) to be -$0.22, which is expected to increase to $0.02 for fiscal year -$0.73 and then to about -$0.56 by fiscal year 2023. Data indicates that the EPS growth is expected to be 26.30% in 2023, while the next year’s EPS growth is forecast to be 23.30%.
Analysts have estimated the company’s revenue for the quarter at $241.76 million, with a low estimate of $240.5 million and a high estimate of $245.09 million. According to the average forecast, sales growth in current quarter could jump up 41.40%, compared to the corresponding quarter of last year. Wall Street analysts also predicted that in 2023, the company’s y-o-y revenues would reach $849.36 million, representing an increase of 43.90% from the revenues reported in the last year’s results.
Revisions could be a useful indicator to get insight on short-term price movement; so for the company, there were no upward and no downward review(s) in last seven days. We see that MDB’s technical picture suggests that short-term indicators denote the stock is a 50% Sell on average. However, medium term indicators have put the stock in the category of Hold while long term indicators on average have been pointing out that it is a 50% Sell.
19 analyst(s) have assigned their ratings of the stock’s forecast evaluation on a scale of 1.00-5.00 to indicate a strong buy to a strong sell recommendation. The stock is rated as a Hold by 4 analyst(s), 14 recommend it as a Buy and 0 called the MDB stock Overweight. In the meantime, 1 analyst(s) believe the stock as Underweight and 0 think it is a Sell. Thus, investors eager to increase their holdings of the company’s stock will have an opportunity to do so as the average rating for the stock is Overweight.
The stock’s technical analysis shows that the PEG ratio is about 0, with the price of MDB currently trading nearly -19.83% and -27.61% away from the simple moving averages for 20 and 50 days respectively. The Relative Strength Index (RSI, 14) currently indicates a reading of 27.89, while the 7-day volatility ratio is showing 9.40% which for the 30-day chart, stands at 6.95%. Furthermore, MongoDB Inc. (MDB)’s beta value is 0.82, and its average true range (ATR) is 29.83. The company’s stock has been forecasted to trade at an average price of $572.21 over the course of the next 52 weeks, with a low of $461.00 and a high of $700.00. Based on these price targets, the low is -31.13% off current price, whereas the price has to move -99.11% to reach the yearly target high. Additionally, analysts’ median price of $558.00 is likely to be welcomed by investors because it represents a decrease of -58.72% from the current levels.
A comparison of MongoDB Inc. (MDB) with its peers suggests the former has fared considerably weaker in the market. MDB showed an intraday change of -3.81% in last session, and over the past year, it grew by 2.33%%. In comparison, Progress Software Corporation (PRGS) has moved lower at -0.07% on the day and was up 4.78% over the past 12 months. On the other hand, the price of Pixelworks Inc. (PXLW) has fallen -0.32% on the day. The stock, however, is off -2.52% from where it was a year ago. Other than that, the overall performance of the S&P 500 during the last trading session shows that it lost -0.15%. Meanwhile, the Dow Jones Industrial Slipped by -0.38%.
Data on historical trading for MongoDB Inc. (NASDAQ:MDB) indicates that the trading volumes over the past 3 months, they’ve averaged 970.63K. According to company’s latest data on outstanding shares, there are 66.39 million shares outstanding.
>> 7 Top Picks for the Post-Pandemic Economy
Nearly 2.70% of MongoDB Inc.’s shares belong to company insiders and institutional investors own 90.40% of the company’s shares. The stock has fallen by -33.58% since the beginning of the year, thereby showing the potential of a further growth. This could raise investors’ confidence to be optimistic about the MDB stock heading into the next quarter.
Article originally posted on mongodb google news. Visit mongodb google news
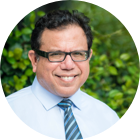
MMS • Ben Linders
Article originally posted on InfoQ. Visit InfoQ
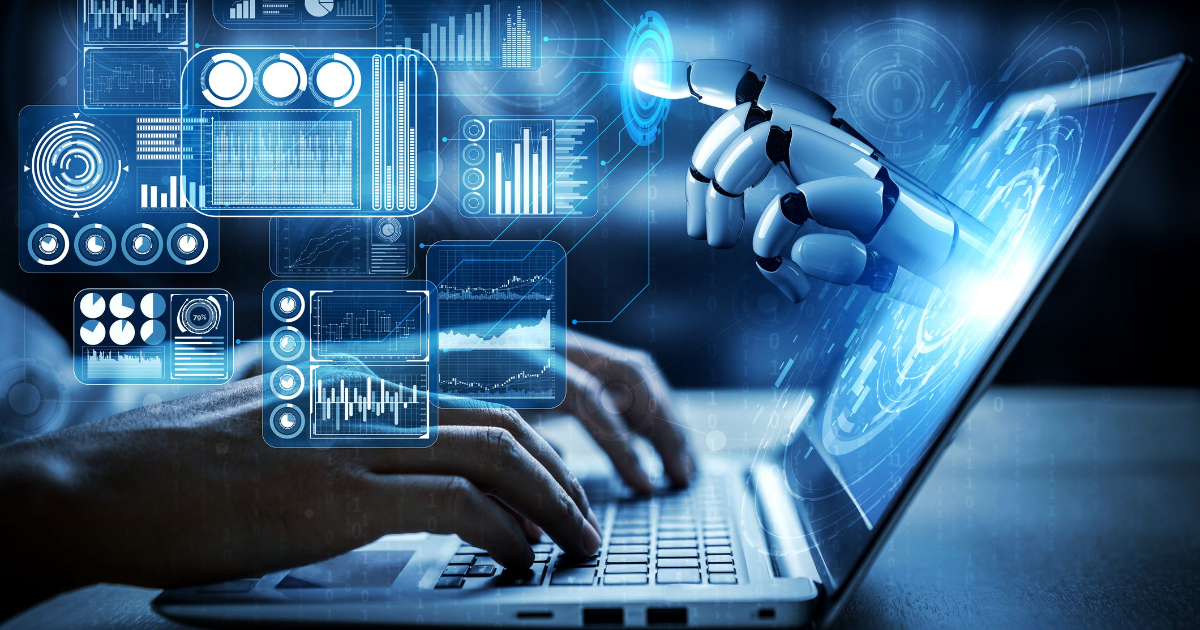
AIOps is all about equipping IT teams with algorithms that can help in quicker evaluation, remediation or actionable insights based on their historical data without the need to solicit feedback from users directly. AI can help IT operators to work smart, resolve issues faster and keep the systems up and running to deliver great end-user experience.
Rajalakshmi Srinivasan spoke about the impact that artificial intelligence has on IT operation management at DevOps Summit, Canada 2021.
Artificial Intelligence (AI) and Machine Learning (ML) techniques have almost impacted every field or industry around us, particularly those that deal with massive data, Srinivasan mentioned. Among those, IT Operation Management (ITOM) has been one of the earlier adopters of AI & ML due to the sheer volume of data generated by IT operations, she said.
One of the primary objectives of ITOM is to send alerts to IT teams when the server/application goes down or when the response time exceeds the defined threshold value or for any incident in the system. Srinivasan gave an example of how AI can help operators to manage a flurry of alerts:
Alerts should be categorized and assigned to relevant technicians based on severity, and degree of business impact. At Site24x7, these alerts are automated and managed by AI algorithms by continuous training and learning from various user actions thus reducing the Mean Time To Repair (MTTR).
With the wide adoption of the cloud, the industry is working towards 99.999% uptime of all its resources, Srinivasan said. This involves many meticulous and mundane processes such as running scripts to perform corrective actions like restarting a process, clearing logs, stopping a service, invoke an URL/Rest API to create a ticket/incident, rebooting virtual/cloud VM, and many more. AI can be used for causal analysis and corrective action, as Srinivasan explained:
Thresholds need not be fixed user configurations; instead they are AI enabled custom values that vary for various parameters. The criteria for each of these actions are also different. If the disk utilization threshold is spiking than its usual range, the AI engine detects this as an anomaly and invokes the disk clearing action. If a new alarm/event is found, the AI engine identifies this and triggers the ticket/incident creation task.
Most of the corrective actions can be automated based on various predefined criteria and AI can be a breather in such repetitive scenarios, Srinivasan concluded.
InfoQ interviewed Rajalakshmi Srinivasan about applying AI in IT operations management.
InfoQ: What is the state of practice of AI in IT operations management? What are the possibilities?
Rajalakshmi Srinivasan: Anomaly detection, outage prediction, natural language processing, root cause analysis, seasonality trend analysis, and capacity planning are a few AI & ML techniques that come in handy in ITOM. Let me explain with some practical use cases.
For capacity planning, based on the past performance values of the disk utilization and how it grows, predictions can be made to forecast the disk usage. We have had instances where AI simply outweighed the regular static approach of extrapolating the data by providing seasonality trends and insights in the data. What we observed is that the values will not always be on the increasing side and it may show a decreasing trend towards the end of every month. Sometimes the value will increase only during the weekends and become normal during the weekdays. These details have been captured in our AI-based forecasting, which helped us smoothen the irregularities in the data collected leading to precise predictions.
AI techniques have also helped us with automatic anomaly detection whenever there is a drastic deviation to the metrics collected due to various reasons, such as a sudden increase in the number of requests to the website, the response time of a web transaction spiking to 4x from its usual range, the JavaScript (JS) error count from a particular geographical region being high, the number of archiving tasks being reduced from its normal count, and more… In all these situations, we greatly depend on AI techniques for automatic anomaly detection in our monitoring systems.
InfoQ: How does AI support IT operators?
Srinivasan: There are numerous ways AI and ML techniques support IT operators. Let me explain a few use cases in our day-to-day work.
Applying dynamic thresholds: defining thresholds for the metrics (response time, CPU usage, request count) collected can be easily automated with help from historical data. This dynamic thresholding not only helps us with operational accuracy & efficiency, but also in optimal resource allocation.
For instance, Site24x7’s web application has multiple distinctive grids for client access, rest API requests, archiving, data collection, data processing, and so on. The response time will vary for each of these grids. A background scheduled task in an archiving grid will take more time, but customer-facing client requests will need an instant response. And even within the same grid, multiple transactions will have different bench-marked response time values.
In these scenarios, we cannot define a constant threshold for all the grids or for all transactions within the same grid. This is a use case where AI & ML has helped us with dynamic thresholds without any user intervention.
Enhanced communication using chatbots: gone are the days where we had to log in to various monitoring tools to know the status of the system. Today, with chatbots, these communications are enhanced and integrated in such a way that we can seamlessly make a simple natural language query from our chat application (Microsoft Teams, Slack, Zoho Cliq) and get the status.
Natural Language Processing (NLP) is the AI technique used in combination with API calls to fetch the required data and act on it wherever required.
InfoQ: What do you expect that the future will bring for AI in operations?
Srinivasan: Like any other developing technologies, AI in IT operations is an ongoing process trying to improve the system to become more efficient and productive. Some of the enhancements will be aimed at:
- Achieving accuracy in anomaly detection and false alerts
- Being proactive and preventing an issue from occurring, rather than being reactive and resolving the issue after it has occurred
- Self-training systems to make precise predictions
- Increasing use of Deep Neural Network Algorithms in place of machine learning techniques to narrow down and pin-point problems/issues
- Availing AI and ML as a Service to create meaning out of the enormous data collected
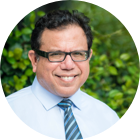
MMS • Jim Walker Yan Cui Colin Breck Liz Fong-Jones Wes Reisz
Article originally posted on InfoQ. Visit InfoQ
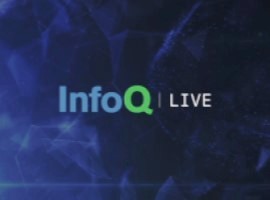
Transcript
Reisz: What do you think of when I say cloud native and scaling? Do you think of tech, maybe things like Kubernetes, serverless? Or maybe you think about it in terms of architecture, microservices, and everything that entails with a CI/CD pipeline. Or maybe it’s Twelve-Factor Apps, or maybe more generally, just an event-driven architecture. Or maybe it’s not even the tech itself. Maybe when you hear scaling and cloud native, it’s more about the cultural shifts that you need to embrace, things like DevOps, truly embracing DevOps, so you can get to things like continuous deployment and testing in production. Regardless of what comes to mind when you hear scaling cloud native apps, Here Be Dragons, or simply put, there are challenges and complexities ahead. We’re going to dive in and explore this space in detail. We’re going to talk about lessons, patterns. We’re going to hit on war stories. We’re going to talk about security, observability, and implications around data with cloud native apps.
Background, and Scaling With Cloud Native
My name is Wes Reisz. I’m a Platform Architect working on VMware Tanzu. I’m one of the co-hosts of the InfoQ podcast. In addition to the podcast, I’m a chair of the upcoming QCon Plus software conference, which is the purely online version of QCon, comes up this November. On the roundtable, we’re joined by Jim Walker of Cockroach Labs, Yan Cui of Lumigo, and The Burning Monk blog, Colin Breck of Tesla, and Liz Fong-Jones of Honeycomb. Our topic is scaling cloud native applications.
I want to ask you each to first introduce yourself. Tell us a little bit about the lens that you’re looking at that you’re bringing to this discussion. Then answer the question, what do you think of when I talk about scaling and cloud native all in one sentence?
Walker: I’m originally a software engineer. I was one of the early adopters of BEA Tuxedo. Way back in ’97, we were doing these kinds of distributed systems. My journey has really been on the marketing side. I might have started as a software engineer, and always data and distributed. I moved into big data. I was Talend. I was Hortonworks. I was early days at CoreOS. Today I’m here at Cockroach Labs, and so really the amalgamation of a lot of things.
When I think of cloud native, honestly, it was funny when you asked me this earlier, I was like, I think of the CNCF. I think about this community of great people that do some really cool things, and a lot of friends that I’ve made. Then I had to really think about, what does it mean for the practitioner? It’s seemingly simple, but practically extremely complex and difficult to do. When I think cloud native, I think there’s lots of vectors that we have to think about. When I think about scale, in particular, in cloud native, as this is about, is it scale of compute? Is it scale of data? Is it scale your operations and what it means to observability? Is it eliminating the complexities of scale? There’s just so many different directions we can go in, and it just all leads back to this like, practically and pragmatically, it’s extremely complex. I think we’re trying to simplify things and I think we are getting a lot better and we are actually seeing huge advances in simplification, but it’s a complex world. That’s the most generic.
Breck: I’m Colin. I spend my career developing software systems that interact with the physical world, so operational technology and industrial IoT. I work at Tesla at the moment, leading the cloud software organization for Tesla Energy. Building platforms focused on power generation, battery storage, vehicle charging, as well as grid services. This includes things like the software experience around supercharging, the virtual power plant program, Autobidder, and the Tesla mobile app, as well as other services.
When I think about scaling cloud native, I don’t think of technologies, actually, I think about architectural patterns. I think about abstracting away the underlying compute, embracing failure, and the fact that that compute or a message or these kinds of things can disappear at any time. I think about the really fundamental difference between scaling stateful, and so-called stateless services. That’s a real big division there in terms of decision making and options in your architecture. Then in IoT, specifically, I think a lot about architectures model physical reality, and eventual consistency, failure, uncertainty, and the ease of modeling something and being able to scale it to millions, is a real advantage in IoT.
Fong-Jones: I’m Liz Fong-Jones. I’m one of two Principal Developer Advocates now at Honeycomb. Before Honeycomb, I spent 11 years working at Google as a site reliability engineer. What I think about with regard to what cloud native is, I think it relates to two achievable practices, specifically around elasticity and around workload portability. That if you can move your application seamlessly between underlying hardware, if you can scale up on demand, and within seconds to minutes, not tens of minutes, I think that that is cloud native to me. Basically, there are a number of socio-technical things that you need to do in order to achieve that, but those best practices could shift over time. It’s not tied to a specific implementation for me.
Cui: My name is Yan. I’ve been working as a soft engineer for 15 years now. Most of that working as an AWS customer, building stuff for mobile games, social games, and sports streaming, and other things on AWS. As for my experience, when I think about cloud native, I think about using the managed services from the cloud, so that offloading as much responsibility to the cloud provider as you possibly can so that you work on a higher level of abstraction where you can provide value to your own customers as engineer for your own business.
In terms of scaling that cloud native application, I’m thinking about a lot of the challenges that comes into it. How they necessitate a lot of the architectural patterns that I think Colin touched on in terms of needing high availability, needing to have things like multi-region, and thinking about resilience and redundancy. Applying things like active-active patterns, so that if one region goes down, your application continues to run. Some of the implications that comes into it, when you want to do something like that in terms of your organization, in terms of the culture stuff, I think, Wes mentioned in a sense. You need to have CI/CD. You need to have well defined boundaries, so that different teams knows what they’re doing. Then you have ways to isolate those failures, so if one team messes up, it’s not going to take down the whole system. Those other things around that, which touches on infrastructure. It touches on tooling, like observability. I think it all comes into a big ball of almost a complexity that lots of things need to tackle when it comes to scaling those applications.
Reisz: When I was putting this together, I came up with so many questions, and the best way to describe it, I just came up with that Here Be Dragons. It resonated with me when I put this together.
Is Cloud Native Synonymous with Containers?
When we talk about cloud native, is it synonymous with containers?
Cui: From my perspective, I do see a lot of, at least the dialogue around cloud native is actually focused on containers, which to me is actually weird. If you think about any animals or plants or anything that you think is native to the U.S., would you think the first thing that comes to mind is, that thing can grow anywhere, or it can live anywhere. It’s probably not. There’s something specific about U.S. that these things are particularly well suited to, and so they can blossom there. When I think about containers, one of the first thing that comes to mind is just portability. That you can take your workload, you can run it in your own data center. You can run it in different clouds, but that doesn’t make it native to any cloud. When I think about cloud native, I’m just thinking about the native services that allows you to extract maximum value from the cloud provider that you’re using, as opposed to containers. I think containers is a great tool, but I don’t think that should be cloud native, at least in my opinion.
Fong-Jones: That’s really interesting, because to me, I think about cloud native as a contrast to on-prem workloads. That the contrast is on-prem workloads that have been lifted and shifted to the cloud are not necessarily cloud native to me, because they don’t have the benefits of scalability. They don’t have the benefits of portability. I think that the contrast is not to portability between different cloud providers. I think it’s the portability to run that same workload to stamp out a bunch of copies of it, for instance, between your dev and prod environments. To have that standardization, so you can take that same workload and run it with a slight tweak.
Do You Have To Be On A Cloud Provider, To Be Cloud Native?
Reisz: I hear Liz and Yan talking about cloud providers, in particular. To be cloud native, do you have to be on a cloud provider?
Breck: No, I think that goes back to those architectural principles. Yes, actually, like Erlang/OTP, that’s the most cloud native you can get in some ways. That’s old news. That’s like abstracting away the underlying compute, embracing multicore, embracing distributed systems, embracing failure, those things. That’s the most cloud native you can get. Especially in IoT, in my world, the edge becomes a really important part of the cloud native experience. If from the edge, like a cloud is just another API to throw your data at, you’re not going to develop great products. If the edge becomes an extension of this cloud native experience, you can develop really good platforms. If you look at the IoT platforms from the major cloud providers, that’s the direction they’ve gone in this. There’s an edge platform that marries with what they have in the cloud. I think that cloud native thinking can extend beyond a cloud provider into your own data center, or out to the edge, or whatever you’re doing.
Reisz: I loved what Yan said about portability, because when I think cloud native, I often tend to think about containers. Yan’s right, it’s more about the portability. It’s about being able to move things. It’s not necessarily a specific technology. That may be hot today, may be popular today, but it’s really the portability.
Walker: Don’t just lift and shift. I talk to customers all the time about, you need to move and improve, because simply taking something and running it on new infrastructure is not cloud native. You’re running it somewhere else on somebody else’s server. That’s all that is. Containers allow us to do that. There’s a fundamental different approach to the way that you build software when you’re cloud native. It’s not just all the tools around it. You have to take into consideration, at least in my opinion, the physical location of what you’re doing. I think that’s this distributed mindset. Moving to this new world has to take that into consideration. We deal with this all the time. The speed of light is no joke. When you start talking about some of the things you were talking about, Colin, like eventual consistency, and are things active-active? The problem that we’re dealing with here is software engineering has advanced to the point where we’ve caught up with the speed of light. To be truly scalable across the globe, in real time, whatever that means for you, I think we’re running into those things. It’s not just simply moving a container into the cloud. It’s rethinking how it works inside that piece of software. I think that’s the stuff that gets really challenging for people.
Thinking Security with Cloud Native
Reisz: We’ve talked about some different ways of thinking when we talk cloud native. I want to talk a little bit about security. How do you have to think about security when we’re talking about cloud native?
Fong-Jones: I think that security can be a challenge when you introduce new control planes, when you introduce new ways to interact with your workloads. For instance, Ian Coldwater gives these amazing talks about how to break out of that container into another container to take over the Kubernetes control plane. These are things that are new threat models that are introduced when you have all these things that are meant to be flying information. I think that there are things you need to worry about around authorization. You can no longer have this idea that if it’s in my cluster, I trust it. If it can talk to me, I trust it. You have to start adopting these things like gRPC, security certificates. I think that when you want to level up, you have to level up all the practices including your security practices. That being said, you don’t have to use Kubernetes in order to be cloud native. I think that it’s a thing where if you don’t adopt Kubernetes, you can still use some of the more tried and true practices, and you don’t have to keep quite as much on the cutting edge.
Walker: When I think about security and cloud native, all of the core principles that we’ve been following for security for years still apply. It’s still AAA. It’s all this stuff. I think this layer of zero trust, and just this whole movement towards zero trust between everything, thinking about it in that way, is what is allowing people to identify these types of threats, and being able to actually figure out how to contend against them. The threat vectors have gone up exponentially. I think zero trust and that whole movement is wildly interesting.
Reisz: It’s with the supply chain, too.
Breck: I think part of your cloud native scaling strategy is adopting managed services or fundamental platforms from the cloud providers. I think the security story gets a lot better. I trust Microsoft and Amazon to be better at threat modeling, and patching, and all those kinds of things than most organizations. The more you can turn over to them, I think the security story in some ways gets better.
Walker: Do you remember back in the day when it was like, you didn’t want to go to the public cloud provider because you were worried about their security. That’s a serious change.
Breck: I agree. I think that’s completely inverted now.
Cui: I was talking to quite a few customers, because my focus is on serverless. That’s one of the big selling points of serverless to large enterprise companies, is around security. Because when you offload infrastructure security to AWS, or to Google, or to Microsoft, you essentially eliminate a whole massively complex class of security problems around infrastructure, around your operating system, around patching and making sure everything is nice and secured. That is now no longer your problem. You don’t have to worry about the VM security. You don’t have to worry about the security of the virtualization layer, or the operating system, just focusing on the application level security, which is still a lot of things to do. It’s a much smaller attack surface that you have to worry about.
Think of when you’re using things like managed services, you’re also much more shifted towards this mindset of zero trust, because every service you want to talk to, you have to authenticate yourself somehow. I can’t talk to DynamoDB by being asynchronous to DynamoDB. That just doesn’t happen. Everything is based on the same consistent authentication model. You can easily use the same model in your own application as well, which makes security much more interesting and consistent for me. I think that has been one of the big reasons a lot of enterprise customers, especially like banking and the financial institutions, they are moving to serverless because of security.
Cloud Native and Observability
Reisz: How does the observability story change, or does it, when we start talking cloud native?
Fong-Jones: I think that observability becomes more of a requirement as you adopt cloud native technologies, because of that decoupling from hardware, because of the fact that you’re potentially running many more microservices. I think all those complex interactions make it so that you can no longer achieve observability with the previous set of tools that we all used to use, and that you have to think about your ability to examine unknown interactions. Things that you didn’t anticipate were going to happen and things that were complex interactions between services or between your users and your services.
Reisz: How do we deal with that, particularly when we start thinking IoT, with thousands in digital twins? We start talking about just thousands of potential things. How do we really get our mind around doing some of the stuff that Liz just said, with cloud native and observability?
Breck: I don’t think we quite have as an industry. Actually, I wrote an article along these lines of like, at huge scale, we need to get away from that feeling of I want to trace every event through the system, or I want to know what’s happening with every customer. Discrete becomes more continuous at huge scale. How do we take continuous signals and use that to tell what’s going on in our systems. To me that looks like systems engineering or process engineering. You don’t control a distillation column by looking at every molecule, you control it with these global signals around temperature and flow rates, and those kinds of things. We use a lot of those techniques for streaming systems in IoT at scale to tell what’s going on, not looking at discrete events.
Walker: It’s signal and noise here. There’s reasons why Honeycomb and some of the other people that are out there doing this have a business because there’s a lot of information that we can collect. In fact, I love what’s going on with tracing, and I find it extremely interesting. I’m really excited about OpenTelemetry. The OpenTelemetry project in the CNCF is right now the number two most traffic. It’s awesome, because it’s important. It’s not just the tracing side of it. It’s the telemetry coming off of each one of these machines as well. I think, to me, right now, in the cloud native space, one of the most interesting movements that’s going on. Like in the database, we use this all the time. We use tracing, because what is a query when you’re going across multiple different nodes, and you’re doing some join across huge, massive regions? How do we help our customers troubleshoot a query, a statement that was really simple in a single instance of Postgres? Now it’s spread out all over the whole planet. What’s worse is, there is a network lag between this node and that node, why, between this region and this region it’s gone, and maybe a piece of hardware. The telemetry that’s coming off of these things is awesome. It’s hard to find the signal.
Challenges with Monitoring Everything
Fong-Jones: I want to back up a step here, and let’s just talk through. Some people might have the naïve thought, why don’t we just monitor everything? Why don’t you monitor the network lag between all of these things? Why don’t you alert on everything? I think that that’s an important subject that we should also talk about when we talk about this, is like, why can’t we just monitor everything?
Reisz: Why Can’t We? Why can’t we just monitor all the things?
Walker: We could collect the data, it’s just too difficult to identify the issues. We’re right back in the same problem. This has been our problem for a long time, but there’s ways to do it.
Fong-Jones: Yes. I think that that goes to this premise that if you start from the top of your system, where your user traffic is coming in, and you’re able to systematically investigate, ok, it got slow here, then it got slow here, until you find where the problem is. You’re going to have a lot more luck doing that, than if you start from a million things screaming at you, because this one node is unhealthy, and the network lag has temporarily spiked for five seconds between these two data centers. That’s just too noisy. You’re just never going to be able to correlate it. Having the data isn’t the only thing that matters, it also matters to alert on the right signals of user pain, and also to have that ability to trace things all the way through the system. I think that’s what you’re getting at, Jim, when you were like, we can figure out that the issue with this whole query was because of this network issue. You wouldn’t otherwise have alerted on that network issue problem.
Walker: We’re actually looking into the query itself. We actually have telemetry coming out of the execution of the statements. It’s not just the physical and everything we think about network, it’s actually inside the software. This is what I meant earlier when I was talking about the distributed mindset does not extend just to operations and hardware and everything that’s around these cloud native systems. It’s the way you think about the software you’re building itself. I’ve seen that firsthand with the engineers at Cockroach Labs. I always say that, I think the CockroachDB code base is almost a PhD in a distributed system, because some of the problems that they’re challenged with, in terms of the speed of light, in terms of these sorts of things, and observability, it’s just tremendous at this scale. We’re just scratching the surface and trying to figure these things out, I think as an overall community, in my opinion. I think we’re just getting there.
Cui: I think certainly from this thing about that we’re building resilient, self-healing systems as well, that if the network is slow or some node is down, your system should just handle it and recover. There shouldn’t be any manual intervention. Why would you be monitoring those things when all you care about is user experience? If it doesn’t impact you, you probably shouldn’t be waking up in the morning to have a look at it.
OpenTelemetry
Reisz: Jim, you mentioned OpenTelemetry and literally the room perked up. There’s people on here that are not familiar with OpenTelemetry. Give us the two-minute elevator pitch, why should everybody care? What is this? I’ve never heard of it.
Fong-Jones: OpenTelemetry is a vendor neutral standard for instrumenting and sending telemetry out of your code so that you can then analyze it in some tool in order to achieve observability. Some of the formats of data that OpenTelemetry supports include traces and metrics. There are SDKs in almost every popular language. As Jim mentioned, we are the number two project in the CNCF right now, in terms of number of contributors. We’re a collaboration of pretty much a bunch of end users like Shopify, like DoorDash, as well as a number of vendors such as Honeycomb, and Lightstep. Pretty much almost the entire observability community has come together to build this bar, to say, we are building a set of standards that you only need to insert your code once. That way, even if you decide that you want to change vendors, it provides vendor portability, which is really exciting, or lets people grow from Jaeger on to a more sophisticated solution.
Walker: I just wanted to highlight one thing that it is practitioners as well, it is not just the vendors, which is such a key part of that project. DoorDash was the team that pushed us towards it, because they get this problem at scale. It’s a great project, because it is a lot of practitioners.
Challenges with Observability in Serverless
Reisz: Talk about challenges with observability in the serverless space. What are the problems and what are the realities of it today?
Cui: In your mind, there’s a very different execution paradigm compared to when you’re running containers or VMs, in that, you don’t have access to a server, so we used to just install an agent on a box and be done. You can’t do that anymore. In terms of those monitoring agents, people just talk about how much overhead it adds to your machine, because any CPU cycle you spend on collecting telemetry is the CPU cycle you’re not using to process user events. When it comes to things like Lambda functions, you don’t have to worry about it anymore, because the invocation handles one user request at a time. The fact that you also just have more event-driven systems, or architectures all over the place, event files into S3, triggers a Lambda function, picks it up, process the event. Write something to DynamoDB. That triggers a stream event, triggers another Lambda function. There’s a lot more of this distributed system type of problems that when it comes to debugging, you have to figure out. You have to be able to put together a picture, end-to-end, of what actually happens. Why does something bomb at the fourth function in a call chain, it all traces back to the user request that came in the first place to the API, that something has an invalid payload or something. In terms of debugging problems, it gets more interesting. The fact that the execution model is quite different also puts a spin on how we collect telemetry in the first place. A lot of that has been improving and getting better. We now can run like a sidecar similar to a sidecar to containers, in the Lambda function, but it still requires a bit more complexity. It’s an interesting challenge because there’s a different set of constraints you have to work with.
Fong-Jones: The OTel Lambda extension is awesome.
Cui: Yes, it makes life a lot easier. Do you see the fact that now you can actually run after the user function returns?
Fong-Jones: Yes.
Cui: That was so good.
Observability in the Device and IoT Space
Reisz: Any tips, tricks, and thoughts on the device or IoT space, when it comes to observability?
Breck: It’s hard. Even if you have really the best intentions in IoT, you end up with really long tails of things that you maybe have improved, but there’s still four devices out there that are on some old firmware and the tails are just really long. IoT is so diverse in terms of moving things forward. I think it’s actually the hardest problem in IoT is managing assets. It plays into scaling. It plays into observability. It plays into security, and ultimately building a great product. I don’t think there’s a lot of great patterns or tooling for managing millions of assets. I’m sure that Apple and Google have great ways of managing millions of phones, but those types of platforms are not really available to others. I think there’s a huge opportunity there.
The Challenges with Cloud Native and Data
Reisz: When we talk about cloud native and data, what are the challenges that come to mind for you? What are some of the things that you think about?
Walker: Cloud native and data can be really simple if you’re in a single region, or a single AZ. Like, it’s prop up an instance of a database, preferably Cockroach, you’re going to get active-active, all these things. It’s when we start thinking about scale across regions. What is a region but another cloud provider, in my opinion? I think right now people are trying to say, you can do Kubernetes, and you can have it in multiple regions, but what happens with data at that point. For me, it’s a very different lens because I’m in a database company. We believe in federating data above the Kubernetes clusters. I don’t care if it’s three cloud providers, multi-cloud, on-prem, we don’t care. We federate at a different layer, and it’s at the data layer.
The biggest challenge I think we have with scale is thinking about regions as clouds, thinking about regions as wholly owned different environments. When we get to the point where it is truly a serverless world, and you’re running in lots of different environments, what is that going to mean for the database? Colin you touched on this, what’s a stateful versus a stateless application? We start talking about apps, and most developers I know, there’s a database behind a large amount of our applications. It’s a big challenge. Multi-region is a problem, A, because of the speed of light, again. There’s the network hops between areas. Once you have data moving between New York and Sydney, what does that mean for you? What happens when there’s two transactions happening at the same time, one in New York, and one in Sydney, who wins? Understanding Raft and understanding MVCC, these core principles behind these distributed systems, to basically guarantee transactional consistency and these things, they become really important and really difficult. I think speed of light and consistency of data are two huge challenges, when we think about these things.
Stateful and Stateless
Reisz: Colin, in your introduction, you focused on stateful and stateless a bit. Why was that one of the things that made you draw attention to?
Breck: Services that have state are just fundamentally harder to scale. Often, you’re joining data there, and you need to pay attention to how to join, especially in eventually consistent systems, how those joins are working out. It’s in a distributed system, if you want to have multi-region failover, and you’re trying to replicate state across regions, that’s just really difficult. In IoT or OT, you’re ultimately controlling things in the physical world, too, so the decisions you’re making, from that consistency of data, also play into the model. It’s just really hard. I think often in platforms that have a good separation between compute and storage, you end up with some better models. Another problem that comes up in OT and IT all the time is, you can’t take downtime. You need to migrate systems or upgrade systems or add new functionality while you’re still flying the airplane. If you can have a good separation between compute and state, and that offers you some migration patterns or upgrade patterns, that’s also a real advantage.
War Stories
Reisz: We all have some great war stories about scale in cloud native, anybody have anything in particular that comes to mind that you might want to share?
Fong-Jones: The one hilarious one that I wanted to share is the $15,000 Amazon Lambda bill. It turns out that sometimes elastic scaling gets a little bit too elastic and gets out of control.
Cui: I’ve seen a few. The surprise is things like, I’ve got an infinite recursion. I think that happens a lot is that you trigger a Lambda function with file dropping to S3, and then you process the data. You write into the same bucket in a different folder, but then your root is pointing to the same function, so it just keeps running. Then, that can fan out pretty quickly as well. Those problems that I think have hit a few people in the past.
In terms of cloud native war stories, I don’t have one myself. This didn’t come from me. I heard it from Corey Quinn, who was talking about a company that was spending something in the order of hundreds of thousands of dollars a month or something like that on just network, or network bandwidth, because I think they were using a network gateway that you get out of the box from AWS to basically manage the net. That is charged based on number of gigabytes processed, so when you are sending through terabytes of data over the network every day, then that can get really expensive really quickly. I think he managed to cut it down to almost nothing by just using a bunch of net servers that the client runs themselves instead. Those problems pop up regularly, way more than any other problems as CPU run into serverless, just because cost management in the cloud is really complex. Especially, once you factor into all the different layers that factors into it, like data transfer, networking, picking the right instance sizes, and things like that, it just gets really messy really quickly.
Resiliency in Event-Driven Systems
Reisz: There’s a question here specifically about Pub/Sub. When we talk about resiliency in event-driven systems, how do you think about recovery? How do you think about making them resilient? How do you think about just recovery modes, when there’s issues that happen? What comes to mind? What do you think about? What are some quick hits for someone who may be struggling with an event-driven system? How do you start to think about this?
Breck: If I constrain it to event driven, I’ll not think too much about IoT, you do have to have a sense of like, when devices talk to you, or when customers or whatever it is talk to you. Because if it’s purely Pub/Sub or purely event driven, and you haven’t heard from something in five minutes, is it offline? Is it in a reboot loop? Is it broken, or does it only never talk to you every 10 minutes or something like that? You need to have a sense of the data model built in when you’re in a pure Pub/Sub or event-based system.
Walker: I would extend a little bit of that, and just say it’s not active-passive. It’s not replication, one-to-one. It’s collaboration and coordination. If you’re going to actually get anything that’s getting any resilience in a distributed system, it’s about coordination. Don’t think about one-to-one, think about many coordinating and getting consistency. That’s the trick, and that’s in your software itself.
Breck: Especially when it’s interacting with the physical world, just say what you know, don’t try and make things up. Don’t try and infer from data, or a Pub/Sub system, or a join that didn’t quite work, something like that. Don’t infer, just present what you know.
Reisz: There’s a lot of times devices are offline, you have to infer to be able to get a complete picture. Particularly when we’re talking not at a macro level, at a micro level, you’re going to get an incomplete picture. You’re going to get, not the full story. How do you deal with that?
Breck: The example there would be, maybe you haven’t heard from a device in a while. You can say this is the last state that was reported, but it’s also stale. I would have expected to hear from this device a minute ago, so here’s the last state that I know. Don’t draw a line on a graph extrapolating from that. Draw that in some shade of gray that says, here’s what I know, but take it with a grain of salt because it’s not up to date.
Walker: You’re right, but it comes back to your workload and what you want to accomplish. If you can infer in some correct way. It depends on what you’re trying to accomplish. If you have to have consistency, like it’s going to cause a nuclear missile to go up or something, you can’t do that. There’s this range of what you want to accomplish from the value of the data that you actually have to interrogate before you implement the system. Sometimes it can do with a five minute delay, that’s ok. It depends on how mission critical. If it’s a bank account, no, you need quorum. It doesn’t work otherwise. It really comes down to that question.
Key Takeaways on Scaling an Event-Driven System
Reisz: I want to give everybody an opportunity to, your just one more thing, your big takeaway. I want to give everyone an opportunity to give some food for thought for the developers and the leadership that’s in the room on what it means to scale an event-driven system.
Cui: You need to have good practices before you even try to attempt this system, because they are quite complex. There’s no magic bullet that you can just, use this tool and you’ll be good. No, you need to scale up your team. You need to scale up the organization on how you do things. Having a good CI/CD, security models, and everything else around it.
Fong-Jones: Provocative statement, everyone should have the privilege of being able to deploy to production within an hour. It doesn’t matter if you have all the elasticity in the world and all the workload portability, if you don’t have the ability to actually make changes to your code, and to deploy it and get it out there.
Walker: I’ll go old school and give it the framework of people, process, tech. A distributed mindset will go a long way. The best practices around these things are critical to get right. You can go adopt all these cloud native technologies, if you don’t understand what an SRE does, or what that function actually was there for, you’re going to gyrate for a while, and not really get far. I think it’s really important to choose the right technology. Some tech claims to be cloud native. It’s a lift and shift. It’s a move and improve. I think the technologies out there that are interesting right now are those that have been re-architected for this world. This is a fundamentally different world we live in. I’m a startup-aholic, so there’s so many really interesting startups right now, because they are re-architecting for the cloud. I think that’s the stuff that gets really interesting. People, process, tech is always my lens for these things.
Breck: Don’t get too hung up on technologies, and more focused on the fundamental architectural principles. I actually like what Reactive Foundation is doing in that regard, of, it’s technology agnostic but focuses on the principles. It’s really a fundamental shift. Some of the new service platforms or what the cloud providers are doing actually encourage you in a much better direction.
See more presentations with transcripts
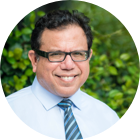
MMS • RSS
Posted on mongodb google news. Visit mongodb google news
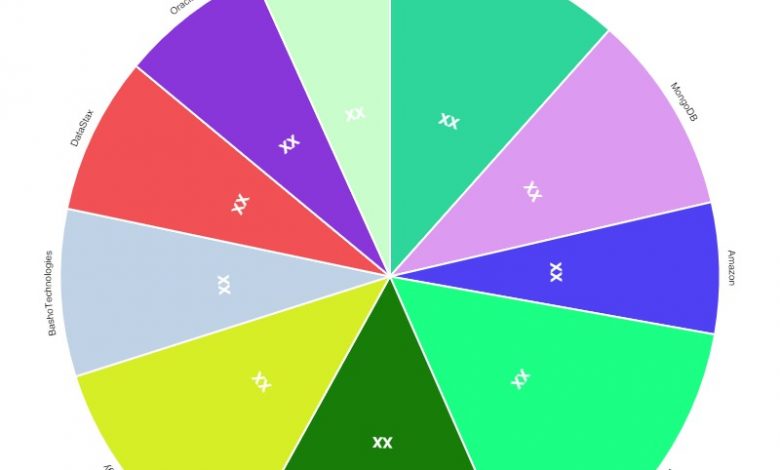
JCMR recently introduced Document Databases study with focused approach on market size & volumes by Application, Industry particular process, product type, players, and production & Consumption analysis considering major factors, cost Structure and regulatory factors. At present, the Document Databases market is developing its presence and some of the key players from the complete study are Couchbase, MongoDB, Amazon, MarkLogic, Aerospike, Neo Technology, Basho Technologies, DataStax, Oracle, MapR Technologies
The report offers a comprehensive evaluation of the Document Databases market. It does so via in-depth Document Databases qualitative insights, Document Databases historical data, and Document Databases verifiable projections about market size. The Document Databases projections featured in the report have been derived using proven research methodologies and assumptions
Our report will be revised to address COVID-19 pre-Post pandemic effects on the Global Document Databases Market.
Click to get Global Document Databases Market Research Free Sample PDF Copy Here Before Purchase @: jcmarketresearch.com/report-details/1114792/sample
Document Databases Market segmentation information from 2013-2029 on The Basis Of follwing coverage:-
[Segments]
This study also contains Document Databases company profiling, Document Databases product picture and specifications, Document Databases sales, Document Databases market share and contact information of various international, regional, and local vendors of Global Document Databases Market, some of them are following key-players Couchbase, MongoDB, Amazon, MarkLogic, Aerospike, Neo Technology, Basho Technologies, DataStax, Oracle, MapR Technologies. The Document Databases market competition is constantly growing higher with the rise in technological innovation and M&A activities in the Document Databases industry. Moreover, many local and regional vendors are offering specific application products for varied end-users. The new vendor entrants in the market are finding it hard to compete with the international Document Databases vendors based on quality, Document Databases reliability, and innovations in Document Databases technology.
Buy Full Copy with Exclusive Discount on Global Document Databases Market @ jcmarketresearch.com/report-details/1114792/discount
Highlights about Document Databases report coverage:
– A complete background analysis, which includes an assessment of the Global Document Databases Market.
– Important changes in Document Databases market dynamics
– Document Databases Market segmentation up to the second & third level regional bifurcation
– Historical, current, and projected size of the Document Databases market with respect to both value (Revenue) and volume (Production & Consumption)
– Reporting and evaluation of recent Document Databases industry developments
– Document Databases Market shares and strategies of key players
– Emerging niche Document Databases segments and regional markets
– An objective assessment of the trajectory of the Document Databases market
– Recommendations to companies for strengthening their foothold in the Document Databases market
Additionally the export and import policies that can make an immediate impact on the Global Document Databases Market. This study contains a EXIM* related chapter on the Global Document Databases Market and all its associated companies with their profiles, which gives valuable data pertaining to their outlook in terms of finances, product portfolios, investment plans, and marketing and business strategies.
Enquire for customization in Global Document Databases Market Report @ jcmarketresearch.com/report-details/1114792/enquiry
There are following 15 Chapters to display the Global Document Databases Market.
Table of Contents
1 Document Databases Market Overview
1.1 Global Document Databases Introduction
1.2 Market Analysis by [Type]
1.3 Market Analysis by [Application]
1.4 Market Analysis by North America, Europe, China, Japan, Rest of the World
1.5 Document Databases Market Dynamics
1.5.1 Document Databases Market Opportunities
1.5.2 Document Databases Market Risk
1.5.3 Document Databases Market Driving Force
2 Document Databases Manufacturers Profiles
2.1.1 Document Databases industry Business Overview
2.1.2 Global Document Databases Market Type and Applications
2.1.3 Document Databases Sales, Price, Revenue, Gross Margin and Market Share and SWOT analysis (2019-2020)
3 Global Document Databases Market Competition, by Manufacturer
4 Global Document Databases Market Analysis by Regions including their countries
5 By Document Databases diffrent Regions
6 Document Databases Product Types
7 Document Databases Application Types
8 Key players- Couchbase, MongoDB, Amazon, MarkLogic, Aerospike, Neo Technology, Basho Technologies, DataStax, Oracle, MapR Technologies
.
.
.
10 Document Databases Segment by Types
11 Document Databases Segment by Application
12 Document Databases COVID-19 Impacted Forecast (2021-2029)
13 Document Databases Sales Channel, Distributors, Traders and Dealers
14 Document Databases Research Findings and Conclusion
15 Appendix
….Continued
Complete report on Global Document Databases Market report spread across 200+ pages, list of tables & figures, profiling 10+ companies. Select license version and Buy this updated Research Report Directly @ jcmarketresearch.com/checkout/1114792
How Are We Different? & Why Choose Us?
We always believe in the quality, so JCMR will provide you instant 24*7 sales support. In case, you have any queries or any doubts then we will provide post purchase priority analyst assistance to clarify even single doubt on the Document Databases study.
If you still have a question, give it a try- [email protected]
Find more research reports on Document Databases Industry. By JC Market Research.
About Author:
JCMR global research and market intelligence consulting organization is uniquely positioned to not only identify growth opportunities but to also empower and inspire you to create visionary growth strategies for futures, enabled by our extraordinary depth and breadth of thought leadership, research, tools, events and experience that assist you for making goals into a reality. Our understanding of the interplay between industry convergence, Mega Trends, technologies and market trends provides our clients with new business models and expansion opportunities. We are focused on identifying the “Accurate Forecast” in every industry we cover so our clients can reap the benefits of being early market entrants and can accomplish their “Goals & Objectives”.
Contact Us: https://jcmarketresearch.com/contact-us
JCMARKETRESEARCH
Mark Baxter (Head of Business Development)
Phone: +1 (925) 478-7203
Email: [email protected]
Connect with us at – LinkedIn
Article originally posted on mongodb google news. Visit mongodb google news
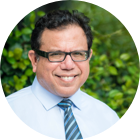
MMS • RSS
Posted on mongodb google news. Visit mongodb google news
MongoDB, Inc. (NASDAQ:MDB) was the target of unusually large options trading activity on Wednesday. Stock traders acquired 36,130 call options on the stock. This represents an increase of 2,077% compared to the typical daily volume of 1,660 call options.
Several equities research analysts have recently commented on MDB shares. Barclays reduced their price target on shares of MongoDB from $590.00 to $556.00 in a report on Wednesday, January 12th. Needham & Company LLC boosted their price objective on shares of MongoDB from $534.00 to $626.00 and gave the stock a “buy” rating in a research note on Tuesday, December 7th. Credit Suisse Group assumed coverage on shares of MongoDB in a research note on Tuesday, November 16th. They set an “outperform” rating and a $700.00 price objective for the company. Morgan Stanley boosted their price objective on shares of MongoDB from $525.00 to $633.00 and gave the stock an “overweight” rating in a research note on Thursday, December 16th. Finally, Citigroup boosted their price objective on shares of MongoDB from $526.00 to $660.00 and gave the stock a “buy” rating in a research note on Tuesday, December 7th. Four investment analysts have rated the stock with a hold rating and twelve have given a buy rating to the company. According to data from MarketBeat.com, the stock currently has an average rating of “Buy” and a consensus target price of $542.13.
In other MongoDB news, CFO Michael Lawrence Gordon sold 10,000 shares of MongoDB stock in a transaction on Thursday, December 9th. The shares were sold at an average price of $532.51, for a total value of $5,325,100.00. The sale was disclosed in a legal filing with the Securities & Exchange Commission, which is available through this hyperlink. Also, CEO Dev Ittycheria sold 45,056 shares of MongoDB stock in a transaction dated Tuesday, January 11th. The stock was sold at an average price of $449.00, for a total transaction of $20,230,144.00. The disclosure for this sale can be found here. Insiders sold 114,506 shares of company stock worth $55,874,990 over the last quarter. Insiders own 7.40% of the company’s stock.
The first platform of its kind for pet owners is about to flip the NFT space on its head.
Several institutional investors and hedge funds have recently modified their holdings of MDB. Price T Rowe Associates Inc. MD raised its stake in shares of MongoDB by 191.2% in the 2nd quarter. Price T Rowe Associates Inc. MD now owns 5,766,896 shares of the company’s stock valued at $2,084,848,000 after acquiring an additional 3,786,467 shares in the last quarter. Moors & Cabot Inc. bought a new stake in shares of MongoDB during the third quarter worth $601,000. Vanguard Group Inc. increased its holdings in MongoDB by 7.9% in the second quarter. Vanguard Group Inc. now owns 5,378,537 shares of the company’s stock valued at $1,944,448,000 after purchasing an additional 391,701 shares during the last quarter. Growth Interface Management LLC acquired a new position in MongoDB in the third quarter valued at about $86,758,000. Finally, Caas Capital Management LP acquired a new position in MongoDB in the second quarter valued at about $65,542,000. 87.90% of the stock is currently owned by institutional investors.
Shares of NASDAQ MDB opened at $351.57 on Thursday. The firm has a market capitalization of $23.47 billion, a P/E ratio of -74.33 and a beta of 0.65. MongoDB has a one year low of $238.01 and a one year high of $590.00. The company’s fifty day moving average is $480.49 and its two-hundred day moving average is $456.96. The company has a debt-to-equity ratio of 1.71, a quick ratio of 4.75 and a current ratio of 4.75.
MongoDB (NASDAQ:MDB) last announced its earnings results on Monday, December 6th. The company reported ($0.11) earnings per share for the quarter, beating the consensus estimate of ($0.38) by $0.27. MongoDB had a negative net margin of 38.32% and a negative return on equity of 101.71%. The firm had revenue of $226.89 million during the quarter, compared to the consensus estimate of $205.18 million. During the same quarter in the previous year, the company posted ($0.98) EPS. The firm’s revenue for the quarter was up 50.5% on a year-over-year basis. On average, sell-side analysts forecast that MongoDB will post -4.56 earnings per share for the current fiscal year.
About MongoDB
MongoDB, Inc engages in the development and provision of a general purpose database platform. The firm’s products include MongoDB Enterprise Advanced, MongoDB Atlas and Community Server. It also offers professional services including consulting and training. The company was founded by Eliot Horowitz, Dwight A.
Read More: Diversification Important in Investing
This instant news alert was generated by narrative science technology and financial data from MarketBeat in order to provide readers with the fastest and most accurate reporting. This story was reviewed by MarketBeat’s editorial team prior to publication. Please send any questions or comments about this story to [email protected]
Should you invest $1,000 in MongoDB right now?
Before you consider MongoDB, you’ll want to hear this.
MarketBeat keeps track of Wall Street’s top-rated and best performing research analysts and the stocks they recommend to their clients on a daily basis. MarketBeat has identified the five stocks that top analysts are quietly whispering to their clients to buy now before the broader market catches on… and MongoDB wasn’t on the list.
While MongoDB currently has a “Buy” rating among analysts, top-rated analysts believe these five stocks are better buys.
Article originally posted on mongodb google news. Visit mongodb google news
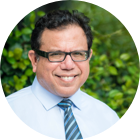
MMS • RSS
Posted on nosqlgooglealerts. Visit nosqlgooglealerts
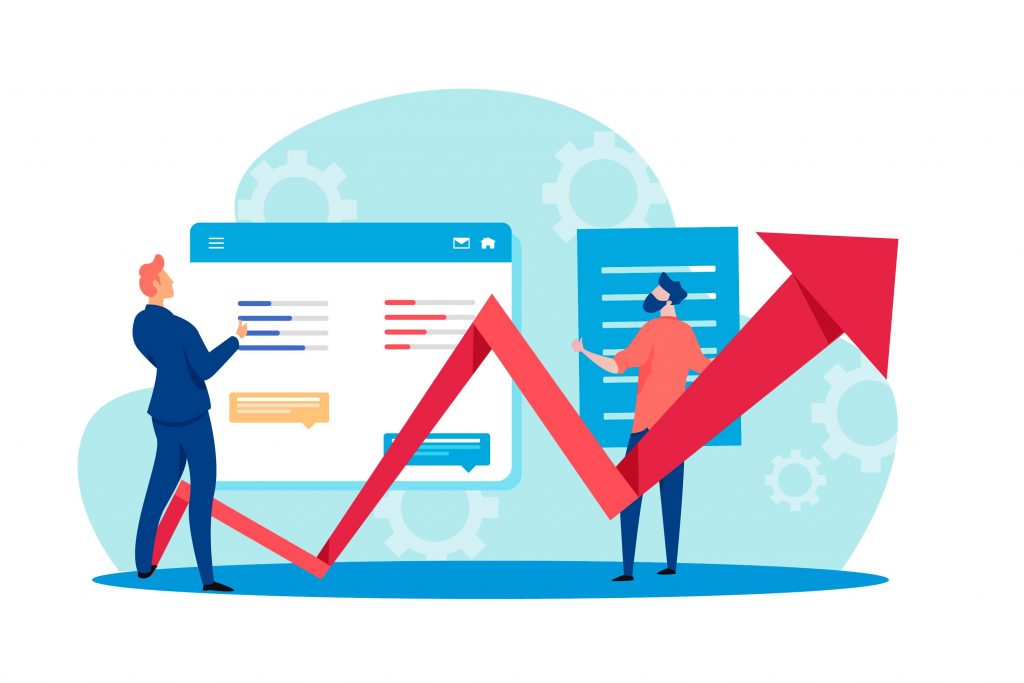
The Global NoSQL market report is a perfect foundation for people looking out for a comprehensive study and analysis of the Global NoSQL market. This report contains a diverse study and information that will help you understand your niche and concentrate of key market channels in the regional and global market for Global NoSQL. To understand competition and take actions based on your key strengths you will be presented with the size of the market, demand in the current and future years, supply chain information, trading concerns, competitive analysis and the prices along with vendor information. The report also has insights about key market players, applications of Global NoSQL, its type, trends and overall market share.
To set your business plan into action based on our detailed report, you will also be provided with complete and accurate prediction along with future projected figures. This will provide a broad picture of the market and help in devising solutions to leverage the key profitable elements and get clarity of the market to make strategic plans. The data present in the report is curated from different publications in our archive along with numerous reputed paid databases. Additionally, the data is collated with the help of dealers, raw material suppliers, and customers to ensure that the final output covers every minute detail regarding the Global NoSQL market, thus making it a perfect tool for serious buyers of this study.
Global NoSQL Market: Competition Landscape
The Global NoSQL market report includes information on the product launches, sustainability, and prospects of leading vendors including: (IBM Cloudant, MongoDB, Aerospike, MapR Technologies, Couchbase, MarkLogic, Basho Technologies, Neo4j, MongoLab, Google, SAP, AranogoDB, Microsoft, Apache, DataStax, RavenDB, Oracle, Amazon Web Services, Redis, CloudDB, MarkLogic)
Click the link to get a free Sample Copy of the Report @ https://crediblemarkets.com/sample-request/global-nosql-market-822354?utm_source=AkshayT&utm_medium=SatPR
Global NoSQL Market: Segmentation
By Types:
Key-Value Store
Document Databases
Column Based Stores
Graph Database
By Applications:
Retail
Online gaming
IT
Social network development
Web applications management
Government
BFSI
Healthcare
Education
Others
Global NoSQL Market: Regional Analysis
All the regional segmentation has been studied based on recent and future trends, and the market is forecasted throughout the prediction period. The countries covered in the regional analysis of the Global Global NoSQL market report are U.S., Canada, and Mexico in North America, Germany, France, U.K., Russia, Italy, Spain, Turkey, Netherlands, Switzerland, Belgium, and Rest of Europe in Europe, Singapore, Malaysia, Australia, Thailand, Indonesia, Philippines, China, Japan, India, South Korea, Rest of Asia-Pacific (APAC) in the Asia-Pacific (APAC), Saudi Arabia, U.A.E, South Africa, Egypt, Israel, Rest of Middle East and Africa (MEA) as a part of Middle East and Africa (MEA), and Argentina, Brazil, and Rest of South America as part of South America.
Key Benefits of the report:
- This study presents the analytical depiction of the global Global NoSQL industry along with the current trends and future estimations to determine the imminent investment pockets.
- The report presents information related to key drivers, restraints, and opportunities along with detailed analysis of the global Global NoSQL market share.
- The current market is quantitatively analyzed from 2021 to 2028 to highlight the global Global NoSQL market growth scenario.
- Porter’s five forces analysis illustrates the potency of buyers & suppliers in the market.
- The report provides a detailed global Global NoSQL market analysis based on competitive intensity and how the competition will take shape in the coming years.
Direct Purchase this Market Research Report Now @ https://crediblemarkets.com/reports/purchase/global-nosql-market-822354?license_type=single_user;utm_source=AkshayT&utm_medium=SatPR
Major Points Covered in TOC:
Market Overview: It incorporates six sections, research scope, significant makers covered, market fragments by type, Global NoSQL market portions by application, study goals, and years considered.
Market Landscape: Here, the opposition in the Worldwide Global NoSQL Market is dissected, by value, income, deals, and piece of the pie by organization, market rate, cutthroat circumstances Landscape, and most recent patterns, consolidation, development, obtaining, and portions of the overall industry of top organizations.
Profiles of Manufacturers: Here, driving players of the worldwide Global NoSQL market are considered dependent on deals region, key items, net edge, income, cost, and creation.
Market Status and Outlook by Region: In this segment, the report examines about net edge, deals, income, creation, portion of the overall industry, CAGR, and market size by locale. Here, the worldwide Global NoSQL Market is profoundly examined based on areas and nations like North America, Europe, China, India, Japan, and the MEA.
Application or End User: This segment of the exploration study shows how extraordinary end-client/application sections add to the worldwide Global NoSQL Market.
Market Forecast: Production Side: In this piece of the report, the creators have zeroed in on creation and creation esteem conjecture, key makers gauge, and creation and creation esteem estimate by type.
Research Findings and Conclusion: This is one of the last segments of the report where the discoveries of the investigators and the finish of the exploration study are given.
Key questions answered in the report:
- What will the market development pace of Global NoSQL market?
- What are the key factors driving the Global Global NoSQL market?
- Who are the key manufacturers in market space?
- What are the market openings, market hazard and market outline of the market?
- What are sales, revenue, and price analysis of top manufacturers of Global NoSQL market?
- Who are the distributors, traders, and dealers of Global NoSQL market?
- What are the Global NoSQL market opportunities and threats faced by the vendors in the Global Global NoSQL industries?
- What are deals, income, and value examination by types and utilizations of the market?
- What are deals, income, and value examination by areas of enterprises?
About US
Credible Markets is a new-age market research company with a firm grip on the pulse of global markets. Credible Markets has emerged as a dependable source for the market research needs of businesses within a quick time span. We have collaborated with leading publishers of market intelligence and the coverage of our reports reserve spans all the key industry verticals and thousands of micro markets. The massive repository allows our clients to pick from recently published reports from a range of publishers that also provide extensive regional and country-wise analysis. Moreover, pre-booked research reports are among our top offerings.
The collection of market intelligence reports is regularly updated to offer visitors ready access to the most recent market insights. We provide round-the-clock support to help you repurpose search parameters and thereby avail a complete range of reserved reports. After all, it is all about helping you reach an informed strategic decision about purchasing the right report that caters to all your market research demands.
Contact Us
Credible Markets Analytics
99 Wall Street 2124 New York, NY 10005
Email: [email protected]
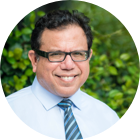
MMS • RSS
Posted on mongodb google news. Visit mongodb google news
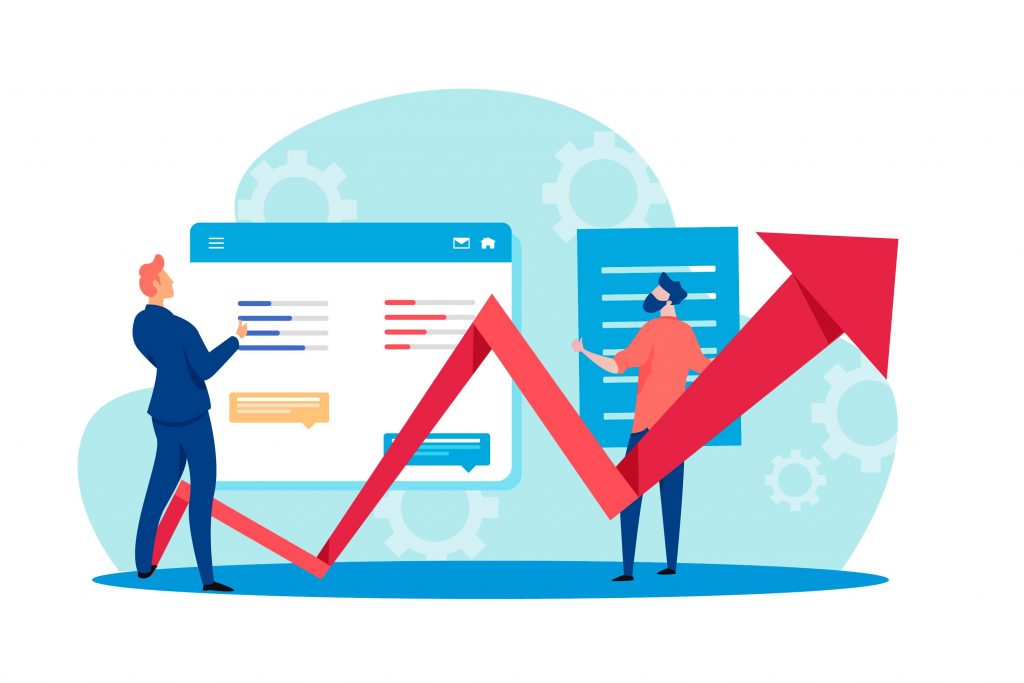
The Global Data Management System (DBMS) Market market report is a perfect foundation for people looking out for a comprehensive study and analysis of the Global Data Management System (DBMS) Market market. This report contains a diverse study and information that will help you understand your niche and concentrate of key market channels in the regional and global market for Global Data Management System (DBMS) Market. To understand competition and take actions based on your key strengths you will be presented with the size of the market, demand in the current and future years, supply chain information, trading concerns, competitive analysis and the prices along with vendor information. The report also has insights about key market players, applications of Global Data Management System (DBMS) Market, its type, trends and overall market share.
To set your business plan into action based on our detailed report, you will also be provided with complete and accurate prediction along with future projected figures. This will provide a broad picture of the market and help in devising solutions to leverage the key profitable elements and get clarity of the market to make strategic plans. The data present in the report is curated from different publications in our archive along with numerous reputed paid databases. Additionally, the data is collated with the help of dealers, raw material suppliers, and customers to ensure that the final output covers every minute detail regarding the Global Data Management System (DBMS) Market market, thus making it a perfect tool for serious buyers of this study.
Global Data Management System (DBMS) Market Market: Competition Landscape
The Global Data Management System (DBMS) Market market report includes information on the product launches, sustainability, and prospects of leading vendors including: (MongoDB, HP, Actian Corporation, Oracle, IWay Software, Couchbase Server, InterSystems, Embarcadero Technologies, BMC Software, Teradata, CA Technologies, IBM, EnterpriseDB Software Solution, Informatica Corporation)
Click the link to get a free Sample Copy of the Report @ https://crediblemarkets.com/sample-request/global-data-management-system-dbms-market-market-871829?utm_source=AkshayT&utm_medium=SatPR
Global Data Management System (DBMS) Market Market: Segmentation
By Types:
Relational
Non-Relational
By Applications:
Banking & Financial
Government
Hospitality
Healthcareand Life Sciences
Education
Media & Entertainment
Professional Service
Telecom & IT
Global Data Management System (DBMS) Market Market: Regional Analysis
All the regional segmentation has been studied based on recent and future trends, and the market is forecasted throughout the prediction period. The countries covered in the regional analysis of the Global Global Data Management System (DBMS) Market market report are U.S., Canada, and Mexico in North America, Germany, France, U.K., Russia, Italy, Spain, Turkey, Netherlands, Switzerland, Belgium, and Rest of Europe in Europe, Singapore, Malaysia, Australia, Thailand, Indonesia, Philippines, China, Japan, India, South Korea, Rest of Asia-Pacific (APAC) in the Asia-Pacific (APAC), Saudi Arabia, U.A.E, South Africa, Egypt, Israel, Rest of Middle East and Africa (MEA) as a part of Middle East and Africa (MEA), and Argentina, Brazil, and Rest of South America as part of South America.
Key Benefits of the report:
- This study presents the analytical depiction of the global Global Data Management System (DBMS) Market industry along with the current trends and future estimations to determine the imminent investment pockets.
- The report presents information related to key drivers, restraints, and opportunities along with detailed analysis of the global Global Data Management System (DBMS) Market market share.
- The current market is quantitatively analyzed from 2021 to 2028 to highlight the global Global Data Management System (DBMS) Market market growth scenario.
- Porter’s five forces analysis illustrates the potency of buyers & suppliers in the market.
- The report provides a detailed global Global Data Management System (DBMS) Market market analysis based on competitive intensity and how the competition will take shape in the coming years.
Direct Purchase this Market Research Report Now @ https://crediblemarkets.com/reports/purchase/global-data-management-system-dbms-market-market-871829?license_type=single_user;utm_source=AkshayT&utm_medium=SatPR
Major Points Covered in TOC:
Market Overview: It incorporates six sections, research scope, significant makers covered, market fragments by type, Global Data Management System (DBMS) Market market portions by application, study goals, and years considered.
Market Landscape: Here, the opposition in the Worldwide Global Data Management System (DBMS) Market Market is dissected, by value, income, deals, and piece of the pie by organization, market rate, cutthroat circumstances Landscape, and most recent patterns, consolidation, development, obtaining, and portions of the overall industry of top organizations.
Profiles of Manufacturers: Here, driving players of the worldwide Global Data Management System (DBMS) Market market are considered dependent on deals region, key items, net edge, income, cost, and creation.
Market Status and Outlook by Region: In this segment, the report examines about net edge, deals, income, creation, portion of the overall industry, CAGR, and market size by locale. Here, the worldwide Global Data Management System (DBMS) Market Market is profoundly examined based on areas and nations like North America, Europe, China, India, Japan, and the MEA.
Application or End User: This segment of the exploration study shows how extraordinary end-client/application sections add to the worldwide Global Data Management System (DBMS) Market Market.
Market Forecast: Production Side: In this piece of the report, the creators have zeroed in on creation and creation esteem conjecture, key makers gauge, and creation and creation esteem estimate by type.
Research Findings and Conclusion: This is one of the last segments of the report where the discoveries of the investigators and the finish of the exploration study are given.
Key questions answered in the report:
- What will the market development pace of Global Data Management System (DBMS) Market market?
- What are the key factors driving the Global Global Data Management System (DBMS) Market market?
- Who are the key manufacturers in market space?
- What are the market openings, market hazard and market outline of the market?
- What are sales, revenue, and price analysis of top manufacturers of Global Data Management System (DBMS) Market market?
- Who are the distributors, traders, and dealers of Global Data Management System (DBMS) Market market?
- What are the Global Data Management System (DBMS) Market market opportunities and threats faced by the vendors in the Global Global Data Management System (DBMS) Market industries?
- What are deals, income, and value examination by types and utilizations of the market?
- What are deals, income, and value examination by areas of enterprises?
About US
Credible Markets is a new-age market research company with a firm grip on the pulse of global markets. Credible Markets has emerged as a dependable source for the market research needs of businesses within a quick time span. We have collaborated with leading publishers of market intelligence and the coverage of our reports reserve spans all the key industry verticals and thousands of micro markets. The massive repository allows our clients to pick from recently published reports from a range of publishers that also provide extensive regional and country-wise analysis. Moreover, pre-booked research reports are among our top offerings.
The collection of market intelligence reports is regularly updated to offer visitors ready access to the most recent market insights. We provide round-the-clock support to help you repurpose search parameters and thereby avail a complete range of reserved reports. After all, it is all about helping you reach an informed strategic decision about purchasing the right report that caters to all your market research demands.
Contact Us
Credible Markets Analytics
99 Wall Street 2124 New York, NY 10005
Email: [email protected]
Article originally posted on mongodb google news. Visit mongodb google news