Month: October 2023
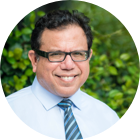
MMS • Sergio De Simone
Article originally posted on InfoQ. Visit InfoQ
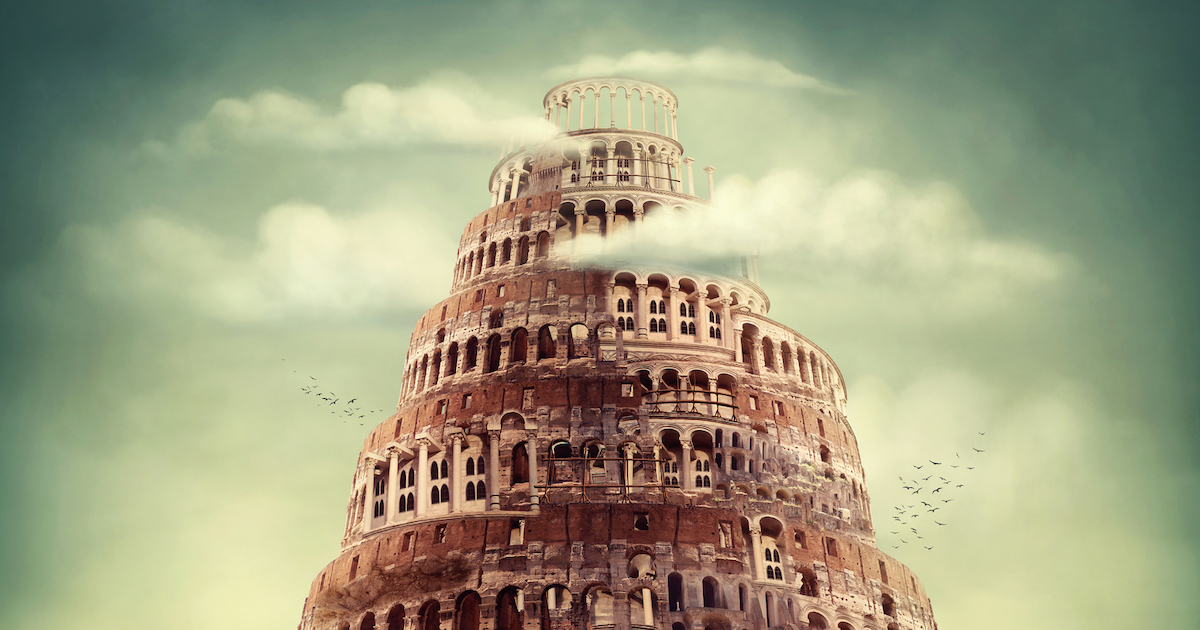
Announced a few months ago, MediaPipe diffusion plugin is now available as an experimental tool on Android devices. Named Image Generator, the plugin can generate images entirely on-device in approximately 15 seconds on high end devices, says Google.
The new MediaPipe Image Generator can be used to generate images based on textual prompts using standard diffusion models. The Image Generation API supports any models conforming to the Stable Diffusion v1.5 architecture.
In addition to using a pre-trained model, you can fine tune your models and convert them to a supported model format using a conversion script provided by Google. This makes it possible to inject conditioning images into your models to better control the generation process and the final generated image. Additionally, you can use Low-Rank Adaptation (LoRA) weights to create images of specific, pre-defined concepts.
To use a diffusion model directly by feeding a textual prompt into the Image Generator API, the first step is creating an options
object with the path to your foundation model files on the device, then pass it to the ImageGenerator
constructor. Once you have the ImageGenerator
instance, you pass it the prompt, the number of iterations, and a seed value, and get back the generated image:
val options = ImageGeneratorOptions.builder().setImageGeneratorModelDirectory(MODEL_PATH).build() imageGenerator = ImageGenerator.createFromOptions(context, options)
val result = imageGenerator.generate(prompt_string, iterations, seed) val bitmap = BitmapExtractor.extract(result?.generatedImage())
Alternatively, you can use a new plugin system that Google developed to make the process of passing a conditioning image easier.
We currently support three different ways that you can provide a foundation for your generations: facial structures, edge detection, and depth awareness. The plugins give you the ability to provide an image, extract specific structures from it, and then create new images using those structures.
Google has provided a number of plugins that should be used in combination with a foundational model, each tailored to a specific end effect. In particular, the Canny Edge plugin uses the edges implied by the condition image, and generates a new image based on the text prompt; the Face Landmark plugin provides a detailed face mesh of a single face and generates a new face over the mesh; finally, the Depth plugin uses the condition image to infer the size and depth of the object to generate. Each plugin supports a number of options to customize their behavior.
The Image Generator can also be customized using LoRA to extend a foundation model by teaching it a new concept.
With the new LoRA weights, the Image Generator becomes a specialized generator that is able to inject specific concepts into generated images.
For example, you can create LoRA weights using several images of a given subject, then use those weights to generate a new image of the same subject in a different environment.
If you are interested in trying the new MediaPipe Image Generator, you can start from the official sample on GitHub, which demonstrates the three ways you can use it.
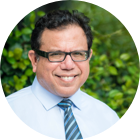
MMS • RSS
Posted on mongodb google news. Visit mongodb google news
LPL Financial LLC boosted its stake in shares of MongoDB, Inc. (NASDAQ:MDB – Free Report) by 13.4% in the second quarter, according to its most recent disclosure with the SEC. The firm owned 25,816 shares of the company’s stock after acquiring an additional 3,059 shares during the period. LPL Financial LLC’s holdings in MongoDB were worth $10,610,000 at the end of the most recent quarter.
Several other hedge funds and other institutional investors have also added to or reduced their stakes in the stock. GPS Wealth Strategies Group LLC acquired a new stake in shares of MongoDB in the second quarter worth approximately $26,000. Capital Advisors Ltd. LLC boosted its stake in MongoDB by 131.0% during the 2nd quarter. Capital Advisors Ltd. LLC now owns 67 shares of the company’s stock worth $28,000 after purchasing an additional 38 shares during the last quarter. Bessemer Group Inc. acquired a new stake in MongoDB during the 4th quarter worth $29,000. Global Retirement Partners LLC boosted its stake in MongoDB by 346.7% during the 1st quarter. Global Retirement Partners LLC now owns 134 shares of the company’s stock worth $30,000 after purchasing an additional 104 shares during the last quarter. Finally, Manchester Capital Management LLC acquired a new position in MongoDB during the first quarter worth about $36,000. 88.89% of the stock is owned by hedge funds and other institutional investors.
Analysts Set New Price Targets
MDB has been the topic of a number of recent research reports. Bank of America initiated coverage on shares of MongoDB in a research report on Thursday, October 12th. They set a “buy” rating and a $450.00 price target on the stock. Barclays boosted their price objective on MongoDB from $421.00 to $450.00 and gave the stock an “overweight” rating in a research note on Friday, September 1st. Morgan Stanley boosted their price objective on MongoDB from $440.00 to $480.00 and gave the stock an “overweight” rating in a research note on Friday, September 1st. Macquarie boosted their price target on shares of MongoDB from $434.00 to $456.00 in a research report on Friday, September 1st. Finally, JMP Securities boosted their price target on shares of MongoDB from $425.00 to $440.00 and gave the company a “market outperform” rating in a research report on Friday, September 1st. One investment analyst has rated the stock with a sell rating, three have given a hold rating and twenty-two have assigned a buy rating to the stock. Based on data from MarketBeat.com, MongoDB has an average rating of “Moderate Buy” and an average target price of $415.46.
View Our Latest Stock Analysis on MongoDB
Insider Buying and Selling at MongoDB
In other news, CAO Thomas Bull sold 518 shares of the firm’s stock in a transaction dated Monday, October 2nd. The stock was sold at an average price of $342.41, for a total transaction of $177,368.38. Following the sale, the chief accounting officer now owns 16,672 shares of the company’s stock, valued at approximately $5,708,659.52. The sale was disclosed in a filing with the Securities & Exchange Commission, which is available through this hyperlink. In other MongoDB news, CAO Thomas Bull sold 518 shares of the firm’s stock in a transaction that occurred on Monday, October 2nd. The shares were sold at an average price of $342.41, for a total transaction of $177,368.38. Following the sale, the chief accounting officer now owns 16,672 shares of the company’s stock, valued at $5,708,659.52. The transaction was disclosed in a filing with the SEC, which can be accessed through the SEC website. Also, Director Dwight A. Merriman sold 1,000 shares of the business’s stock in a transaction on Friday, September 1st. The shares were sold at an average price of $395.01, for a total transaction of $395,010.00. Following the completion of the sale, the director now directly owns 535,896 shares of the company’s stock, valued at $211,684,278.96. The disclosure for this sale can be found here. Insiders have sold a total of 187,984 shares of company stock worth $63,945,297 over the last ninety days. Corporate insiders own 4.80% of the company’s stock.
MongoDB Stock Up 2.4 %
Shares of NASDAQ:MDB opened at $335.31 on Monday. The firm’s 50 day moving average price is $357.51 and its 200 day moving average price is $344.49. The stock has a market cap of $23.92 billion, a price-to-earnings ratio of -96.91 and a beta of 1.13. MongoDB, Inc. has a fifty-two week low of $135.15 and a fifty-two week high of $439.00. The company has a quick ratio of 4.48, a current ratio of 4.48 and a debt-to-equity ratio of 1.29.
MongoDB (NASDAQ:MDB – Get Free Report) last announced its quarterly earnings data on Thursday, August 31st. The company reported ($0.63) earnings per share for the quarter, beating analysts’ consensus estimates of ($0.70) by $0.07. MongoDB had a negative net margin of 16.21% and a negative return on equity of 29.69%. The business had revenue of $423.79 million for the quarter, compared to analyst estimates of $389.93 million. As a group, equities research analysts predict that MongoDB, Inc. will post -2.17 earnings per share for the current fiscal year.
About MongoDB
MongoDB, Inc provides general purpose database platform worldwide. The company offers MongoDB Atlas, a hosted multi-cloud database-as-a-service solution; MongoDB Enterprise Advanced, a commercial database server for enterprise customers to run in the cloud, on-premise, or in a hybrid environment; and Community Server, a free-to-download version of its database, which includes the functionality that developers need to get started with MongoDB.
Featured Articles
Receive News & Ratings for MongoDB Daily – Enter your email address below to receive a concise daily summary of the latest news and analysts’ ratings for MongoDB and related companies with MarketBeat.com’s FREE daily email newsletter.
Article originally posted on mongodb google news. Visit mongodb google news
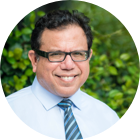
MMS • Michael Redlich
Article originally posted on InfoQ. Visit InfoQ
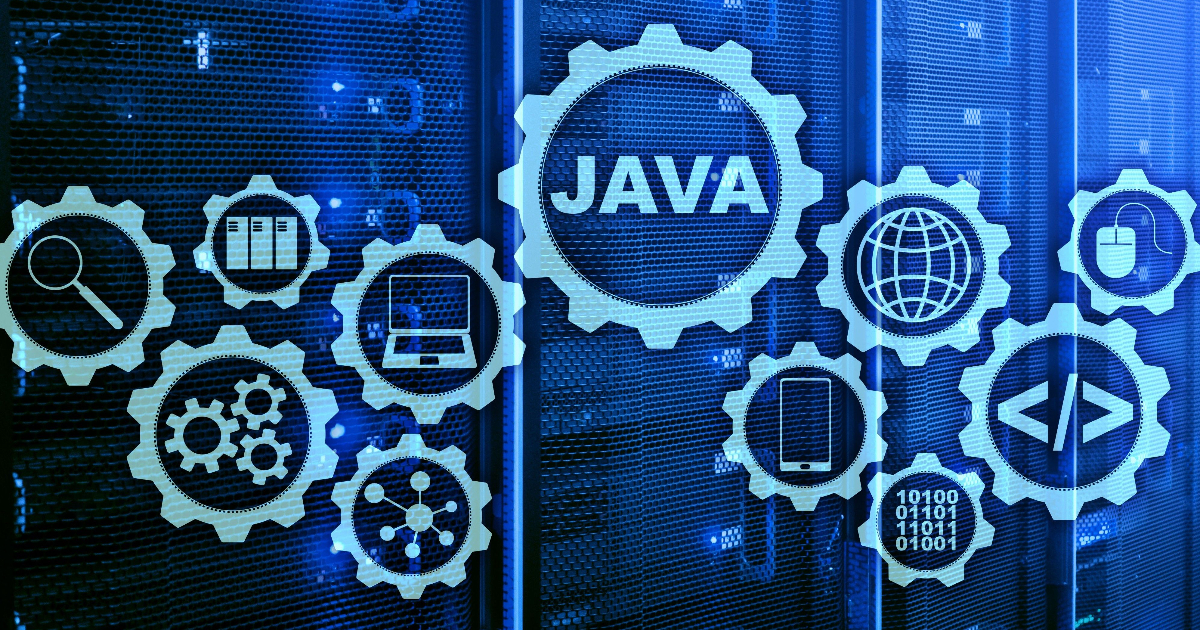
This week’s Java roundup for October 23rd, 2023 features news from OpenJDK, JDK 22, Jakarta Data 1.0-M1, GraalVM 21.0.1, Spring 6.1-RC2, Spring Modulith 1.1-RC1, Spring Vault 3.1-RC1, Helidon 4.0, Eclipse Serializer 1.0, Quarkus 3.5, Liberica NIK 22.3.4, Hibernate ORM 6.4-CR1, Hibernate Search 7.0-CR1, Maven 4.0.0-alpha8, Camel 4.0.2, Camel Quarkus 3.5, JHipster Lite 0.46 and JDKMonitor.
OpenJDK
After its review has concluded, JEP 456, Unnamed Variables & Patterns, has been promoted from Proposed to Target to Targeted for JDK 22. This JEP proposes to finalize this feature after one previous round of preview: JEP 443, Unnamed Patterns and Variables (Preview), delivered in JDK 21. This feature will “enhance the language with unnamed patterns, which match a record component without stating the component’s name or type, and unnamed variables, which can be initialized but not used.” Both of these are denoted by the underscore character as in r instanceof _(int x, int y)
and r instanceof _
.
JEP 460, Vector API (Seventh Incubator), has been promoted from Candidate to Proposed to Target for JDK 22. This JEP, under the auspices of Project Panama, incorporates enhancements in response to feedback from the previous six rounds of incubation: JEP 448, Vector API (Sixth Incubator), to be delivered in the upcoming GA release of JDK 21; JEP 438, Vector API (Fifth Incubator), delivered in JDK 20; JEP 426, Vector API (Fourth Incubator), delivered in JDK 19; JEP 417, Vector API (Third Incubator), delivered in JDK 18; JEP 414, Vector API (Second Incubator), delivered in JDK 17; and JEP 338, Vector API (Incubator), delivered as an incubator module in JDK 16. The most significant change from JEP 448 includes an enhancement to the JVM Compiler Interface (JVMCI) to support Vector API values. The review is expected to conclude on November 3, 2023.
JEP 462, Structured Concurrency (Second Preview), has been promoted from its JEP Draft 8317302 to Candidate status. This JEP will propose to re-preview the API in JDK 22, without change, in order to gain more feedback from the previous round of preview: JEP 453, Structured Concurrency (Preview), delivered in JDK 21. This feature simplifies concurrent programming by introducing structured concurrency to “treat groups of related tasks running in different threads as a single unit of work, thereby streamlining error handling and cancellation, improving reliability, and enhancing observability.”
JEP 461, Stream Gatherers (Preview), has been promoted from its JEP Draft 8317955 to Candidate status. This JEP proposes to enhance the Stream API to support custom intermediate operations. “This will allow stream pipelines to transform data in ways that are not easily achievable with the existing built-in intermediate operations.” More details on this JEP may be found in the original design document written by Viktor Klang, Software Architect, Java Platform Group at Oracle.
JDK 22
Build 21 of the JDK 22 early-access builds was made available this past week featuring updates from Build 20 that include fixes to various issues. Further details on this build may be found in the release notes.
For JDK 22, developers are encouraged to report bugs via the Java Bug Database.
Jakarta Data
The first milestone release of Jakarta Data 1.0.0 provides: a new BasicRepository
interface for performing basic operations on entities; new insert()
and update()
methods in CrudRepository
interface to extend the capabilities of basic operations on entities, including insert and update operations; and new annotations, Insert
, Update
, Delete
and Save
, for CRUD operations. More details on this release may be found in the release notes.
GraalVM
Oracle Labs has released GraalVM for JDK 21 Community 21.0.1 featuring fixes based on the Oracle Critical Patch Update for October 2023 These include: a new CEntryPointErrors
class to return errors if the stack boundaries cannot be determined; the process crashing when uncommitting unused memory; and an occasional crash using the ProcessBuilder
class on macOS. Further details on this release may be found in the release notes.
Spring Framework
The second release candidate of Spring Framework 6.1 delivers bug fixes, improvements in documentation, dependency upgrades and new features such as: support for ContextLoader
configuration in the @SpringJUnitConfig
and @SpringJUnitWebConfig
annotations for compatibility with the @ContextConfiguration
annotation; improve the extensibility of the ControlFlowPointcut
class to support pattern matching in method names; and a new annotation, DisabledInAotMode
, in the TestContext
interface to skip AOT processing. More details on this release may be found in the release notes.
The first release candidate of Spring Modulith 1.1.0 and service release 1.0.2 ship with bug fixes, dependency upgrades and improvements such as: drop support of the memoize()
method in the Google Suppliers
class in favor of the of()
method in the Spring SingletonSupplier
class; and add a mission statement to reference documentation. New features in version 1.1.0-RC1 include: create a corresponding @ApplicationModuleListener
annotation to be defined in the org.springframework.modulith.events
package from its original org.springframework.modulith
package and mark the original annotation as deprecated; and a refactor of the @Modulith
annotation to define a Spring Boot application that follows the Modulith structuring conventions. Further details on this release may be found in the release notes for version 1.1.0-RC1 and version 1.0.2.
The first release candidate of Spring Vault 3.1.0 features improvements in documentation, dependency upgrades and new features: support for role_name
and entity_alias
token parameters in the VaultTokenRequest
class; support for the prehashed
property for the Transit Secrets Engine API; add rewrap()
methods to the VaultTransitOperations
interface and VaultTransitTemplate
class for improved rewrap of the provided batch of cipher text using the latest version of the named key. More details on this release may be found in the release notes.
Helidon
Just over one year since Helidon 4.0.0-ALPHA1 was introduced to the Java community, Oracle has released version 4.0.0 of Helidon featuring the new Helidon Níma server, support for MicroProfile 6.0; and a shift from asynchronous to blocking APIs. The Helidon Níma server was designed and built from the ground up to fully harness the capabilities of virtual threads, one of the final features of JDK 21. Further details on this release may be found in the release notes and InfoQ will follow up with a more detailed news story.
Service releases 3.2.3 and 2.6.4 of Helidon both ship with notable changes such as: the tarketKeys
variable defined in the HttpSignProvider
class now returns an instance of the Java ConcurrentHashMap
class over the previously used HashMap
class; the max-payload-size
property is now parsed as of type Long
to align with Helidon 4.0 and to eliminate the IllegalArgumentException
when the value is greater than Integer.MAX_VALUE
; and add zero-argument non-private constructors to the NonTransactionalEntityManager
and ExtendedEntityManager
classes. More details on these releases may be found in the changelogs for version 3.2.3 and version 2.6.4.
Eclipse Serializer
The Eclipse Foundation has released version 1.0 of Eclipse Serializer, a project designed to handle any Java object, with complex object structure, and highly secure. Formerly known as MicroStream Serializer, this project enables developers to serialize any Java object, but unlike traditional Java serialization, there is no need to implement the Serializeable
interface, and no specific interfaces, superclasses or annotations are required.
Quarkus
Red Hat has released version 3.5.0 of Quarkus 3.5.0 featuring bug fixes, improvements in documentation and performance, and notable changes such as: support for JDK 21; enhancements in OIDC token propagation filters to customize the exchange status and provide the client name; and allow the parallel execution of blocking health checks. Further details on this release may be found in the changelog.
BellSoft
BellSoft has released versions 22.3.4, 23.0.2, 23.1.1 for JDK 11.0.10, 17.0.9, and 21.0.1 of their Liberica Native Image Kit builds as part of aforementioned Critical Patch Update release cycle to address: CVE-2023-22025, a vulnerability that allows an unauthenticated attacker, with network access via multiple protocols, to compromise Oracle Java SE, Oracle GraalVM Enterprise Edition and Oracle GraalVM for JDK resulting in unauthorized update, insert or delete access to accessible data; CVE-2023-22067, a vulnerability that allows an unauthenticated attacker, with network access via CORBA, to compromise Oracle Java SE resulting in unauthorized update, insert or delete access to some of Oracle Java SE accessible data; and CVE-2023-22081, a vulnerability that allows unauthenticated attacker, with network access via HTTPS, to compromise Oracle Java SE and Oracle GraalVM for JDK resulting in an unauthorized ability to cause a partial denial of service of Oracle Java SE, Oracle GraalVM for JDK.
Hibernate
The first release candidate of Hibernate ORM 6.4.0 delivers: a new @SoftDelete
annotation to support soft deletes, values as deleted/non-deleted versus active/inactive (reversed); implementation of the remaining functions for handling arrays in HQL and Criteria queries; and support for writing Hibernate-specific events in the JDK Flight Recorder.
The first release candidate of Hibernate Search 7.0.0 features: bug fixes; compatibility with the Hibernate ORM discriminator-based multi-tenancy, Elasticsearch 8.10 and OpenSearch 2.10/2.11; dependency upgrades to Hibernate ORM 6.3.1.Final and Apache Lucene 9.8; and rename of some Maven artifact related to JSR-352, Batch Applications for the Java Platform, to reflect the move to the Jakarta Batch specification.
Apache Software Foundation
The eighth alpha release of Apache Maven 4.0.0 provides notable changes such as: drop support for Plexus XML in favor of StAX/Woodstox for XML parsing; a new 4.1.0 POM model for future releases of Maven; and attach the build POM with a build
classifier to simplify the build/consumer implementation. More details on this release may be found in the release notes.
The release of Apache Camel 4.0.2 ships with bug fixes, dependency upgrades and new features/improvements such as: introduce the ability to use the old Micrometer meter names or follow the new Micrometer naming conventions; support for subfolders in the Dev console for uploading; and an optimization of the matchEndpoint()
method defined in the EndpointHelper
class to avoid regular expressions for endpoints. Further details on this release may be found in the release notes.
To maintain alignment with Quarkus, Camel Quarkus 3.5.0 has been released with notable resolutions to issues such as: a failure in the CamelOracleJdbcTest
class due to timezone information not having been initialized; the Kafka container fails to start when configured for SSL; and an UnsupportedOperationException
with the FastCamelContext
class. More details on this release may be found in the release notes.
JHipster
Version 0.46.0 of JHipster Lite has been released featuring improvements in documentation, dependency upgrades and enhancements: use more universal shebang for scripts to fix compatibility with NixOS; and add interactions, such as hover and selection, to match the current state in the Landscape MiniMap. Further details on this release may be found in the release notes.
JDKMonitor
At Devoxx Morocco, Gerrit Grunwald, Principal Engineer at Azul, introduced a new macOS widget for the desktop that displays the number of days until the next release/update of OpenJDK. The widget also includes functionality to display the latest version of the last four long-term support releases with the ability to download them either as a JDK or JRE with an option to bundle with JavaFX. This widget requires macOS Sonoma and can be downloaded from the App Store.
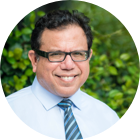
MMS • RSS
Posted on nosqlgooglealerts. Visit nosqlgooglealerts
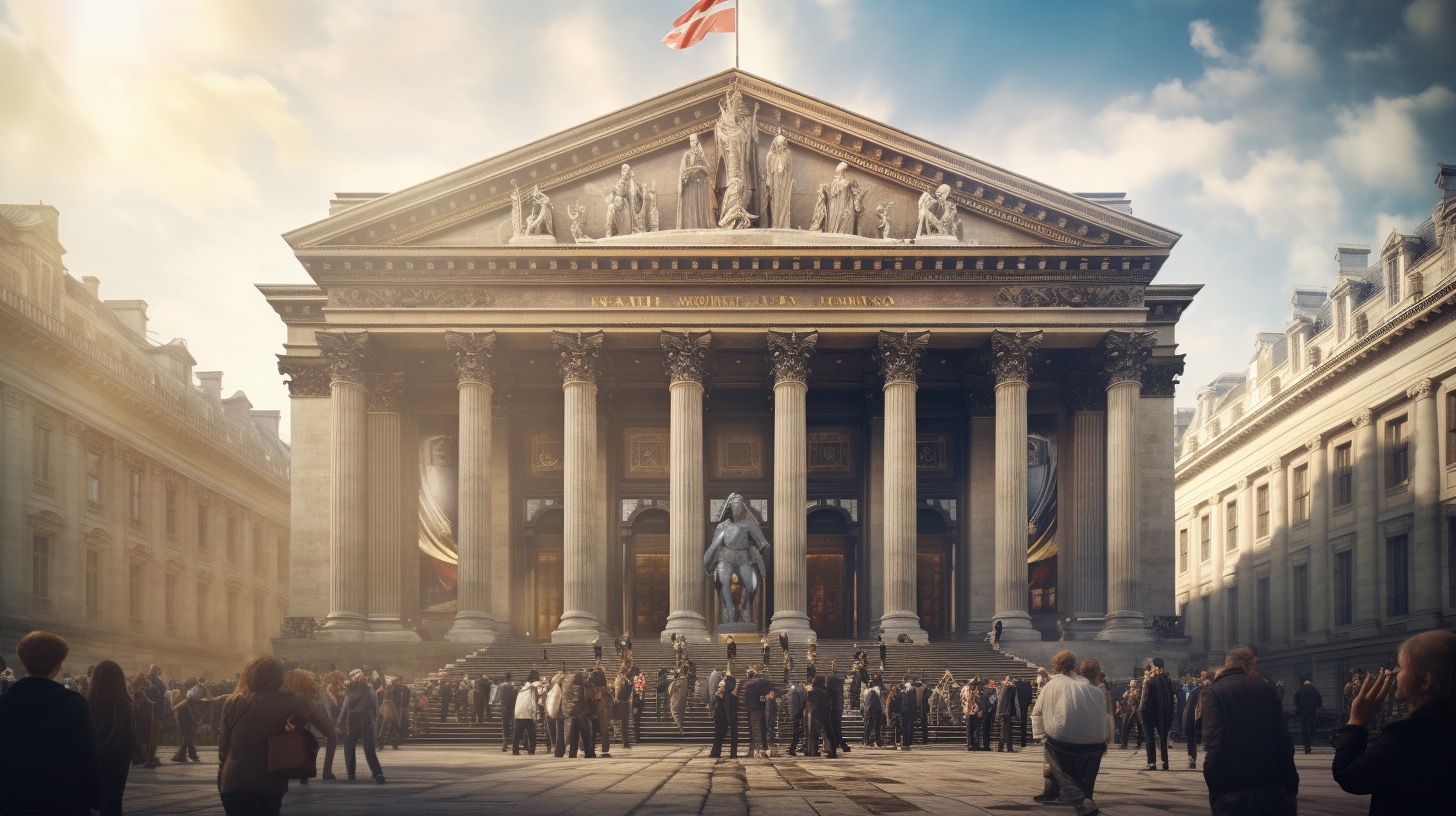
The Evolution of Data Management with Couchbase Inc
Couchbase Inc. has revolutionized the world of data management, transforming the way businesses handle and process their information. With its innovative technology and cutting-edge solutions, Couchbase has become a leading player in the industry, providing organizations with the tools they need to thrive in the digital age.
In order to understand the impact of Couchbase Inc. on modern data management, it is important to trace the evolution of data management itself. In the early days, data was primarily stored in traditional relational databases, which were rigid and inflexible. These databases were designed for structured data and struggled to handle the increasing volume, variety, and velocity of data being generated.
As businesses began to generate more and more data, the limitations of traditional databases became apparent. This led to the rise of NoSQL databases, which offered a more flexible and scalable solution. Couchbase Inc. was at the forefront of this movement, developing a NoSQL database that combined the best features of both relational and non-relational databases.
Couchbase’s flagship product, Couchbase Server, is a distributed NoSQL database that provides high performance, scalability, and availability. It is designed to handle large amounts of data and support a wide range of use cases, from real-time analytics to content management systems. With its flexible data model and powerful querying capabilities, Couchbase Server has become a go-to choice for businesses looking to modernize their data management infrastructure.
One of the key advantages of Couchbase Server is its ability to handle unstructured and semi-structured data. Traditional databases struggle with this type of data, as they require a predefined schema. Couchbase Server, on the other hand, can store and process data in its native format, allowing businesses to capture and analyze a wide variety of data types.
Another area where Couchbase Inc. has made a significant impact is in the realm of real-time analytics. With the rise of big data and the need for instant insights, businesses require a database that can handle the high velocity of data ingestion and provide real-time analytics. Couchbase Server excels in this area, offering low-latency data access and powerful indexing capabilities.
In addition to its core database product, Couchbase Inc. also offers a range of complementary tools and services. These include Couchbase Mobile, which enables businesses to extend their data management capabilities to mobile devices, and Couchbase Cloud, a fully managed database-as-a-service offering. These tools further enhance the flexibility and scalability of Couchbase’s data management solutions, allowing businesses to adapt to changing needs and requirements.
Overall, the impact of Couchbase Inc. on modern data management cannot be overstated. Its innovative technology and comprehensive solutions have transformed the way businesses handle and process their data. With its ability to handle unstructured and semi-structured data, support real-time analytics, and provide flexible deployment options, Couchbase has become a trusted partner for organizations looking to unlock the full potential of their data. As the digital landscape continues to evolve, Couchbase Inc. is well-positioned to lead the way in shaping the future of data management.
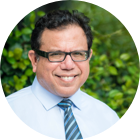
MMS • RSS
Posted on nosqlgooglealerts. Visit nosqlgooglealerts
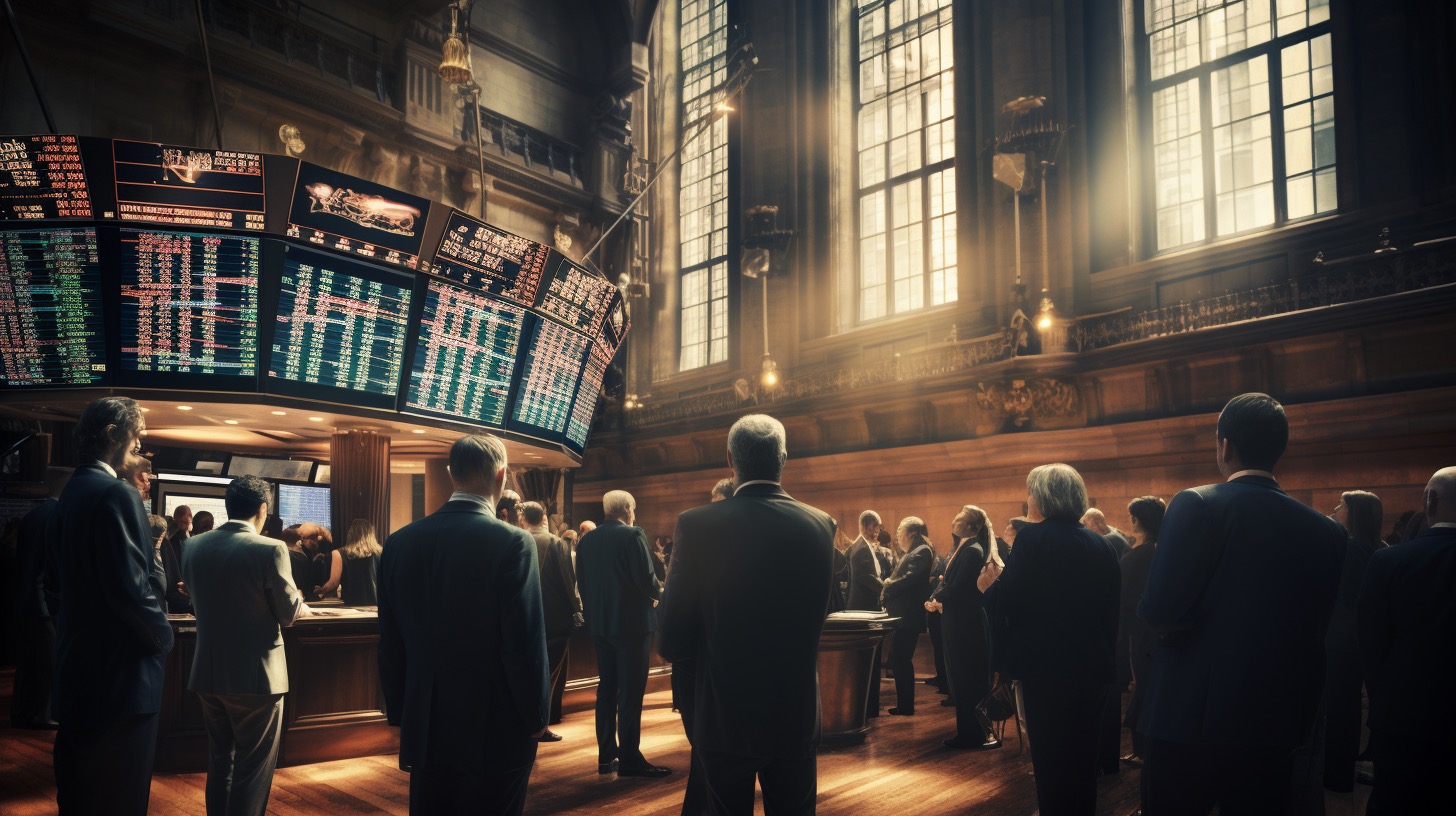
Unveiling the Success Story of Couchbase Inc.: A Leader in Database Innovation
Couchbase Inc. has emerged as a prominent player in the world of database technology, revolutionizing the way organizations manage and store their data. With a rich history and a relentless drive for innovation, Couchbase has become a trusted name in the industry.
The story of Couchbase begins in 2009 when it was founded as a merger between two companies, CouchOne and Membase. The goal was to create a powerful, scalable, and flexible NoSQL database solution that could meet the demands of modern applications. This merger laid the foundation for what would become Couchbase Server, the flagship product of the company.
Couchbase Server quickly gained recognition for its ability to handle large amounts of data with high performance and low latency. Its flexible data model allowed developers to easily adapt to changing business requirements, making it a popular choice among enterprises. As the demand for NoSQL databases grew, Couchbase Inc. continued to innovate and expand its product offerings.
In 2014, Couchbase introduced Couchbase Mobile, a groundbreaking solution that brought the power of NoSQL databases to mobile devices. This allowed developers to build offline-first applications that could seamlessly sync data between the device and the server. Couchbase Mobile became a game-changer in the mobile app development space, enabling organizations to deliver exceptional user experiences even in low or no connectivity environments.
The success of Couchbase Mobile further solidified Couchbase Inc.’s position as a leader in database innovation. The company continued to invest in research and development, pushing the boundaries of what was possible with NoSQL technology. This led to the introduction of Couchbase Analytics, a powerful analytics engine that enabled real-time analysis of data stored in Couchbase Server.
Couchbase Inc. also recognized the importance of cloud computing and embraced it wholeheartedly. In 2016, the company launched Couchbase Cloud, a fully managed database-as-a-service platform that allowed organizations to deploy and scale Couchbase Server in the cloud with ease. This move was well-received by the market, as it provided a hassle-free way for businesses to leverage the power of Couchbase without the burden of managing infrastructure.
As Couchbase Inc. continued to grow, it attracted the attention of investors. In 2020, the company secured a significant funding round led by venture capital firms, further validating its position as a leader in the database industry. This influx of capital allowed Couchbase Inc. to accelerate its product development efforts and expand its global presence.
Today, Couchbase Inc. serves a diverse range of customers, including Fortune 500 companies, government agencies, and startups. Its innovative database solutions have been adopted by organizations across various industries, including finance, healthcare, e-commerce, and gaming. The company’s commitment to customer success and continuous innovation has earned it a loyal customer base and numerous industry accolades.
Looking ahead, Couchbase Inc. shows no signs of slowing down. With a strong focus on cloud-native architectures, machine learning, and artificial intelligence, the company is poised to shape the future of data management. As organizations increasingly rely on data to drive their business strategies, Couchbase Inc. remains at the forefront of providing cutting-edge solutions that empower businesses to unlock the full potential of their data.
In conclusion, the success story of Couchbase Inc. is a testament to the power of innovation and the ability to adapt to changing market demands. From its humble beginnings as a merger between two companies to becoming a leader in database technology, Couchbase Inc. has consistently pushed the boundaries of what is possible. With a strong foundation, a commitment to customer success, and a relentless drive for innovation, Couchbase Inc. is poised to continue shaping the future of data management.
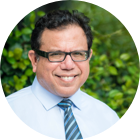
MMS • RSS
Posted on nosqlgooglealerts. Visit nosqlgooglealerts
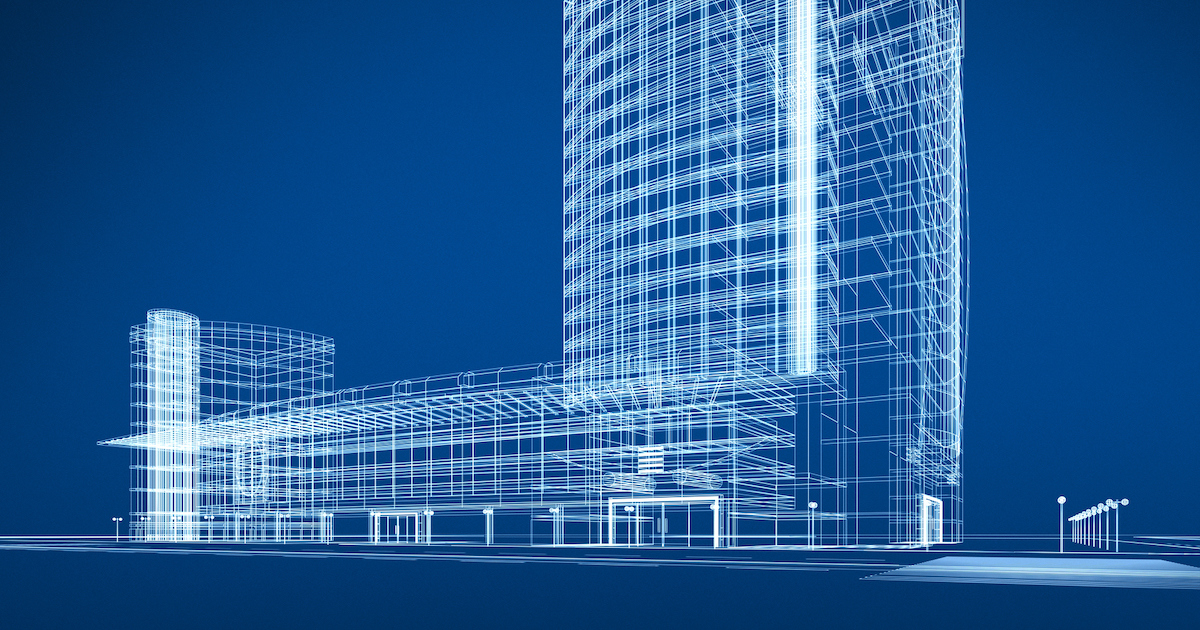
DoorDash rearchitected the heterogeneous caching system they were using across all of their microservices and created a common, multi-layered cache providing a generic mechanism and solving a number of issues coming from the adoption of a fragmented cache.
Caching is a common mechanism used to optimize performance in a system without requiring expensive optimizations. This was especially relevant in DoorDash case since implementing business logic has higher priority than performance optimization, explain DoorDash engineers Lev Neiman and Jason Fan.
Unfortunately, different teams at DoorDash relied on different caching systems, including Caffeine, Redis Lettuce, and HashMaps, which also meant they were experiencing and solving again and again the same issues, such as cache staleness, heavy dependency on Redis, inconsistent key schema, and more. For this reason, a team of engineers set off to create a shared caching library for all of DoorDash microservices, starting with DashPass, a key service that was experiencing scaling challenges and frequent failures due to increasing traffic levels.
The first step was defining a common API based on two Kotlin interfaces: CacheManager
to create a new cache for a specific key type and a fallback method, and a CacheKey
class abstracting over key types.
This allows us to use dependency injection and polymorphism to inject arbitrary logic behind the scenes while maintaining uniform cache calls from business logic.
While striving to keep the cache simple, DoorDash engineers opted for a multi-layered design with three layers to push the possibility of performance optimization further. In the first layer, named request local cache, data reside in a hash map and its lifetime is bound by the request’s. In the second layer, the local cache, Caffeine is used to share data among all workers within the same Java virtual machine. The third layer is the Redis cache visible to all pods in the same Redis cluster and using Redis Lettuce.
An important feature of this multi-layered cache system is runtime control, available for each separate layer, to enable turning the cache on or off, setting the cache time to live (TTL), or shadow mode, where a given percentage of cache requests are also compared to the source of truth. Additionally, the cache system included support for metrics collection, including hits and misses, cache latency, and for logging.
Once the cache system was ready and working as expected for DashPass, it was progressively rolled out to the rest of the organization, with clear guidance about when and how to use it or not to use it.
According to Neiman and Fan, the new cache system improved scalability and safety across all of their services while also making it simple for teams to adopt a cache when necessary to improve performance.
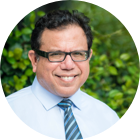
MMS • Sergio De Simone
Article originally posted on InfoQ. Visit InfoQ
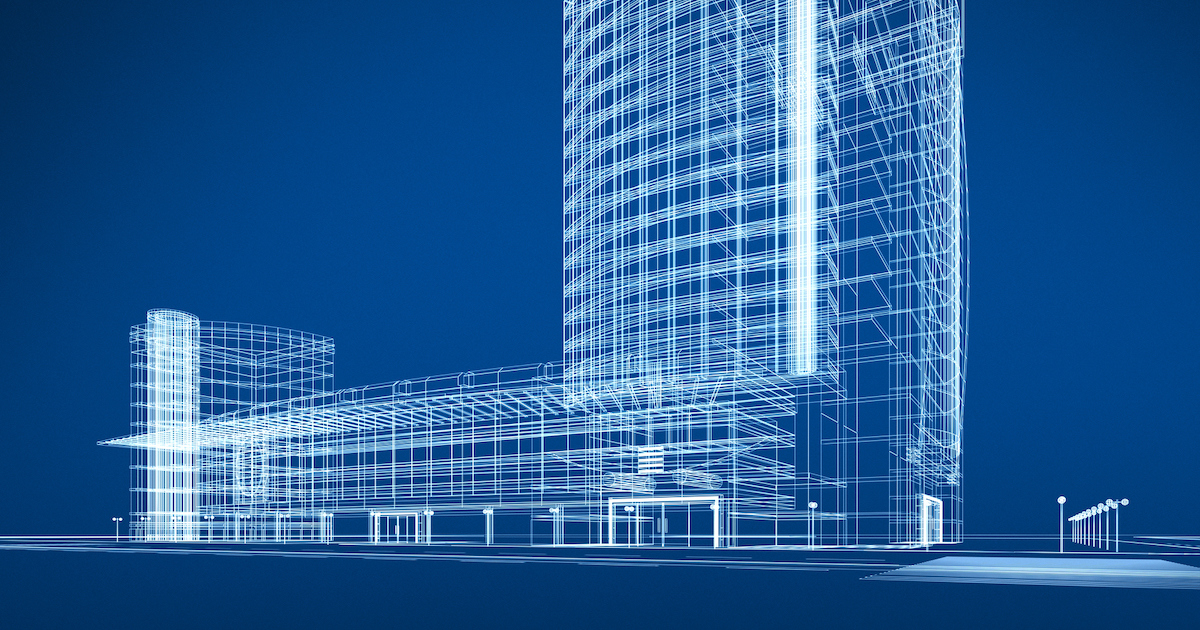
DoorDash rearchitected the heterogeneous caching system they were using across all of their microservices and created a common, multi-layered cache providing a generic mechanism and solving a number of issues coming from the adoption of a fragmented cache.
Caching is a common mechanism used to optimize performance in a system without requiring expensive optimizations. This was especially relevant in DoorDash case since implementing business logic has higher priority than performance optimization, explain DoorDash engineers Lev Neiman and Jason Fan.
Unfortunately, different teams at DoorDash relied on different caching systems, including Caffeine, Redis Lettuce, and HashMaps, which also meant they were experiencing and solving again and again the same issues, such as cache staleness, heavy dependency on Redis, inconsistent key schema, and more. For this reason, a team of engineers set off to create a shared caching library for all of DoorDash microservices, starting with DashPass, a key service that was experiencing scaling challenges and frequent failures due to increasing traffic levels.
The first step was defining a common API based on two Kotlin interfaces: CacheManager
to create a new cache for a specific key type and a fallback method, and a CacheKey
class abstracting over key types.
This allows us to use dependency injection and polymorphism to inject arbitrary logic behind the scenes while maintaining uniform cache calls from business logic.
While striving to keep the cache simple, DoorDash engineers opted for a multi-layered design with three layers to push the possibility of performance optimization further. In the first layer, named request local cache, data reside in a hash map and its lifetime is bound by the request’s. In the second layer, the local cache, Caffeine is used to share data among all workers within the same Java virtual machine. The third layer is the Redis cache visible to all pods in the same Redis cluster and using Redis Lettuce.
An important feature of this multi-layered cache system is runtime control, available for each separate layer, to enable turning the cache on or off, setting the cache time to live (TTL), or shadow mode, where a given percentage of cache requests are also compared to the source of truth. Additionally, the cache system included support for metrics collection, including hits and misses, cache latency, and for logging.
Once the cache system was ready and working as expected for DashPass, it was progressively rolled out to the rest of the organization, with clear guidance about when and how to use it or not to use it.
According to Neiman and Fan, the new cache system improved scalability and safety across all of their services while also making it simple for teams to adopt a cache when necessary to improve performance.
Presentation: Offline and Thriving: Building Resilient Applications with Local-first Techniques
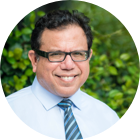
MMS • Carl Sverre
Article originally posted on InfoQ. Visit InfoQ
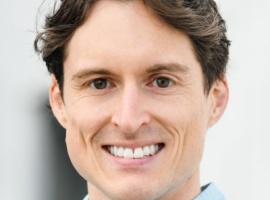
Transcript
Sverre: We all get really used to loading spinners. We see this all the time, and it’s almost like something we just accept. We see it, and we’re just like, take a deep breath, wait for it to go away or whatever. We’re all really used to this. My talk is going to be about whether or not this is a necessary evil. Like, do we need this? Do we have to always put loading spinners in our applications? Maybe there are some alternatives. I’m going to be talking about an idea called offline-first, or local-first. This is a definition that ChatGPT came up with. Basically, it’s pretty accurate. An offline-first application is designed to function effectively without an internet connection. Critically, it has the capability to synchronize with whatever other systems are connected to it, when it is able to. This is the definition I’m going to be using whenever I talk about either offline-first, or local-first technology.
You have used an offline-first application. I absolutely guarantee it. Here are some examples. WhatsApp is an offline-first application. Pretty much any email client you’ve ever used is an offline-first application, calendar clients as well. If you think about it, a lot of the functionality of those applications work without the internet, like WhatsApp. The thing you think about with WhatsApp, like what’s the purpose of WhatsApp? Sending and receiving messages. It’s also about searching your messages, seeing what you’ve sent before, queuing up things, looking at images from your mom. Basically, WhatsApp is absolutely an offline-first application. Similarly, email clients, calendars, and actually probably most of the apps on your phone are some definition of offline-first. Offline-first is everywhere. This is not a new concept. However, the technologies we have to basically make offline-first applications easier to build and safer to build have gotten a lot better over the years. I’m going to be talking about exactly that.
Background & Outline
My name is Carl Sverre. I’m currently an entrepreneur in residence at Amplify Partners, which is a venture capital company in San Francisco. An entrepreneur in residence is basically someone who is making a company and doesn’t quite know exactly what that company is. It’s like a pre-preseed position. I’m still figuring that out. The research of this talk really helps me out and I will very likely be doing something in the offline-first space. I previously worked at a company called SingleStore, which is a database company. I’ve worked there for the last decade. We’re going to be talking about three things, first, offline-first. What is it? Why is it interesting? I want to present a couple of high-level ideas that offline-first enables in your application. The second thing is some case studies. Just talking about some companies. I’m going to talk again about WhatsApp. What makes them offline-first. How they are offline-first. I’m going to be talking about a couple other companies as well. Finally, I want to talk about getting started. Let’s say you leave this talk being super amped up about offline-first like me, what do you do? What are the tradeoffs you have to consider in how you build your applications? What are the problems? Nothing is a silver bullet. If you go offline-first, you are going to have a whole bunch of new problems you have to deal with. Of course, you gain some other things that you don’t have to deal with anymore. There’s always tradeoffs.
Why Offline-First?
Why offline-first? This slide again, spinners suck. This is the thing that I am drawn to the most about offline-first. I hate opening up my phone and opening up an app and immediately seeing a loading spinner. I find it really frustrating. I’m trying to move fast. I’m trying to get stuff done. A lot of things that I’m doing actually don’t require internet access. I know the data is already on my phone, cached there. I’ve just looked at it maybe 10 minutes ago, because I’m one of those people who constantly brings out their phone. The data is there. Why can’t I just see it? Why do I have to wait again for a REST API access? Offline-first is a pattern which allows you to remove a lot of loading spinners in your application by making certain tradeoffs about essentially consistency, conflict resolution, and eventualities. We basically are trading off getting exactly the right thing for user experience, like a better lower latency experience. Latency is the first thing.
The second one is reliability. Let’s talk about post offices. If you take a package to a post office, you would be really annoyed if they were like, “Sorry, we’re having internet problems. Can you come back tomorrow?” That doesn’t happen. You take your package to the post office, they’re immediately able to take all your information, even generate tracking IDs and stuff like that for the package. When they eventually have internet, it works. That’s because post offices were designed around this idea, they wanted to be a very reliable solution. They didn’t want to be dependent on having perfect connectivity to a function. Most post offices have this property that they are effectively offline-first. The post office can continue to operate in absence of the internet, or if the server is having problems, or whatever.
The third thing is collaboration. I propose that offline-first makes collaboration easier. This might be a little bit counterintuitive. Let’s consider the collaboration spectrum. If we think about all operations as being somewhere on the spectrum from low latency, so something that you might think of as like a real-time application. You have high latency, like probably using your bank. These are how most applications think about API interactions. What happens when the limit of latency approaches infinity? Of course, you get offline. Within collaboration, it’s like, how does offline-first help with collaboration? Offline-first is a forcing function. You have to be able to handle a user having essentially data on their application for a period of time without knowing about that information. Your cloud service has to basically accept that there’s some authoritative data on the user’s device that you want to be able to receive at some point in the future. You want to be able to incorporate those changes into your data model on the cloud. By building your data model with this restriction in mind, it forces you to essentially build a data model that can handle collaboration, because collaboration is nothing more than exchanging data with other people and being able to interlace those changes into a cohesive result. The cool thing about offline-first is that you can build collaborative apps, like real-time collaborative apps using the same technologies that also give you offline-first applications.
The fourth thing that I want to present is development velocity. Tuomas Artman, the cofounder of Linear, which is a task management tool that’s very similar to Jira, presented this in a really great way on Twitter. Tuomas Artman talks about offline-first as something that gave their team faster development velocity. Why is that? The reason is that they found that the synchronization engine, the thing that asynchronously synchronizes the client with the server was handling all of the complexity around APIs, network latency, retries, reliability, caching, all of these things that normally you’d have to consider as a developer, suddenly disappeared. Because of this, they were able to move faster. Developers would basically just mutate the data locally, it didn’t really matter what they did to the data, and their synchronization engine would handle dealing with essentially sending it to the server. In effect, they had a networked application with real-time collaboration, with offline-first capabilities, and they don’t actually have an explicit API. That’s a really cool property. It enables faster development velocity. I’m going to propose that these four things are fantastic features of building an offline-first app. You have performance, reliability, collaboration, and development velocity.
Case Study 1 – WhatsApp
Let’s talk about some case studies. The first case study is WhatsApp. As you can see from the screenshot, this is WhatsApp on my phone. WhatsApp is 148 megabytes. We shouldn’t probably make smaller binaries, but that’s a different conversation. More interestingly is that it’s storing 1.2 gigabytes of data on my phone. That’s not a bunch of photos, that’s a bunch of text. I’ve been using WhatsApp since WhatsApp came out. I use it to talk to a lot of people. Interestingly enough, the entire history of all of my WhatsApp conversations are on my phone. That’s awesome. I search WhatsApp all the time. I use it as a way of backing up information. I send messages to myself. These are really nice features, and I know that when I open WhatsApp, I’m never waiting for a loading spinner. That’s a cool experience. It’s like a thing that’s just always available on my device no matter what. WhatsApp is an offline-first app, and they also have a bunch of other features that were made easier because of this offline-first architecture, end-to-end encryption, on-device messages and media. They have the ability to queue up messages at a later time. They have the ability to download rich media, videos, stuff like this. All of this is supported by background synchronization. If you want your app to be more like WhatsApp, consider offline-first architectures.
Case Study 2 – Figma
The next one is Figma. This is a really fun video that I love to show, which is probably 10 or 15 people all interacting with a Figma document at the same time. Figma is like an online version of Adobe Photoshop, and it’s a vector editor, against Illustrator. It’s very similar to that. It’s entirely in the web browser. It uses really cool technologies, everything from WebAssembly to other things to work in a really performant way in the web browser. What’s really cool and relevant to this talk is that the data model that they built at Figma is offline-first. The data model that they built, there’s a tree hierarchy for the document. The document stores all of the properties of every single object in essentially a CRDT. A CRDT is a Conflict-Free Replicated Data Type, which is a data structure that can be merged in any order to achieve the same consistent result at the end of the day. This works really well in the offline-first world. I can take a Figma document offline. I can edit it. When I come back online, those changes can be interlaced with other people’s changes, and we can arrive to a consistent document state. Figma is a really good example of how offline-first enables both real time collaboration and offline-first collaboration. It’s one of my favorite applications to showcase that.
Case Study 3 – Linear
The third application is Linear. We talked about Tuomas Artman a little bit earlier, about how offline-first helped him build faster development velocity at Linear. Linear is itself an absolutely world-class example of offline-first. Linear is like a Jira alternative. If you know anyone using Linear, one of the first things they might say is that it’s super-fast. When you interact with Linear, it’s like every single thing you do is instant. You might ask, how do they do that? It sounds like magic. It’s done because every single thing you do is local, it only runs on your device. There’s no network traffic associated with editing an issue, creating a new issue, any of that stuff. Instead, all of that is deferred to background synchronization. Tuomas Artman made a really interesting observation, in addition to offline-first enabling, essentially, development velocity, it was a lot simpler to build than he originally thought. The reason was because the amount of conflicts that happen in a huge task management data structure is actually relatively infrequent. How often are you and another person editing this exact same issue at the exact same time? It’s much more infrequent than you creating an issue and someone else creating another issue. These changes naturally converge to the same state. Linear is another example of offline-first being used to build a really fantastic application.
Backend Use Cases for Offline-First
I do want to talk a little bit about backend context. Here are a couple of backend-first use cases. So far, we’ve been talking about apps that really focus on the user. They’re user facing applications, and there’s a human in the loop interaction that happens. Here are some examples of where offline-first can help more like backend applications. Just talking about the first one, so a ship at sea with a flaky, low bandwidth satellite connection. If you think about it, a lot of cruise ships actually run small data centers, like on their cruise ship. It’s a pretty common paradigm, because they have a lot of services to run. They need to be able to track credit card purchases. They need to be able to do things like coordinate room service. There’s a million little things that they have to do with these massive cities that float at sea. Running that over the internet over a satellite connection is a really expensive proposition. Not only is it expensive, but it’s also very unreliable. It’s much safer to be able to run everything as an independent data center. How do we do that? Erica’s talk showed off the Snowball. I hadn’t seen that before, so I want to refer to it because I think it’s another great example of an offline-first backend thing. You have this massive physical thing that you ship to any location and suddenly you have like a [inaudible 00:15:19] AWS data center, you can run lambda, you can store data on it. Ultimately, that data can be reincorporated into the cloud version of your dataset. This is another example of an offline-first mental model of how you build around these solutions.
How to Get Started with Offline-First
This is the meat of the talk. I’ve established so far why I think offline-first is really cool. I’ve presented a couple of companies that are doing it, so it’s not just like a hypothetical idea. What do you do next? How do you get started if you’re excited about this idea. Let’s talk about normal applications. The specific application I’m going to be referring to is a user facing app, maybe a web app, maybe a mobile app, but something that involves a user connecting to a cloud service over an API. Everyone here has either built something like this, or at least thought about something like this. It’s pretty straightforward. You have a user in the top left, they are using an app. They’re connected to the cloud over maybe a REST API or a GraphQL API, some kind of network link. Ultimately, that data is stored in the database. There’s a couple of complexities here. You have to build a REST API. To build a REST API, you ultimately have to think about your data model on the server side. You have to think about your data model on the client side. You have to basically figure out how to map those things together. It’s very common that the data model that renders in the client is not actually the same as the data model that renders on the server, like that exists in the database. There’s usually some kind of transformation, and that either happens in the REST API, or it happens in your application.
Eventually, if you go down this path, you’re going to eventually want to add some performance. Some user is going to be like, why do I have to wait for this network thing to finish before I see the results of the thing I click? You’re going to add some performance optimization in that way. Waiting on the network is not going to be sufficient for that performance you want to provide. The most common thing that people do is they add some cache. The joke in computer science is like, once you add a cache, now you have two problems. I think it’s very true, especially when you add like a cache with the goal of essentially building an optimistic mutation layer. Essentially, whenever you run some mutation in your app, you’re effectively optimistically mutating the cache. Then you go to the server, you run your mutation on the server. Then you get back the results from the server, you update your cache, and you re-render your application. There’s a huge problem with this. It’s not something that everyone thinks about explicitly, which is that in order to do this correctly, you often need to have a very deep knowledge of what happens on the server side to be able to correctly invalidate the cache. That’s because if you make a change in one part of your data model, it may actually result in a different query being invalidated. You have to understand that. We depend on our developers to do this. We depend on our developers to be correct and understand our data model super well, so that they know exactly what subset of the cache to invalidate after every single mutation. I propose that that’s not a scalable solution. It’s much better that our users, our developers didn’t have to think about the interrelationships between different parts of the data model to be able to correctly model a cache on the client side.
I think this is a really complex solution, so what is an alternative? Maybe you could build an offline-first application. This is one way you could build an offline-first application. You can essentially run a full-blown database in the client. That sounds really crazy and complicated, but it offers some interesting advantages. The first thing is that now the app doesn’t have to think about the server at all. The app simply just mutates the database. It sees the data, it’s able to run queries, it’s able to run mutations on the database. As it does so, it’s not thinking about how am I coordinating with a server? The data model is there. In the background, there’s some asynchronous mechanism that is essentially replicating the database that’s running in the client into the server, and vice versa, establishing some multi-master link between the client-side database and the server-side database. Critically, this is asynchronous, so don’t worry, everyone’s red flags are going off. Everyone here is senior architects. Everyone’s been like, that sounds like a bad idea. It has some tradeoffs. There are complexities that arise once you try to do multi-master asynchronous replication. If it works magically, let’s assume for a second, this is a really great way to build an application. Like Linear actually shows that this way is a very sufficient way to build certain kinds of applications. This is how their architecture works. They have a local database running in the client. They have a database running on the server. There’s asynchronous synchronization that happens. Their client code, their actual app never thinks about the API, never thinks about anything, it simply mutates this database. That is, in my opinion, a very beautiful result. Offline-first architecture, this is loosely what it looks like.
The Downsides of Offline-First Applications – Conflict Resolution
It sounds awesome. What’s the catch? What’s the downside of this? You got a couple of new problems that you have to deal with. You have to deal with a lot of problems that databases have to deal with. You have to deal with them in a completely spread out, distributed system involving many different devices and many different users. It’s a really complex problem. We have to be able to understand these problems really well. We have to be able to address them with really clear tradeoffs to be able to make an app that you can reason about. The remainder of this talk is going to be talking about each of these different problems in a little bit of detail, to make sure that everyone understands the problems and also some suggested solutions, or path to solutions, or maybe just some ideas about how to think about this and reason about it.
Conflict resolution is the first problem that I want to talk about. Let’s consider that we have users A and B, both using some eventually consistent offline-first data model. At time 0, they both agree that the color of some object is green. Then at time t plus 1, they both change the color of the object to some other color, so now the color is blue and red, but they haven’t yet communicated about this because they’re connected via an eventually consistent asynchronous solution. At time t plus 2, they synchronize and it occurs to them that they’ve both made the same change at the same time. Conflict resolution is basically deciding what to do in this situation. What are some options just from looking at this image? We could just reject one of the changes. We could basically order the users by their ID, so A and B. We could just say the highest ID wins. In this case, blue wins. Or maybe we have a really fancy application, and we basically are like, it’s purple, they both win. You could solve this in any number of ways. It’s not necessarily true that any of those ways are correct. It really depends on your business, what your application is doing. You do have to think about it. That’s what I’m trying to convey right now in this talk.
You’re not on your own, there has been a lot of research in this topic space. Here’s just a small subset of the most common solutions to distributed conflict resolution in an offline-first context. The first one is branch rebase. You can loosely think of this as GitHub or Git style conflict resolution. We have multiple branches, and we want to basically rebase branches onto each other. Rebasing effectively says like, any changes that have already happened, we want to agree, ok, they’ve happened. Then any new changes we want to replay, and then there’s some merge resolution type problem. Oftentimes, branch rebase results in requiring a human to be involved. That’s why in Git, hopefully your merge just works, but every once in a while, you get some merge conflict, and you have to go and fix it yourself. Branch rebase is a method of conflict resolution.
The second one is conflict-free replicated data types. I mentioned that one earlier with Figma, so CRDTs. Conflict-free replicated data types are amazing. They’re one of my favorite data types and technologies. They allow basically fairly complex data structures to be created, that can merge in any order and get the same consistent result. The simplest version of this that you can all probably visualize is just like an integer counter, that can only count up by one. Let’s just keep it really simple. The operation is increment, and the state is the total that sum of all the increments. If you receive all of the increments in any order, you will get the same value. It doesn’t matter the order in which the increments come to you. This is obviously an extremely trivial example. People have used this exact same concept to build extremely complex data structures, and it works really well. CRDTs are really cool.
The next one, operational transformer, OT, is the system that is used by Google Docs. If you’ve used Google Docs, the word processor, this uses operational transformer. OT is a very complex conflict resolution method. However, it leads to pretty reliable systems if you actually build them really well. I think docs is probably at least the one that everyone knows is very mature and generally works really well. Most people now, if you’re starting a project from scratch, you probably should go down the CRDT path first. I like to think about them as like CRDT is basically like a Raft consensus, if you’re familiar with consensus algorithms, and OT is like Paxos consensus. The fourth one is, last write wins. You have some way of determining order, and you can’t just use physical clocks, because physical clocks are basically wrong everywhere. You can’t depend on a device’s physical time being correct, so you need some way of creating a logical version of time. We call that logical clocks or vector clocks. This allows us to deterministically order all of the transactions and apply them consistently on all the clients. Finally, we have one of my favorite ones, which is Hypothetical Amnesia Machine, or HAM. This sounds like very esoteric, most people don’t know about it, but I definitely recommend checking it out. It’s a part of gun.eco, which is the database for freedom fighters. Just go look it up. It’s crazy, but very fun.
Eventual Consistency
We’ve talked about conflict resolution. Now our application has decided this is how we’re going to handle a conflict. When two things happen at the same time, we don’t know exactly how to determine. We’ve come up with a method to resolve those conflicts. The next thing you have to think about is eventual consistency. These two problems are very related, because eventual consistency implies that we have some asynchronous communication protocol. Conflict resolution basically arises when you have an asynchronous communication protocol, because you don’t synchronously all see the same state. Eventual consistency is the second problem, so let’s walk through with this example. It’s going to be the bank balance example that everyone learns in distributed systems classes. It’s a pretty great example. We’re going to start off, both A and B agree that there’s like $100 in an account. Let’s say that, at the same time, A and B decide to do the following operations. B says I’m going to withdraw $30 from the account, ok, great, it’s now 70 bucks in the account. A is going to say, what is the current balance? 100 bucks, in some function. Then the next thing that happens is that B sends the value, like synchronizes and asynchronously, $70 over to A. Probably some people might already see a problem here. This function has no idea what happened. It is like an external piece of temporary state that is still executing concurrently with the rest of the application. The function decides to also withdraw some money, so it withdraws 50 bucks, and it posts that back into the local database. Now we end up with a situation which maybe starts to look a little bit problematic, which is that we’ve somehow created money, and we don’t want to do this. Now, I definitely do not recommend building banking applications in an offline-first context. It leads to things like double spending problems. You have to deal with that in a different way. I think this is a great way of explaining eventual consistency and the second order effect of asynchronicity with regard to how applications are generally written. There’s ways to address this, which we can talk about.
Some methods that you can deal with, with handling eventually consistent systems. The first one is like a compensation. I probably should have made these examples consistent, but I find these other examples easy to talk about quickly, which is a room booking software. If you think about a Google Calendar, and you go onto your Google Calendar, and you want to book a room. Let’s say the Google Calendar was offline-first, which it sort of is, and you go book a room at the same time someone else books the same room. How do we deal with this problem that both users have seen that the room is available, so they’ve essentially run a read query to say, ok, the room’s available. Then they’ve both written into the database that they want that room. Then at some point, the system synchronizes and realizes that the room has been double booked. The first way you can solve is like a compensation, just book a different room. The user could potentially submit like a priority. Instead of saying I want this specific room, they could say, I want a room with these criteria. Or, you could simply just book them into a different room and notify them. There’s a lot of ways to deal with compensations. Within the eventual consistent world, compensations is any automated solution that you’ve pre-decided is acceptable to solve an eventually consistent problem.
The second one is error handling. A room is double booked, just tell one of the users that, we’re really sorry, we tried really hard, but that room is taken. The thing to observe about human in the loop systems is that they don’t move that fast, like computers move way faster than human loop systems. These types of consistency violations don’t actually occur as much as the regular everyday behavior. You just want to make sure that your system is synchronizing fast enough and is online enough that you can minimize these types of cases. Error handling is a great way to handle the bad condition where they actually do conflict. The third one is versioning. Essentially, keep around all of the potential states. Instead of having the winning state overwrite the losing state, you keep around all of the states. You basically say, the room is booked by these three different people. Then you have a second workflow that runs which might include a human, or might include an AI, or it might include some other reasoning system, which is basically able to collapse those multiple versions into a single version. In the room booking example, that would be a little bit weird. Maybe you have an admin who ultimately says, they get the room, they win. For example, if you imagine a text field that you’re trying to merge, so if you imagine like Jira, and you had two different edits to the same text field, you could automatically resolve this using like an AI, which I think is a really interesting idea that I’d like people to pursue. Imagine giving an AI the original version of the text field and two edits of the text field, GPT probably wouldn’t do a terrible job at actually just coming up with a version that incorporated the intentions of both of those changes. The point is that eventual consistency, you can solve these problems. You can build them into the business of your application, however, there are going to be tradeoffs. It’s not going to be a perfect solution, but you’re getting a lot of other advantages. With any tradeoff, there’s always both sides to the coin.
Device Storage
The next one is storage. Basically, user devices have really bad storage. If you’re running an offline-first app, it means that some portion of your application is running on a user’s device. If it’s a mobile app, and mobile apps are actually getting really good. As Erica pointed out in her talk, like people still run really old mobile phones, and you’re going to have to deal with that. You have to basically handle the reality that you probably won’t have very much space, you probably won’t have very performant space, and you almost certainly won’t have very durable space. This is just true for consumer devices. They’re not like fancy AWS web servers with RAID 10, and NVMe drives. Some people have those but not everyone. You have to be able to handle that. When you’re building an offline-first app, the ways to handle this is a couple of different things. The first thing is, build performant offline-first apps. Consider how the data structures that run in your offline-first app behave on basically slower media. Ultimately, it’s a problem of performance optimization. You want to be able to do less. A project that I’m currently working on is putting SQLite in devices, and hooking them up into an offline-first asynchronous pattern. An advantage of using SQLite is that SQLite is really well designed. It’s already designed to do very low latency operations for certain kinds of workflows. It has things like indexes. The point is that SQLite is already really well architected. By putting them in our applications, we can take advantage of that and hopefully be able to handle some of these sort of performance problems better. Again, when you’re building offline-first, you will have to consider device storage as a main key point of how you’re building your app.
Access Control
Access control is really interesting in an offline-first context. Ultimately, access control boils down to authorization, like, who’s allowed to do what? Also, visibility, who’s allowed to see what? There’s what you could be allowed to do and what you’re allowed to see. Let’s talk about those two ideas separately. To encode what you’re allowed to do, we basically depend on a trusted authority to make that decision. In an offline-first context, we have a bunch of devices that are all essentially mutating shared state. If they all have equal permissions, then this is fine. You just let them all mutate the shared state, there’s no problem of like, one device should be allowed to mutate something that another device is not allowed to mutate. If your app does require asymmetric authorization, this becomes a lot more complicated. The solution that some companies have done, like I think Linear does this, is they revalidate all of the changes that each client does on the server side. When they do the background synchronization, they also are revalidating. If there’s a problem with it, then they can reject it. They can show the user a notification or an error. They can provide some error compensation. They do this asynchronously. Because if the user is running the Linear client, like they aren’t hacking it, it already knows how to run authentication and authorization locally, which means that it’s already basically not allowing the user to do things they’re not allowed to do. In the general case, this doesn’t result in a slower laggy solution or a lot of rollbacks. You do have to add this to your application at a centralized location, because you need that authority. That’s authorization.
What about visibility? Visibility is actually really tricky with offline-first, because with offline-first you need to synchronize some amount of data to the user, and then be able to at a later time move mutations that they’ve done to that data back up to the server. You need to always know exactly what subset of the overall dataset exists on that client in order to be able to correctly reconcile those changes. How you solve visibility is ultimately going to be dependent on how you do synchronization. If you’re using some database replication approach, where you’re replicating an entire SQLite database to every single client, then you need to make sure that everything in that SQLite database is allowed to be seen by every single client. If you don’t have that case, if you have an asymmetric solution, and you have that architecture, you may need to look into things like partial replication and sharding, basically, to be able to split up your physical data into a bunch of logical data silos. Then you can synchronize those logical data silos based on permissions. Two things you have to consider, authorization and visibility.
Some people in this group might be thinking, what about cryptography? Cryptography does provide pretty compelling solutions to these two problems. Encryption solves for visibility. If I encrypt a file, I can be reasonably sure that only people with the key that I encrypted for can decrypt it. Similarly, if I want to provide some authorization, I can encode those authorizations in a shared-nothing approach using signing, by signing things saying everyone agrees that this thing requires this signature in order to append to this log. There’s a lot of different ways to do both visibility and authorization with cryptography, as we’ve seen through the world of blockchain and crypto. However, there’s a lot of complexity that comes along with cryptography. You have to now deal with key management, so you have to now make sure that users actually have their keys, their keys aren’t getting stolen by someone else. Just a huge amount of complexity. With all tradeoffs, there’s always both sides of the coin. Cryptography offers a lot of great solutions in this area, but at a fairly high cost.
Application Upgrades
Finally, I want to talk a bit about application upgrades in an offline-first context. In an offline-first context, you now have versions of your data stored across many different devices. Imagine the complexity of just a normal cloud app, you have one database and you want to migrate the schema, so you run migrations. That’s already pretty hard to do correctly with your app, make sure that nothing goes wrong. Now let’s imagine that you have a thousand versions of the same database and they’re all running slightly different versions of the schema, and you want to be able to upgrade them over time. This is a huge problem. It’s a very complex problem that you will have to consider how you solve. It’s actually even a little bit worse in offline-first, because not only do you have thousands of versions of schemas, but you also have mutations that were run locally on those thousands of versions of schemas. All of those mutations have to eventually make their way back to a server, and all converge into a consistent view of the same state, not as really complicated because when you run a mutation or a migration, you have to basically migrate not only all of these schemas, but also all of the intermediate, not yet fully committed change log effectively. Application upgrades is a huge problem. Luckily, the world of research has been really focused on this problem. There’s a whole bunch of people and organizations working on this issue. One idea that they came up with was data lensing, or data translation. The idea is that not only should you build migrations to the actual structure of your state, but you can also essentially write lenses which allow different versions of your application to, at read time, change a piece of state into what they expect. If you imagine versions 1 and version 2 of my application, I migrate some data to version 2, but my app version 1 still needs to see that data. It needs to be able to, at read time, downgrade that data back to what it expects to be able to read it. Data lensing is this idea of building these lenses that allow you to translate between different versions of your data. Data lensing and data translation is a really useful method to be able to make your system more reliable.
The other thing about application upgrades is reducing the amount of your application that is offline-first. I definitely want to make it clear that I’m not saying necessarily that every application should be entirely offline-first. There’s a lot of places in your app that you just don’t need that complexity, but for the parts of your application that could really benefit from offline-first, so, for example, a real-time collaborative part of your app. That is a very small subset of the overall application. It’s a little bit easier if you reduce the scope of what is offline-first to a smaller subset. You should make sure that the user can still access that smaller subset without internet access. That’s the critical piece of it. If you do do that, you can have a great experience. Figma is a good example of this. Figma, the document editor, which is the thing that actually lets you edit documents and do all the fun visual things, that is the main offline-first part of the overall application. If you go to your user profile, and you want to change your password, that’s not offline-first. That still requires a synchronous connection to the network, because you don’t really have to do that in an offline-first context. You don’t need that feature to be able to edit a doc that already synchronized locally. Something to think about is application upgrades and how you’re going to deal with it.
Summary
That’s a lot of tradeoffs. I as a software engineer, as someone who’s been software hacking for a while, I like to be very realistic about software. I don’t want to just stand up here and say offline-first is perfect, because it’s not. It has a lot of tradeoffs. However, offline-first is pretty good. Loading spinners suck. I really dislike it. If I can build apps that show my users this less, they’re going to be happier. I’m willing to live with those tradeoffs and make my life more complex in exchange for a better user experience. Offline-first is a way to provide performance, reliability, collaboration, and development velocity to both your team and your users. I think that that is worth at least us thinking about when we’re building our applications. You should absolutely build at least a little part of your app as an offline-first app.
Questions and Answers
Participant 1: When you’re thinking also these tradeoffs between the different modalities of how to handle offline-first. I’ll use Figma as an example. They’re not a pure CRDT, they just have a CRDT process server that hops off the connection. If you’re modifying two different properties then obviously there’s a conflict, but if you’re modifying the same property, they decided if you’re just going to take your time, you have one that hits the server first, take your timestamp of that and basically [inaudible 00:43:30]. They have a design time decision that said that, that’s ok for our application. If I was to go write my own offline-first application to solve this sort of problem, is there a set of heuristics for thinking about some of those tradeoffs between these different techniques?
Sverre: When humans are in the loop, I think it helps a lot with some of these tradeoffs because humans are pretty forgiving. They aren’t always that forgiving. I’m actually a lot less forgiving about latency spinners than every once in a while, having something flip back and forth. One really interesting observation about Figma and Linear and other real-time collaborative applications is that you see other people editing the doc oftentimes at the same time as you. If you do truly go offline, you go on a plane and you edit a document, and you resynchronize, at least most people that I’ve talked to who have done that are in one of two categories. They either are the only person who’s working on the document, which devolves to just an offline-first document editor. Or they’re very accepting if someone else is working on the doc at the same time that the changes don’t synchronize exactly perfectly. This is true for Google Docs as well. If you write a Google Doc, and someone else edits a Google Doc but offline, like you have no coordination at all, and you come back and your paragraphs are interlaced, you might be a little annoyed, but it’s an expected result. When humans are in the loop, you can be a little bit fuzzier about these things than if you were making an app that was entirely a backend app. If only computers are involved, the computers are not accepting at all of inconsistencies. If suddenly your JSON is interlaced with some other JSON object, and they’re just one big JSON object, it’s not going to work. Humans are actually pretty fuzzy, and so we can make our offline-first apps a little bit fuzzy, which allows them to be simpler to build.
Participant 2: I have a question about the conflict resolution afterwards. In a previous role, we used operational transformers to really great effect, and loved it. One of the things that we noticed over time is that, later we got more users, and the size of the database that continued to operationally transform operations was very large. You also made a comment about storage capacity on some devices. Is there a particular conflict resolution method that you suggest that is going to be a bit more mindful of if you change the device storage?
Sverre: That’s something that is actually very active in research right now in the offline-first space. CRDT’s research and a lot of the research that has gone into newer alternatives to text editing specifically have started to address this particular problem of essentially snapshotting over time. If you only ever keep an event log and you never snapshot, it’s relatively easy to eventually converge to the same state, because everyone just keeps on exchanging all the event logs until everyone gets the same event log, and you get the same state. If you introduce snapshotting where you have to decide on a consistent state that you know that absolutely everyone has seen to be able to correctly snapshot the data and remove all the older event log, because if you do that at the wrong time, you might basically leave someone hanging. They come back online, and they’re like, “I’d like to replicate.” You’re like, “I’m sorry, we deleted all of the event log you need to be able to catch up to the snapshot.” This is really hard.
In terms of solutions, actually, I think there’s a solution in the business side that’s a little bit easier to deal with than the solution in the technical side. The solution in the business side is basically making the realization that a lot of parts of apps that need to be offline-first don’t need to be offline-first for that long. Figma is a good example. When you open a document, if no one else has checked out that document in the last 30 days, you get a new snapshot version of the document that doesn’t have an event history. They have a policy in their docs that they only have 30 day offline-first windows. They keep track of everyone who’s opened a document and the last time they’ve synchronized. They basically are trying their best to basically say, we know there’s a low-water mark. We know everyone who’s checked out a version of this doc, and we know that they’ve synchronized at least up to this point. Even if that point is at the beginning of a 30-day window, their particular CRDT architecture and data structure is able to keep around like 30 days per doc without too much overhead. They’re not keeping around data forever. They’re saying that if you open the doc, and then wait 31 days, you probably will not be able to synchronize back into the system. Again, it’s like offline-first is a lot about interacting with your users and knowing that they’re going to accept fairly fuzzy solutions to these problems.
Participant 3: I’m really excited about that. The only concern I have is like when it comes to access control, revocation, and stuff like that, because like you’re getting offline, you get fired, you have some access concerns to sensitive information. Or you want to manage access, you revoke a user, you add a user, you’re offline, you come online later on. All of those, is it anything about like, and I’m thinking offline-first approaches?
Sverre: Hundred percent. First of all, there are certain workloads that should not be offline-first. If it’s a critical workload that involves an employee database, where continued access to that database would be a risk that your organization cannot take, you’re willing to pay the tradeoff for latency for that security. If you’re going down that path, I would really recommend Erica’s talk where she talks about how you can make your normal centralized applications a little bit faster by using the edge. It’s like, there’s other solutions for those problem spaces. There’s a lot of cases where the difference between an offline-first app and old-school just desktop apps is actually pretty similar. If we think about an old Microsoft Office, like you downloaded a Word document, you still have it on your computer, and we try to say like, give your computer back and don’t back it up on USB, but we can’t really stop that.
See more presentations with transcripts
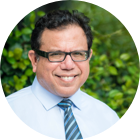
MMS • Almir Vuk
Article originally posted on InfoQ. Visit InfoQ
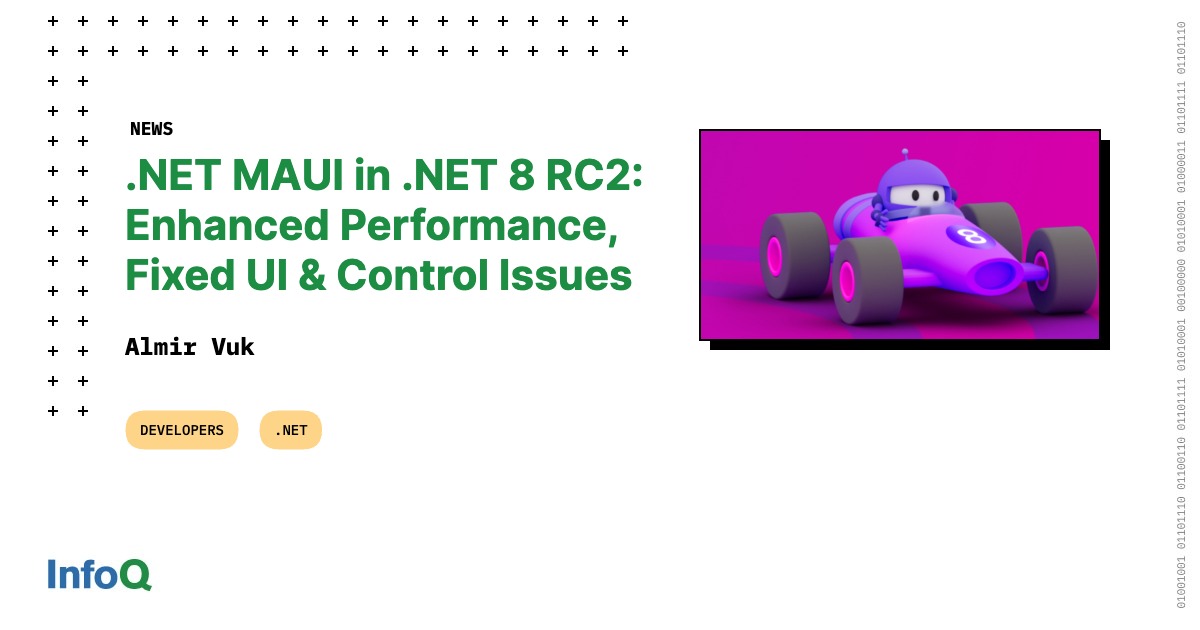
The new version of .NET MAUI has been released with .NET 8 Release Candidate 2, bringing developers closer to the general availability of .NET 8. This release, which is covered by a go-live license, focuses on resolving regression issues encountered during the preview stages. As reported, efforts have also been made to recover performance that was impacted during enhancements to features such as hot reload, visual state manager, bindings, and app themes.
In the latest Release Candidate 2 version, several significant improvements have been introduced, with the primary objectives focused on enhancing performance, fixing UI and control issues, and implementing platform-specific solutions.
Performance enhancements were a key highlight of this release, with a focus on optimising ActivityExtensions.GetWindowFrame on Android and refining Setter Specificity performance. As reported, these changes are expected to result in a smoother and more responsive app performance experience.
The development team also dedicated efforts to fixing UI and control issues, including problems related to CollectionView, TabBar visibility, RoundRectangle Borders, and Android text alignment. These updates aim to create a more consistent and visually accurate user interface across different platforms.
Furthermore, the release introduced platform-specific solutions to ensure a uniform user experience. This involved enhancements in drag-and-drop functionality, improvements in Shell tab bar appearance, and fine-tuning of specific platform behaviours.
In addition to these primary objectives, the release included numerous other bug fixes, enhancements, and contributions that aim to enhance the overall software performance. For a comprehensive overview of these changes, readers are encouraged to refer to the complete release notes.
Alongside RC 2 the .NET 7 Service Release was also released as the .NET MAUI service release 8 (version 7.0.96), targeting essential high-priority fixes in areas like layout, memory leaks, CollectionView, and safe area. Developers can access this service release by installing .NET 8 RC2, allowing them to build applications that target .NET 7. Alternatively, version 7.0.96 is available through an upgrade to Visual Studio 17.7.5.
Moreover, Xamarin developers can now utilize Xcode 15 to target the latest iOS and iPadOS versions, alongside targeting Android API 34 for policy compliance. This can be achieved by installing Visual Studio 17.8 Preview 3 or the latest stable version of Visual Studio for Mac, allowing existing projects to continue building. This flexibility is particularly beneficial for those in the process of transitioning to .NET 8 and .NET MAUI, even with the upcoming Xamarin end-of-support date next year.
Also, the comments section is active as usual. The author of the announcement post and principal product manager on .NET MAUI, David Ortinau, was a part of the communication with community members. So it is recommended that developers take a look into the conversations to gain more insights and look for community feedback.
Lastly, in addition to an original release blog post, and as part of the development process, the development team calls on developers to test the new release and share feedback through the official GitHub issue tracker. The community members are also invited to visit the official GitHub project repository and learn more about this project and its future roadmap.
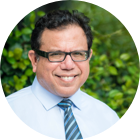
MMS • RSS
Posted on mongodb google news. Visit mongodb google news
MongoDB, Inc. (NASDAQ:MDB – Get Free Report) has been given a consensus rating of “Moderate Buy” by the twenty-six analysts that are currently covering the stock, MarketBeat.com reports. One research analyst has rated the stock with a sell rating, three have assigned a hold rating and twenty-two have given a buy rating to the company. The average 12-month price objective among brokerages that have issued ratings on the stock in the last year is $415.46.
A number of research firms have weighed in on MDB. Guggenheim raised their price target on MongoDB from $220.00 to $250.00 and gave the company a “sell” rating in a research note on Friday, September 1st. Barclays raised their target price on MongoDB from $421.00 to $450.00 and gave the stock an “overweight” rating in a research report on Friday, September 1st. Mizuho raised their price objective on shares of MongoDB from $240.00 to $260.00 in a report on Friday, September 1st. Morgan Stanley raised their price objective on shares of MongoDB from $440.00 to $480.00 and gave the stock an “overweight” rating in a report on Friday, September 1st. Finally, Piper Sandler raised their price objective on shares of MongoDB from $400.00 to $425.00 and gave the stock an “overweight” rating in a report on Friday, September 1st.
Check Out Our Latest Analysis on MDB
MongoDB Stock Up 2.4 %
Shares of NASDAQ MDB opened at $335.31 on Friday. The stock has a market cap of $23.92 billion, a P/E ratio of -96.91 and a beta of 1.13. The company has a current ratio of 4.48, a quick ratio of 4.48 and a debt-to-equity ratio of 1.29. MongoDB has a 12-month low of $135.15 and a 12-month high of $439.00. The business’s fifty day simple moving average is $357.51 and its 200-day simple moving average is $342.74.
MongoDB (NASDAQ:MDB – Get Free Report) last posted its quarterly earnings results on Thursday, August 31st. The company reported ($0.63) earnings per share (EPS) for the quarter, beating analysts’ consensus estimates of ($0.70) by $0.07. The company had revenue of $423.79 million for the quarter, compared to analyst estimates of $389.93 million. MongoDB had a negative return on equity of 29.69% and a negative net margin of 16.21%. As a group, equities analysts predict that MongoDB will post -2.17 EPS for the current fiscal year.
Insiders Place Their Bets
In other news, Director Dwight A. Merriman sold 1,000 shares of the stock in a transaction dated Friday, September 1st. The shares were sold at an average price of $395.01, for a total value of $395,010.00. Following the completion of the transaction, the director now owns 535,896 shares in the company, valued at approximately $211,684,278.96. The sale was disclosed in a legal filing with the Securities & Exchange Commission, which is accessible through the SEC website. In related news, Director Dwight A. Merriman sold 1,000 shares of the firm’s stock in a transaction that occurred on Friday, September 1st. The stock was sold at an average price of $395.01, for a total value of $395,010.00. Following the completion of the transaction, the director now directly owns 535,896 shares of the company’s stock, valued at approximately $211,684,278.96. The transaction was disclosed in a document filed with the Securities & Exchange Commission, which is accessible through the SEC website. Also, Director Dwight A. Merriman sold 6,000 shares of the firm’s stock in a transaction that occurred on Friday, August 4th. The shares were sold at an average price of $415.06, for a total value of $2,490,360.00. Following the completion of the transaction, the director now directly owns 1,207,159 shares of the company’s stock, valued at $501,043,414.54. The disclosure for this sale can be found here. Over the last quarter, insiders sold 187,984 shares of company stock worth $63,945,297. 4.80% of the stock is owned by corporate insiders.
Institutional Inflows and Outflows
Hedge funds have recently modified their holdings of the business. GPS Wealth Strategies Group LLC purchased a new stake in MongoDB during the 2nd quarter worth about $26,000. KB Financial Partners LLC purchased a new stake in MongoDB in the 2nd quarter worth approximately $27,000. Capital Advisors Ltd. LLC grew its position in shares of MongoDB by 131.0% in the second quarter. Capital Advisors Ltd. LLC now owns 67 shares of the company’s stock valued at $28,000 after purchasing an additional 38 shares during the period. Bessemer Group Inc. purchased a new stake in shares of MongoDB in the fourth quarter valued at $29,000. Finally, Global Retirement Partners LLC grew its position in shares of MongoDB by 346.7% in the first quarter. Global Retirement Partners LLC now owns 134 shares of the company’s stock valued at $30,000 after purchasing an additional 104 shares during the period. 88.89% of the stock is owned by hedge funds and other institutional investors.
MongoDB Company Profile
MongoDB, Inc provides general purpose database platform worldwide. The company offers MongoDB Atlas, a hosted multi-cloud database-as-a-service solution; MongoDB Enterprise Advanced, a commercial database server for enterprise customers to run in the cloud, on-premise, or in a hybrid environment; and Community Server, a free-to-download version of its database, which includes the functionality that developers need to get started with MongoDB.
See Also
Receive News & Ratings for MongoDB Daily – Enter your email address below to receive a concise daily summary of the latest news and analysts’ ratings for MongoDB and related companies with MarketBeat.com’s FREE daily email newsletter.
Article originally posted on mongodb google news. Visit mongodb google news