Month: April 2022
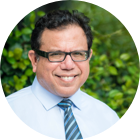
MMS • RSS
Posted on mongodb google news. Visit mongodb google news
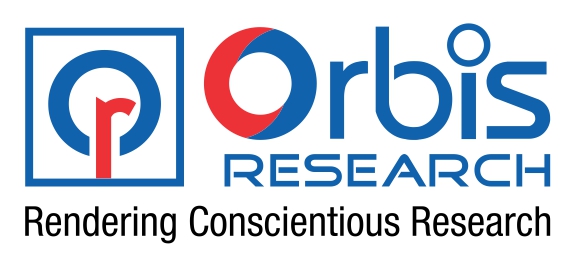
The HTAP-Enabling In-Memory Computing Technologies Market study offers an in-depth study of a wide range of characteristics and strategies, as well as potential and future trends. This market research provides a top-to-bottom assessment of the world’s major industry participants. The segmentation comes with charts, graphs, and data. This segmentation includes all aspects of the market, from manufacturing to distribution, as well as a behavioral profile of end-users. Forecasters looked into the HTAP-Enabling In-Memory Computing Technologies report to learn more about market trends and other important details. The HTAP-Enabling In-Memory Computing Technologies market study provides a thorough picture of the target market. The market value and growth rate are evaluated, as well as market size estimates.
Competition Spectrum:
Microsoft
IBM
MongoDB
SAP
Aerospike
DataStax
GridGain
…
This report examines the competitive landscape of the industry and gives information on the products offered by various companies to help clients improve their market position. Similarly, this HTAP-Enabling In-Memory Computing Technologies market research study includes information on current trends and challenges that might stymie market expansion. This will aid firms in devising strategies to optimize their potential for growth. All of the necessary components for your research inquiry are included in the study. Historical and predicted market statistics, demand, application information, price, and regional trends are all covered in the market report. The study supports and tackles a variety of significant challenges, including procurements and usage of different growth perspectives, among market participants such as end-users, companies, and distributors.
We Have Recent Updates of HTAP-Enabling In-Memory Computing Technologies Market in Sample [email protected] https://www.orbisresearch.com/contacts/request-sample/6298048?utm_source=PoojaB12
For the forecast period, the study contains market estimations and predictions in million tons by region and nation, as well as changes in market share across major geographies. The HTAP-Enabling In-Memory Computing Technologies market study looks into a variety of highly profitable industries that impact market growth. This research piece was created using primary research interviews, structured assessment, and secondary study data. The market analysis report on the HTAP-Enabling In-Memory Computing Technologies market offers thorough information on market shares, trends, and growth. The report also includes expert tips to help clients improve their implementation practices and make the best decisions possible.
The market is roughly segregated into:
• Analysis by Product Type:
Single Node Based
Distributed Systems Based
Hybrid Memory Structure Based
• Application Analysis:
Retail
Banks
Logistics
Others
• Segmentation by Region with details about Country-specific developments
– North America (U.S., Canada, Mexico)
– Europe (U.K., France, Germany, Spain, Italy, Central & Eastern Europe, CIS)
– Asia Pacific (China, Japan, South Korea, ASEAN, India, Rest of Asia Pacific)
– Latin America (Brazil, Rest of L.A.)
– Middle East and Africa (Turkey, GCC, Rest of Middle East)
Table of Contents
Chapter One: Report Overview
1.1 Study Scope
1.2 Key Market Segments
1.3 Players Covered: Ranking by HTAP-Enabling In-Memory Computing Technologies Revenue
1.4 Market Analysis by Type
1.4.1 HTAP-Enabling In-Memory Computing Technologies Market Size Growth Rate by Type: 2020 VS 2028
1.5 Market by Application
1.5.1 HTAP-Enabling In-Memory Computing Technologies Market Share by Application: 2020 VS 2028
1.6 Study Objectives
1.7 Years Considered
Chapter Two: Growth Trends by Regions
2.1 HTAP-Enabling In-Memory Computing Technologies Market Perspective (2015-2028)
2.2 HTAP-Enabling In-Memory Computing Technologies Growth Trends by Regions
2.2.1 HTAP-Enabling In-Memory Computing Technologies Market Size by Regions: 2015 VS 2020 VS 2028
2.2.2 HTAP-Enabling In-Memory Computing Technologies Historic Market Share by Regions (2015-2020)
2.2.3 HTAP-Enabling In-Memory Computing Technologies Forecasted Market Size by Regions (2021-2028)
2.3 Industry Trends and Growth Strategy
2.3.1 Market Top Trends
2.3.2 Market Drivers
2.3.3 Market Challenges
2.3.4 Porter’s Five Forces Analysis
2.3.5 HTAP-Enabling In-Memory Computing Technologies Market Growth Strategy
2.3.6 Primary Interviews with Key HTAP-Enabling In-Memory Computing Technologies Players (Opinion Leaders)
Chapter Three: Competition Landscape by Key Players
3.1 Top HTAP-Enabling In-Memory Computing Technologies Players by Market Size
3.1.1 Top HTAP-Enabling In-Memory Computing Technologies Players by Revenue (2015-2020)
3.1.2 HTAP-Enabling In-Memory Computing Technologies Revenue Market Share by Players (2015-2020)
3.1.3 HTAP-Enabling In-Memory Computing Technologies Market Share by Company Type (Tier 1, Tier Chapter Two: and Tier 3)
3.2 HTAP-Enabling In-Memory Computing Technologies Market Concentration Ratio
3.2.1 HTAP-Enabling In-Memory Computing Technologies Market Concentration Ratio (Chapter Five: and HHI)
3.2.2 Top Chapter Ten: and Top 5 Companies by HTAP-Enabling In-Memory Computing Technologies Revenue in 2020
3.3 HTAP-Enabling In-Memory Computing Technologies Key Players Head office and Area Served
3.4 Key Players HTAP-Enabling In-Memory Computing Technologies Product Solution and Service
3.5 Date of Enter into HTAP-Enabling In-Memory Computing Technologies Market
3.6 Mergers & Acquisitions, Expansion Plans
Do You Have Any Query or Specific Requirement? Ask Our Industry [email protected] https://www.orbisresearch.com/contacts/enquiry-before-buying/6298048?utm_source=PoojaB12
Key Points Covered in the HTAP-Enabling In-Memory Computing Technologies market Report:
• Comprehensive profiles of the leading firms in the HTAP-Enabling In-Memory Computing Technologies industry.
• An in-depth examination of innovation and other industry developments in the HTAP-Enabling In-Memory Computing Technologies market.
• In-depth examination of the industry’s value chain and supply chain
• A thorough examination of the primary growth drivers, limits, challenges, and development opportunities.
• Identifying and evaluating growth opportunities in key industries and economies.
Reasons to buy this Report:
• To decrease growth possibilities, hazards, and valid solutions by reviewing production techniques, major challenges, and valid remedies.
• Get an in-depth market analysis as well as a full understanding of HTAP-Enabling In-Memory Computing Technologies industry trends and the commercial landscape.
• To know production techniques, major issues, and solutions.
About Us:
Orbis Research (orbisresearch.com) is a single point aid for all your market research requirements. We have vast database of reports from the leading publishers and authors across the globe. We specialize in delivering customized reports as per the requirements of our clients. We have complete information about our publishers and hence are sure about the accuracy of the industries and verticals of their specialization. This helps our clients to map their needs and we produce the perfect required market research study for our clients.
Contact Us:
Hector Costello
Senior Manager Client Engagements
4144N Central Expressway,
Suite 600, Dallas,
Texas 75204, U.S.A.
Phone No.: USA: +1 (972)-362-8199 | IND: +91 895 659 5155
Article originally posted on mongodb google news. Visit mongodb google news
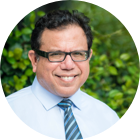
MMS • Fabio Gratz Thomas Wollmann
Article originally posted on InfoQ. Visit InfoQ
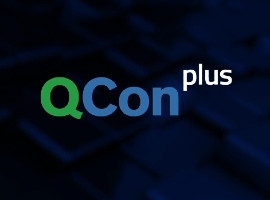
Transcript
Wollmann: Fabio and I will talk about developing and deploying machine learning across teams with our ML Ops automation tool. First of all, we will explain how Merantix Labs fits into the Merantix ecosystem, and how we do machine learning at Merantix Labs. After that, we will get into more detail what infrastructure we use for conducting deep learning projects, and how the Merantix devtool helps us to have best practices as code and infrastructure as code.
Merantix Labs
Merantix Labs is part of Merantix, which is a venture builder for AI companies in various domains. Within Merantix, we have ventures in medicine, automotive, but also in business intelligence. Merantix Labs itself is a solution provider, where we create bespoke solutions for our clients across industries and machine learning use cases.
Chameleon Ecosystem
For these very different machine learning projects, we don’t always write everything from scratch, but rely on an ecosystem with reusable components. Going from low level, which we call our tool chain for data access for ML Ops, over our platforms for specific areas of machine learning, to solutions for machine learning use cases, to actual services. Because all of these things in this hierarchy are needed for successful machine learning projects. Namely, access, having a platform for developing your models, solving your use case, and then providing the services around it to bring into production.
Chameleon Core Platform
Within that, we have for example, Chameleon, which is our computer vision platform. It serves the needs of creating, for example, models for object detection and segmentation. Our philosophy here is that we try to automate everything that can be automated for heuristics of our optimization, and make everything flexible and adaptable that can’t be automated. For example, the special needs of a machine learning model for a client. Within Chameleon, we don’t just write everything from scratch again, but rely on several packages from the open source community. I want to share a few interesting things that we use. For example for ETL, we use Apache Beam. For machine learning, we use PyTorch. For configuration management, Hydra. For our unified data format, we use Zarr, or FSSpec to have cloud agnostic data access so you’re not just tied to one cloud. Finally, for experiment tracking, we use MLflow. All of these technologies bring several requirements to our machine learning infrastructure.
Chameleon in Client Projects
How is Chameleon now used in client projects? In comparison to a product company, we have to create Chinese walls between our clients. Even if two projects have a very similar infrastructure, we have to create it over again for every client, so we have separate environments in terms of code, but also in terms of the compute resources. These reusable components like Chameleon can be used as Python packages within these projects. Custom code is injected for the project. For example, the obvious things are for data formatting or preprocessing, you’ll usually have custom code because the data of the client is always a little bit different. Also, for tackling the challenging machine learning tasks of new clients, we have a plugin system for custom models and much more.
It doesn’t end there. If you look at the whole workflow of a machine learning project with Chameleon, you also need infrastructure for uploading datasets, labeling the data, but also an experiment infrastructure so you can develop the model. When you have your model, you also need infrastructure to serve that model in your cluster with an API. You can’t be sure if the model works in production so you have to monitor it to see for example, domain shifts, but also detect attacks. For that, we need a very elaborate machine learning infrastructure that we developed at Labs.
Software Infrastructure for AI Development is Complex
Grätz: The infrastructure that we would use in a client project could look for example, like this here. We have by default, a GCP project that acts as our Chinese wall, separating different clients. We have a Kubernetes cluster in our projects that we use for model training, as well as for the deployment of models. Also, for example, a labeling tool. We have, of course, storage configured so that we can store our datasets and artifacts. We have ETL, for example, configured using Apache Beam. We need artifact stores for Docker images and Python packages. Many more things to make a project successful. The task of an engineer could be that your project starts in a week, and they need to create all this here, so that they can start working on that project. We believe in a process where the ML engineers themselves, get the tooling to manage that infrastructure instead of having a dedicated DevOps on every project team.
I’m going to give you a walkthrough now of how the process looks like that an engineer goes through to create such an infrastructure for her or his client project.
Demo
We have the MX devtool, which is a click, click, Python click CLI. The core functionality that we will talk about is to generate functionality that we adapted from Ruby, which allows us to generate certain parts of a project including a skeleton of a project using so-called generators. The engineer would start by calling, for example, the MLProjectSkeleton generator and giving a name and entering certain information that is required, like name, compute region, other engineers that will work on this project. After that, the generator that has been called generates the skeleton of this project, that includes infrastructure configuration, the documentation skeleton, the README, and the serialized state here. Where all the information that the engineer entered a second ago is serialized so that when we apply other generators in the future, the engineer doesn’t have to enter this information again.
In the infrastructure folder, there’s, for example, a Docker file, because every project has its dedicated Docker image, so that every project can have its own dependencies. One client project might require PyTorch 1.7, and another one, for some reason, the newest version of PyTorch. Every project has its own base image. There’s a Terraform configuration to create the infrastructure that I just showed you. There’s Kubernetes configuration to manage experimentation and deployment infrastructure within the Kubernetes cluster.
The next step in the workflow would be to apply this Terraform configuration to create the cluster, IAM configuration, storage buckets. To create that the engineer goes to the infrastructure Terraform directory, does a Terraform init, which I omitted here. Terraform apply, then she or he waits a few minutes. Then the outline here, the Google Cloud project, in this case, the Kubernetes cluster has been created. The Apache Beam API, for example, has been activated. Storage buckets have been created. Docker registry has been created.
The next step in the setup is that the engineer creates a GitHub repository for version control and activates CI/CD, which in a Google Cloud project is by default, cloud build. The engineer creates the repository, then to set this up, calls the so-called CloudBuildDefaultConfig generator, which adds Terraform configuration to activate cloud build. The actual manifest that tells cloud build which steps to follow, which would be building the Docker image for that project, testing the code, linting the code, publishing the documentation, pushing the Python package that comes out of this project to our pypiserver, if desired. The engineer would navigate again to the infrastructure Terraform folder, and Terraform apply to set up CI/CD.
Now that that here is done, the next thing the engineer might want to do is create the base image, the base Docker image for this project. Since cloud build CI/CD has already been configured, we just need to push to the newly created repository so that the cloud build will build the project base image. We decided that our test should only pass if the code follows our style guide. To do that, we decided to not write pages of how we want the code to look like but we incorporated all that into the devtool, so the engineers will just run mx lint on a file or an entire folder. The devtool will check all the files, whether they adhere to our style guide, and tell the engineers what needs to be changed to do so. Since this is a newly rendered project, everything is already linted, so we can initialize the Git repo. Connect it to the remote GitHub repository push, and then cloud build will run and will build the base image for the project.
Since version control and CI/CD has been configured, we have a base image that contains all the requirements for our project, we can now go to setting up the experimentation and model training infrastructure in Kubernetes. First, before the engineers use anything, they need to connect to the new project. For that there’s also functionality, again, in the devtool that configures G Cloud correctly, and also configures kubectl to have the credentials for the newly generated Kubernetes cluster. To manage deployments within the Kubernetes cluster, we use a really cool program called devspace. Devspace manages deployments, and also the development of projects on Kubernetes. We really like it. The main functionality we use is that it allows us to group certain manifests together in so-called deployments. For example, there are two here. One deployment is the training infrastructure, which includes a Kubernetes manifest for an MLflow tracking server that we want to install in our cluster. Another devspace deployment could be the CUDA drivers that we need to train models using GPUs. This devspace YAML here is a manifest file, which means that our engineers that don’t edit the file itself, but the generators that engineers execute they add the deployments, so the project skeleton generator added the train infrastructure and CUDA driver deployments. Other generators that we’ll see later will add their own deployments here.
The engineers then call devspace deploy, training infrastructure CUDA drivers, this will take a minute to run. After that, there is an MLflow tracking server running in the cluster that has a Postgres database configured to store experiment metadata and metrics. The MLflow tracking server has been configured to store model artifacts into a Google Cloud bucket that was created when we first call Terraform apply on the newly rendered project.
Now, the engineers following the few steps that we have seen, install the training infrastructure so we can start training a model. To train models we use MLflow with a Kubernetes backend. I don’t want to go too much into detail because we mostly follow the default MLflow setup. There is an MLproject file that allows us to group different project entry points, for example, the trainer. The trainer.py here that is rendered by the project skeleton generator is really just a minimal working example there. There is not much logic in here, but it runs, and it can be configured using Hydra and the default configuration here.
MLflow to run with a Kubernetes backend requires a train_job.yaml that is also part of the default project skeleton generator. Everything we need from the MLflow side is here. We need to connect our localhost 5000, which was the default URI for MLflow from the cluster to our dev machine. We chose to not expose it due to security reasons, but we opened a Kubernetes port forward, which we wrapped inside mx flow connect so that our engineers don’t have to run kubectl port forward, service name every time. Once that port is forwarded, our engineers can run mx flow run, choose the trainer entry point, and the Kubernetes backend just like you would do with MLflow run. Mx flow run is really a light wrapper around MLflow run that sets some environment variables correctly and configures where to reach the tracking server basically.
I’ve shown you how we get from no infrastructure at all to configuring cloud infrastructure using Terraform, installing training infrastructure into the Kubernetes cluster using devspace. Then using MLflow to start a training. This box here is filled. What happens, for example, when the engineers want to do distributed training on their cluster, what do they need to do? There is a generator for that. The engineer would navigate back to the root directory of the project, and call the distributed training infrastructure generator. The distributed training infrastructure generator adds a new train job template for MLflow. This is not a default Kubernetes job, but it’s a Kubeflow PyTorch job manifest. The generator adds one new section to the devspace.yaml. This section here includes a manifest that installs the kubelet PyTorch operator. The good thing is that engineers don’t have to know every detail about how all this is set up, because they don’t need to configure it from scratch. They just read in the documentation which generator they have to call, and which devspace or Terraform command they need to apply so that this manifest here gets installed into the cluster. They can now start distributed training using MLflow and the custom plugin that we build to handle this distributed job manifest.
We have training, experimentation, infrastructure now. I showed you how an engineer can quickly render or create the infrastructure that is required for distributed training. We do the same things for every other component. Let’s say for example, we have a model now and we want to deploy it for a client, or we want to deploy a labeling tool, or the Streamlet app for a milestone meeting to showcase a model. For that we use Istio, which is a service mesh and Seldon Core for model deployment. To install that into the cluster, the engineer would again, run the generator, in this case the deployment infrastructure generator. This requires a Terraform apply, because it creates a static IP for the cluster, it creates DNS records that map a domain that is already configured for this client project to that static IP. Then we can use in devspace install Istio, the service mesh that we use, and Kubernetes manifest that configures an ingress to the cluster. They wait a few minutes, and the domain name is already configured, HTTPS was configured, the certificate was configured, the engineers don’t have to worry about that. Everything is rendered using these generators.
Engineers can then deploy the infrastructure, for example, for Seldon Core, into the cluster. Seldon Core is a Kubernetes library for model serving and model monitoring that we really like. It’s very powerful, and the engineers have to follow only these steps here to install the infrastructure that is required into the cluster to make this work. Same goes for a labeling tool. Let’s say the engineers are in the beginning of a project not on the deployment phase, and client data that has been uploaded needs to be labeled. They run the CVAT labeling tool generator. In this case, the CVAT labeling tool generator will also render the configuration for the service mesh and the ingress, because to expose the labeling tool, we need that tool. Since we already did apply it here, we don’t need to apply it again. Otherwise, engineers would have to, but we can now directly proceed to deploying the CVAT manifest using devspace, the labeling tool that’s installed into the cluster. Since we already configured the ingress, we can reach the labeling tool at labeling.clientname.merantixlabs.com, for example. The engineers don’t have to worry about static IP for the cluster. They don’t have to worry about managed certificates for that domain. They don’t have to worry about these details, because they just render the configuration using the generators.
This is how we created, for example, the service mesh now, labeling tools, deployed models. I didn’t go into detail how the Streamlet app would be deployed, but you do the same thing. There is a generator that gives you a skeleton. The engineers run that generator and have a skeleton with the Kubernetes manifest that are required to deploy it. Also, I didn’t talk about documentation. We use Sphinx for documentation, and there is also devtool command, and xbuild docs to build the documentation. Using a generator, we can also configure cloud build in a way that this is automatically deployed every time we push the master on GitHub.
I gave you an overview now over how an infrastructure looks like for a typical client project at Labs. The important part that I wanted to bring across here is the way that we automate the management of this infrastructure. Because to us, it is important that we don’t need a DevOps engineer on every single client project, but that we build tooling that enables our machine learning engineers to manage the infrastructure themselves.
DevTool – Your ML Project Swiss Army Knife
Wollmann: We showed you how the devtool is our Swiss Army knife for spinning up infrastructure in our client projects. It serves infrastructure as code, so we can be reproducible, we make everything still maintainable, and also traceable. This can be all done by the machine learning engineers and data scientists themselves. They can spin up their infrastructure in the project and manage it without having a DevOps always at hand. We do this also by using project templates, which has several benefits. First of all, you have a common structure of the projects, which makes it easier for an engineer to get into a new project. We can use it to preserve learnings across different projects. Moreover, we can use the tool to have best practices as code, because nobody likes to read, for example, long style guides for code. All these repetitive tasks for checking these style guides and also other repetitive tasks within your workflow can be automated.
We try to make our tool fun to use for other engineers, so they use it voluntarily. Moreover, we didn’t just build that devtool for ourselves, so we made it extendable because we are in the Merantix ecosystem. There’s a core component which saves you all these functionality for so-called generating templates that we just showed. Different ventures within the Merantix ecosystem can write their own extensions as plugins for their own operations. Hopefully today, we could inspire you a bit how ML Ops at Labs works. Maybe it gave you some ideas for your own operations.
Questions and Answers
Jördening: How many fights did you have about concurrent Terraform applies from machine learning engineers working on the same project?
Grätz: There’s a lock. As long as people don’t force unlock it, that’s ok.
Jördening: You have a convention on how to write the locks too.
Grätz: Terraform does that when one engineer calls the Terraform apply. One important point is we synchronize the Terraform state in the cloud bucket, so then everybody has a lock. As long as they don’t force unlock it, nothing happens.
Jördening: The locks are probably then semantically generated from your project names, so that you have a unique name for each project?
Grätz: Yes.
Jördening: We already had the question regarding the K8s native versus cloud native. What is your tradeoff, especially since you’re working together with customers, you probably sometimes have someone saying, GCS is not really our preferred cloud provider, or is it ok for all your customers?
Grätz: We are a GCP partner, so we like to work with Google Cloud. It’s true that, especially like small and medium enterprises in Germany, they start and say, yes, but only Azure. We can only use Azure, for example. Working with Kubernetes is great for us, because then we can tell them, ok, that works for us, please give us access to a managed Kubernetes service that runs in your infrastructure, and we’ll take it from there. This is why Kubernetes is really great for us.
Jördening: How do you do it if you share the machine learning models between different customers? Because it seems like you have VCS for every project running on a separate GKE cluster? Is there some synchronization you have between the VCS, or how do you avoid the big, do not repeat yourself?
Wollmann: Most of our clients prefer that they are completely isolated from our other clients. There are just a few components that we share to not repeat ourselves. One is, for example, Chameleon to build computer vision models, which are complete like task agnostic, it’s more like a set of things to ease your work and automate what can be automated. This is distributed through a pypiserver, where we have the central storage and the different projects can read from it, from this pypiserver and install things, and the same with more elaborate stuff, which has been, for example, in Docker containers. It’s not that we have lots of reads and writes between the projects, we try to separate them. This was also a motivation for the devtool and this templating because we have to create the same infrastructure multiple times, without doing everything manually. Copy pasting Terraform files gets infeasible at some point.
Jördening: What benefits do you see of building such an environment as opposed to getting an off-the-shelf ML Ops tool with all the elements?
Grätz: Let’s say we build on Google’s Vertex AI, or in SageMaker. Then the next client comes to us and say, but we have to do it on Azure for internal reasons. Then we are completely vendor locked and would have a hard time fulfilling the contract with them, because we rely on a certain platform from another cloud provider. That’s one argument.
I think the other one is that it’s very powerful if you can manage these things yourself, because you’re not locked in by what certain managed solutions offer. That being said, I also don’t want to make managed solutions sound bad. I think managed solutions are great, and you should use them wherever you can. Since this is the core of our business, I think for us having the control here is also important.
Jördening: Regarding control, you said that you basically manage the domain for the ingresses in every project itself. How do you manage the access there? Do you buy a domain for every project, because sharing domain is a sensitive topic, I feel?
Grätz: The main goal for us was that deploying a labeling tool for a client project would be easy. Not every data scientist or machine learning engineer knows exactly how DNS records work. They don’t want to maybe go through the hassle with certificates so that the labeling tool, for example, is TLS secured. This had to be automated in some way, so they do just deploy few things, maybe call Terraform apply here, a devspace deploy there and then you wait until it’s there basically. The way we do it is that we have one domain which is merantixlab.com. That we bought, of course. Then there are subdomains that are for the individual projects. When you create the deployment generator, it creates Terraform configuration that creates a static IP address for the cluster. It creates DNS records that map that subdomain. We own the domain, and the subdomain is mapped onto that static IP, so the engineer doesn’t have to worry about that. Then there is Kubernetes configuration rendered, that creates or configures an ingress object that knows that the static IP exists. It will use it. It will use a managed certificate where the domain has already been rendered into. Everything is there, the engineer just has to apply these configurations using Terraform, and then Kubernetes. Then wait a little. It takes a few minutes to propagate. Everything is there beforehand, and the data scientist doesn’t really have to know until it breaks, of course.
Wollmann: This is done in Google Cloud. We have this zero trust principle. We have a proxy in the front that separates the access from the different clients between the deployments, even if they are under a single domain. This keeps it secure, at least for these tasks.
Jördening: I was more thinking about basically, if you deploy one project, and you have access to the domain that you could redirect some other traffic.
If you have the environment, you have your machine learning code, how long does it then take you to get the product ready for your customers, in terms of man hours? You can put it on a critical time path.
How long it took to get one product released, so from getting all the infrastructure up to having probably an endpoint serving it.
Grätz: Our machine learning projects are very custom, so we might have some that take two weeks, we might have some that take half a year. The infrastructure setup takes an hour or so, tops, with runtime. Getting the infrastructure ready for deployment also takes very little time. What happens in between there, there’s lots of custom development happening usually and that can have very different time spans.
Was the question rather targeted to how long it took us to get to this point where the infrastructure can be managed that quickly?
Jördening: It’s for getting a product ready. It was about the tool.
Grätz: We started a year ago.
Wollmann: It was more like an iterative process. We built the first prototype, then tested if it’s like sticky with the engineers, if they liked it also in terms of usability. Then extended the functionality, especially of the templates to add more stuff that can be automatically templated and deployed. Recently, we worked this whole system to also make this adaptable to different kinds of projects so it’s less static, because in some project, you need some of the functionalities, in some you don’t. For example, with this generator system, you are very flexible. We looked at different solutions, tried different things until we got to the final thing. We started the waterfall development, and now it’s there. It was more like an iterative thing.
Jördening: Is this open source or how can I try this?
Wollmann: Primarily, we built this for ourselves to speed up our workflows and to automate whatever we can. We figured out that this is maybe also interesting for others, so that’s why we also presented now to a larger audience to see if there’s interest. We are currently thinking about how to get a larger user base hands-on on it. In the Merantix ecosystem, we already created it to have it modular to also edge things, according to the different Ops of ventures, because not every company operates the same.
Jördening: Where do your data scientists prototype? I assume they prototype in the K8s cluster where you will deploy in the end this flow, or do you have a separate prototyping environment?
Grätz: We use MLflow to schedule trainings. In MLflow you can have different backends, you can have a local one that runs in a con environment, you can have a local Docker environment, and you can have a Kubernetes environment. You can write plugins for your own environment, basically. The engineers we hired, they typically know Docker. We pay attention to that in the hiring process because we use it everywhere. The engineers locally, they will develop in a Docker container that acts as Python’s runtime. They attach that to their IDE, then they schedule a training on the cluster. The training runs on the cluster. We have custom plugins for distributed training in Kubernetes that use components of Kubeflow.
What we also find really great is that devspace, which I can really recommend to everyone working with Kubernetes, has a dev mode that synchronizes files to your cluster and back. We have like a debug pod configured that has every dependency that the base image of the project has, including JupyterLab and some other tools you might need for debugging. Then you can, in the terminal, just write devspace dev deployment debug pod, and that will spin up this pod running JupyterLab in the cluster, and you get the file synchronized back and forth. You change the notebook you have it on your computer, immediately. You drop an image into the folder on your dev machine, you have it in the cluster immediately. It’s like having a GPU on your laptop.
See more presentations with transcripts
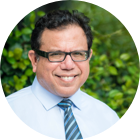
MMS • RSS
Posted on nosqlgooglealerts. Visit nosqlgooglealerts
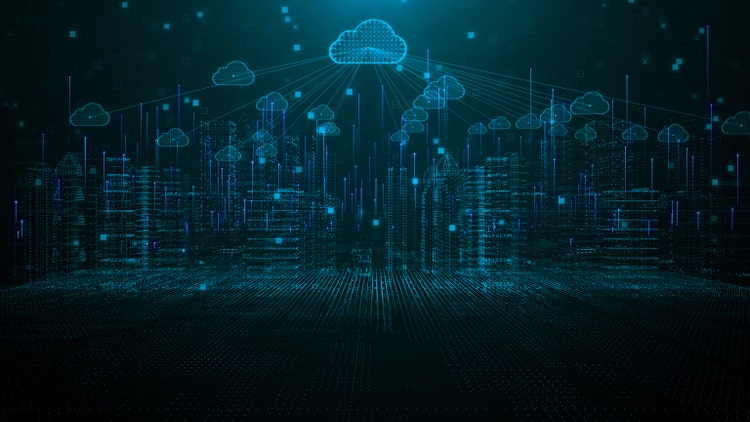
KanawatTH/iStock via Getty Images
Two years ago, I left a role managing foundation and endowment portfolios to launch a software service targeting professional service providers who work with F&E clients. Over the course of 2 to 3 years, I taught myself web development, product management, and software infrastructure.
You might think: “That’s a little strange for an investment manager to leave the field and start building software.” You might be right. But I’m sure it will become more and more common.
We are at a point where the tools for developers are so good that a single individual with no formal education in software development can build, deploy, and manage a subscription software service on their own.
Part of the reason this is possible is because of companies like MongoDB (NASDAQ:MDB).
Data Persistence For Young Companies
When I was educating myself on how I would build my product, the vast majority of tutorials that I came across touted the benefits of using NoSQL for data persistence, like MongoDB (the open-source product, not the company) in place of traditional SQL databases. So I learned it.
I’m glad that I did because there is no way I could move as fast as I do with an SQL database. For those who don’t use databases every day, MongoDB allows developers to make on the fly modifications to the structure of their documents. If I suddenly realize that I need five extra fields describing each of my users, adding them is no problem. This flexibility is vital because budding companies have no idea what their data structures should look like out of the gates. This flexibility is something you just don’t get with SQL solutions.
On top of that, MongoDB documents are stored in a format that is already native to the language of the web: JSON. When I need a record, I call it from the database, and it’s immediately ready for me to process and present to my users. This saves me dozens, maybe hundreds of development hours.
MongoDB, the open-source software, creates a fantastic developer experience – especially for young companies, where the primary constraint is capital and access to developer time.
What The User Base Means For MongoDB, Inc.’s Growth
MongoDB, Inc. hasn’t been around for a long time. The first version was launched just a bit over a decade ago, and its popularity has taken off within just the last five years.
To understand this, all you have to do is look at a chart of the “mongodb” package downloads on NPM (a registry that aggregates open-source packages for javascript developers).
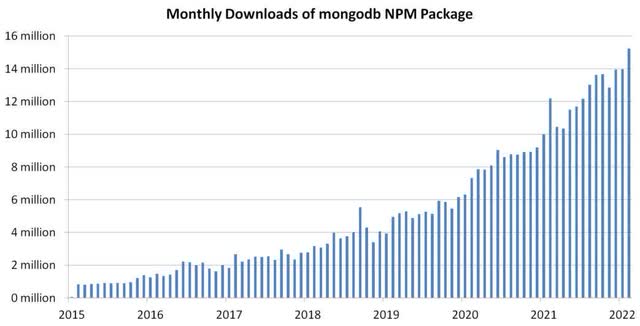
Open-source downloads of MongoDB on NPM (NPM)
Because big, profitable, established products don’t just decide to migrate database architecture, it’s likely that the growth the open-source project has seen over the last five years is concentrated in young companies and new developers who aren’t heavily invested in the SQL ecosystem.
It’s important to remember that the company doesn’t directly capture revenue from users of the open-source MongoDB project. MongoDB, Inc.’s revenue is derived from users & companies who have products that warrant paying for the speed, reliability, and security that Cloud Database Hosting (the Atlas Product) and Enterprise Services provide.
New projects with little to no revenue can’t afford to pay much for cloud hosting services. But as these projects mature, their user base grows, and income starts coming in. Suddenly, getting fast, reliable database hosting becomes essential. The vast majority of new software projects fail, but those that do succeed typically take 24-36 months to achieve revenue sustainability. Because of this, MongoDB, Inc. will see a natural lag in its revenue relative to its open-source database software growth.
According to the International Data Corporation (IDC), the data management software market will be ~$121 billion by 2025. SQL-based solutions currently dominate this market, and as the shift towards NoSQL occurs, MongoDB (the company) is perfectly positioned to capture market share. Today, MongoDB represents less than 1% of the market. There is a lot of room for them to capture spend.
Analyst Growth Estimates
According to Tikr.com, analysts are projecting about 33% compound annual revenue growth for MongoDB, Inc out to January 2025. This is coming off of a sustained period of hyper-growth; see table below.

Revenue Growth at MongoDB (MongoDB 10K)
While I don’t question that revenue growth will slow, 33% revenue growth over the next three years feels far too pessimistic. Let’s look at what would need to occur to get there. Note I’m only looking at services revenue (Atlas & Enterprise Services), which makes up 95% of the company’s revenue.
- Enterprise Services growth would need to collapse to 5%
- The Atlas product would need to fall to 40% growth during a period when downloads of the open-source project (see chart above) have shown no signs of slowing and when we should be expecting user projects initiated over the last 2-3 years to become revenue-generating for MongoDB, Inc.

Analyst Growth Expectations Path (Tikr.com)
These assumptions strike me as more of a bear case than a reasonable base case. Suppose we rework the table, assuming that enterprise customers don’t fall to stable growth over the next three years and that Atlas can retain some semblance of its current momentum (in what appears to be a favorable environment for the product). In that case, you don’t have to stretch too far to see an opportunity for 40% growth out to 2025.
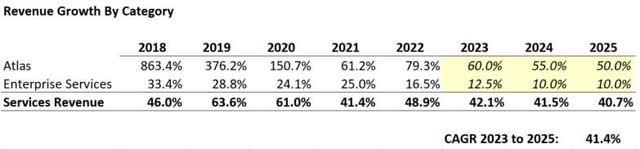
Tweaking MongoDB Growth Estimates (Personal Estimates)
Valuation
From reading the comments and other writeups on MDB, it’s clear that people have trouble getting behind a stock trading at 20x forward revenue.
This viewpoint disregards the speed at which MongoDB is growing and how quickly this valuation will come back to earth after baked-in growth is realized. Let’s look at what happens if the stock appreciates by 7% over the next five years.
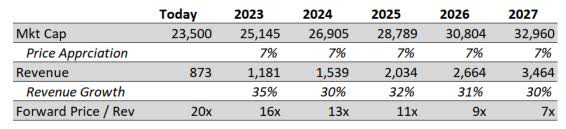
MongoDB Price/Revenue Decline (Personal Estimates)
Very quickly, Price/Rev falls back to a multiple that is much more in line with what you would expect to see in the industry.
Industry And Stock Pullback
There’s no question that tech stocks have fallen out of favor recently, the NASDAQ 100 is down over 25% from its highs in late 2021, and MDB itself is down nearly 40%.
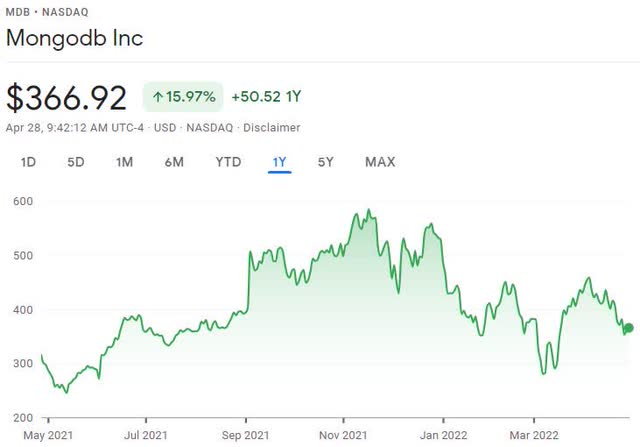
MongoDB Price Chart (Google Finance)
I won’t try to opine on what will happen to tech stocks generally over the next 12-24 months, and if the sector does poorly, MDB will likely follow suit.
What I will say is that MongoDB, Inc. is putting out fantastic open-source software and providing the infrastructure necessary so that companies can quickly and easily run that software in a production environment at scale.
Summary
MongoDB, Inc.’s open-source database is a boon to software developers and young companies. It makes product development faster and less expensive than traditional databases. The software development community is catching on, and usage of the tool is accelerating quickly.
The hosting service that MongoDB, Inc. operates becomes essential for products that reach viability, which we will start to see more of following the explosive growth of the tool.
While the company is richly valued at 20x forward revenue, it seems likely that the market is pricing in growth estimates on the low end of what is likely to occur.
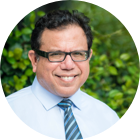
MMS • RSS
Posted on mongodb google news. Visit mongodb google news
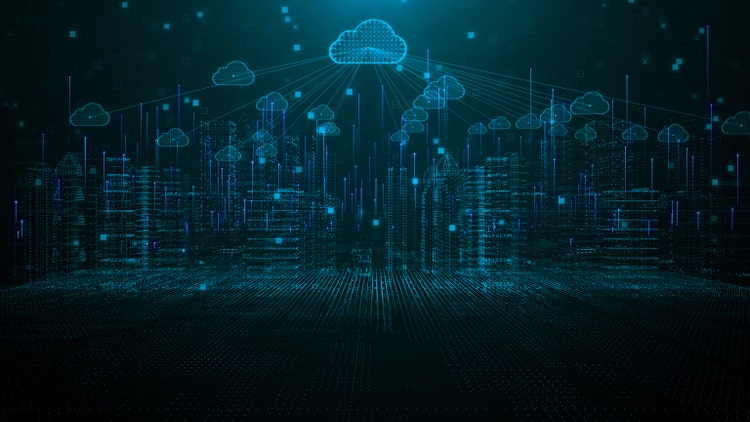
KanawatTH/iStock via Getty Images
Two years ago, I left a role managing foundation and endowment portfolios to launch a software service targeting professional service providers who work with F&E clients. Over the course of 2 to 3 years, I taught myself web development, product management, and software infrastructure.
You might think: “That’s a little strange for an investment manager to leave the field and start building software.” You might be right. But I’m sure it will become more and more common.
We are at a point where the tools for developers are so good that a single individual with no formal education in software development can build, deploy, and manage a subscription software service on their own.
Part of the reason this is possible is because of companies like MongoDB (NASDAQ:MDB).
Data Persistence For Young Companies
When I was educating myself on how I would build my product, the vast majority of tutorials that I came across touted the benefits of using NoSQL for data persistence, like MongoDB (the open-source product, not the company) in place of traditional SQL databases. So I learned it.
I’m glad that I did because there is no way I could move as fast as I do with an SQL database. For those who don’t use databases every day, MongoDB allows developers to make on the fly modifications to the structure of their documents. If I suddenly realize that I need five extra fields describing each of my users, adding them is no problem. This flexibility is vital because budding companies have no idea what their data structures should look like out of the gates. This flexibility is something you just don’t get with SQL solutions.
On top of that, MongoDB documents are stored in a format that is already native to the language of the web: JSON. When I need a record, I call it from the database, and it’s immediately ready for me to process and present to my users. This saves me dozens, maybe hundreds of development hours.
MongoDB, the open-source software, creates a fantastic developer experience – especially for young companies, where the primary constraint is capital and access to developer time.
What The User Base Means For MongoDB, Inc.’s Growth
MongoDB, Inc. hasn’t been around for a long time. The first version was launched just a bit over a decade ago, and its popularity has taken off within just the last five years.
To understand this, all you have to do is look at a chart of the “mongodb” package downloads on NPM (a registry that aggregates open-source packages for javascript developers).
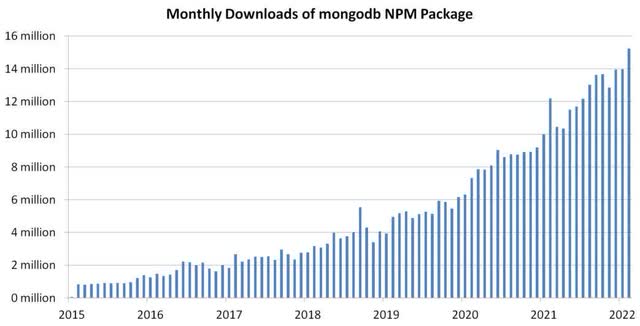
Open-source downloads of MongoDB on NPM (NPM)
Because big, profitable, established products don’t just decide to migrate database architecture, it’s likely that the growth the open-source project has seen over the last five years is concentrated in young companies and new developers who aren’t heavily invested in the SQL ecosystem.
It’s important to remember that the company doesn’t directly capture revenue from users of the open-source MongoDB project. MongoDB, Inc.’s revenue is derived from users & companies who have products that warrant paying for the speed, reliability, and security that Cloud Database Hosting (the Atlas Product) and Enterprise Services provide.
New projects with little to no revenue can’t afford to pay much for cloud hosting services. But as these projects mature, their user base grows, and income starts coming in. Suddenly, getting fast, reliable database hosting becomes essential. The vast majority of new software projects fail, but those that do succeed typically take 24-36 months to achieve revenue sustainability. Because of this, MongoDB, Inc. will see a natural lag in its revenue relative to its open-source database software growth.
According to the International Data Corporation (IDC), the data management software market will be ~$121 billion by 2025. SQL-based solutions currently dominate this market, and as the shift towards NoSQL occurs, MongoDB (the company) is perfectly positioned to capture market share. Today, MongoDB represents less than 1% of the market. There is a lot of room for them to capture spend.
Analyst Growth Estimates
According to Tikr.com, analysts are projecting about 33% compound annual revenue growth for MongoDB, Inc out to January 2025. This is coming off of a sustained period of hyper-growth; see table below.

Revenue Growth at MongoDB (MongoDB 10K)
While I don’t question that revenue growth will slow, 33% revenue growth over the next three years feels far too pessimistic. Let’s look at what would need to occur to get there. Note I’m only looking at services revenue (Atlas & Enterprise Services), which makes up 95% of the company’s revenue.
- Enterprise Services growth would need to collapse to 5%
- The Atlas product would need to fall to 40% growth during a period when downloads of the open-source project (see chart above) have shown no signs of slowing and when we should be expecting user projects initiated over the last 2-3 years to become revenue-generating for MongoDB, Inc.

Analyst Growth Expectations Path (Tikr.com)
These assumptions strike me as more of a bear case than a reasonable base case. Suppose we rework the table, assuming that enterprise customers don’t fall to stable growth over the next three years and that Atlas can retain some semblance of its current momentum (in what appears to be a favorable environment for the product). In that case, you don’t have to stretch too far to see an opportunity for 40% growth out to 2025.
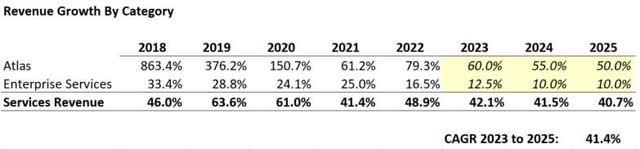
Tweaking MongoDB Growth Estimates (Personal Estimates)
Valuation
From reading the comments and other writeups on MDB, it’s clear that people have trouble getting behind a stock trading at 20x forward revenue.
This viewpoint disregards the speed at which MongoDB is growing and how quickly this valuation will come back to earth after baked-in growth is realized. Let’s look at what happens if the stock appreciates by 7% over the next five years.
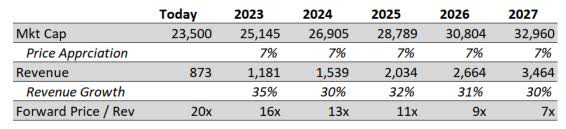
MongoDB Price/Revenue Decline (Personal Estimates)
Very quickly, Price/Rev falls back to a multiple that is much more in line with what you would expect to see in the industry.
Industry And Stock Pullback
There’s no question that tech stocks have fallen out of favor recently, the NASDAQ 100 is down over 25% from its highs in late 2021, and MDB itself is down nearly 40%.
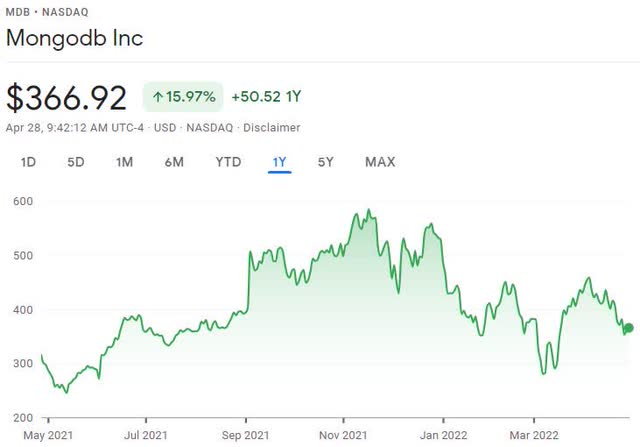
MongoDB Price Chart (Google Finance)
I won’t try to opine on what will happen to tech stocks generally over the next 12-24 months, and if the sector does poorly, MDB will likely follow suit.
What I will say is that MongoDB, Inc. is putting out fantastic open-source software and providing the infrastructure necessary so that companies can quickly and easily run that software in a production environment at scale.
Summary
MongoDB, Inc.’s open-source database is a boon to software developers and young companies. It makes product development faster and less expensive than traditional databases. The software development community is catching on, and usage of the tool is accelerating quickly.
The hosting service that MongoDB, Inc. operates becomes essential for products that reach viability, which we will start to see more of following the explosive growth of the tool.
While the company is richly valued at 20x forward revenue, it seems likely that the market is pricing in growth estimates on the low end of what is likely to occur.
Article originally posted on mongodb google news. Visit mongodb google news
NoSQL Database Market Promising Growth Opportunities & Forecasts 2028 – Tiorienteering –
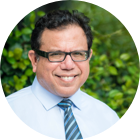
MMS • RSS
Posted on nosqlgooglealerts. Visit nosqlgooglealerts
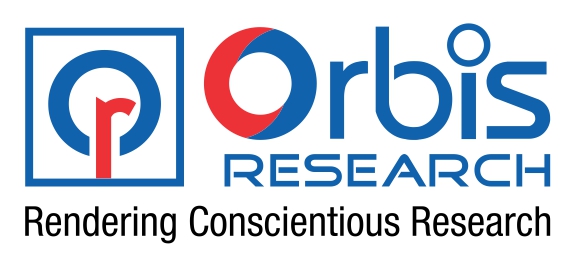
The report is developed to give investors a window into NoSQL Database Market and reveals opportunities for intervention at various points of NoSQL Database market supply chain. The report discusses each and every aspect of the NoSQL Database market supply chain including marketing, imports, exports, distribution, production, etc. The report covers the main products lines of the NoSQL Database market and focuses on the discussion of those products lines that are leading the NoSQL Database market and contributing majorly to its growth. The NoSQL Database market report studies the performance of the NoSQL Database industryly by considering different scenarios.
Crucial references pertaining to the competition spectrum, identifying lead players have been well incorporated in this research report.
DynamoDB
ObjectLabs Corporation
Skyll
MarkLogic
InfiniteGraph
Oracle
MapR Technologies
he Apache Software Foundation
Basho Technologies
Aerospike
Technologies that have enhanced efficiency and are driving growth in the market are studied in the report. The disruptive market forces, growth avenues, innovative business models, regulatory environment of the NoSQL Database industry, current trends in 2021, regulatory issues, and important updates that firms operating in the market should be addressing are analyzed in the study. Importantly, the report studies the market products and services along with the segments and sub-categories that are well-positioned for growth in the year ahead. Moreover, recommendations for the market participants to stay competitive are offered through the report.
The key regions covered in the NoSQL Database market report are:
North America (U.S., Canada, Mexico)
South America (Cuba, Brazil, Argentina, and many others.)
Europe (Germany, U.K., France, Italy, Russia, Spain, etc.)
Asia (China, India, Russia, and many other Asian nations.)
Pacific region (Indonesia, Japan, and many other Pacific nations.)
Middle East & Africa (Saudi Arabia, South Africa, and many others.)
The NoSQL Database Industry research report offers important information on the market as well as helpful insight and guidance for people and businesses interested in the market. This research will also aid a number of significant corporations in identifying their competitors and acquiring a foothold in the market. The NoSQL Database sector is expected to grow quickly.
We Have Recent Updates of NoSQL Database Market in Sample [email protected] https://www.orbisresearch.com/contacts/request-sample/6320878?utm_source=PoojaB12
• Segmentation by Type:
Column
Document
Key-value
Graph
• Segmentation by Application:
E-Commerce
Social Networking
Data Analytics
Data Storage
Others
The NoSQL Database market is divided into three sections: Type, Region, and Application. The segment growth provides exact estimates and predictions for sales by Type and Application in terms of value and volume. Furthermore, this study offers a detailed forecast of the market by product type, application, and region. It also includes sales and revenue projections for the whole projection period. With industry-standard precision in analysis and better data integrity, the research report makes a significant effort to reveal major prospects available in the market to assist organizations in obtaining a strong market position.
Place Inquiry for Buying or Customization of [email protected] https://www.orbisresearch.com/contacts/enquiry-before-buying/6320878?utm_source=PoojaB12
Buyers and providers of research may gain access to verified and reliable market projections, such as those for the entire size of the market in terms of revenue and volume. The NoSQL Database market prediction was generated using an in-depth market investigation completed by a number of industry specialists.
About Us:
Orbis Research (orbisresearch.com) is a single point aid for all your market research requirements. We have vast database of reports from the leading publishers and authors across the globe. We specialize in delivering customized reports as per the requirements of our clients. We have complete information about our publishers and hence are sure about the accuracy of the industries and verticals of their specialization. This helps our clients to map their needs and we produce the perfect required market research study for our clients.
Contact Us:
Hector Costello
Senior Manager Client Engagements
4144N Central Expressway,
Suite 600, Dallas,
Texas 75204, U.S.A.
Phone No.: USA: +1 (972)-362-8199 | IND: +91 895 659 5155
NoSQL Database Market Major Strategies Adopted By Leading Market Companies – themobility.club
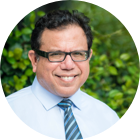
MMS • RSS
Posted on nosqlgooglealerts. Visit nosqlgooglealerts
The Global NoSQL Database Market report offers industry overview including definitions, applications, classifications, and chain structure. The report provides a comprehensive assessment of the studied market, including key trends, historic data, current market scenario, opportunities, growth drivers, potential roadmap, and strategies of the market players. The report further includes regional analysis to evaluate the global presence of Baby Car Seat Market.
In order to simplify the industry analysis and forecast estimation for the NoSQL Database Market, our research report delivers well-defined market scope and systematically developed research methodology.
Access Sample Report – marketreports.info/sample/21207/NoSQL-Database
Global NoSQL Database Market: Segment Analysis
Each segment of the studied market is comprehensively evaluated in the research study. The segmentation analysis discussed in the report presents key opportunities available in the market through leading segments. Following are the segments discussed in the report:
Regional Analysis:
The global NoSQL Database Market is segmented as The regional segmentation of the market includes North America (U.S. & Canada), Europe (Germany, United Kingdom, France, Italy, Spain, Russia, and Rest of Europe), Asia Pacific (China, India, Japan, South Korea, Indonesia, Taiwan, Australia, New Zealand, and Rest of Asia Pacific), Latin America (Brazil, Mexico, and Rest of Latin America), Middle East & Africa (GCC, North Africa, South Africa, and Rest of Middle East & Africa).
The Following are the Key Features of Global NoSQL Database Market Report:
- Market Overview, Industry Development, Market Maturity, PESTLE Analysis, Value Chain Analysis
- Growth Drivers and Barriers, Market Trends & Market Opportunities
- Porter’s Five Forces Analysis & Trade Analysis
- Market Forecast Analysis for 2022-2030
- Market Segments by Geographies and Countries
- Market Segment Trend and Forecast
- Market Analysis and Recommendations
- Price Analysis
- Key Market Driving Factors
- NoSQL Database Market Company Analysis: Company Market Share & Market Positioning, Company Profiling, Recent Industry Developments etc.
Key Players:
This section of the report includes a precise analysis of major players with company profile, market value, and SWOT analysis. The report also includes manufacturing cost analysis, raw materials analysis, key suppliers of the product, mergers & acquisitions, expansion, etc. Following companies are assessed in the report:
DynamoDB, Aerospike, ObjectLabs Corporation, MarkLogic, InfiniteGraph, Skyll, he Apache Software Foundation, Oracle, Basho Technologies, MapR Technologies
By Type
Column
Document
Key-value
Graph
By Application
E-Commerce
Social Networking
Data Analytics
Data Storage
Others
Check For Instant Discount- marketreports.info/discount/21207/NoSQL-Database
Why Market Reports?
- We use latest market research tools and techniques to authenticate the statistical numbers
- Availability of customized reports
- Expert and experienced research analysts in terms of market research approaches
- Quick and timely customer support for domestic as well as international clients
Purchase Full Report @ marketreports.info/checkout?buynow=21207/NoSQL-Database
About Us:
Market Reports offers a comprehensive database of syndicated research studies, customized reports, and consulting services. These reports are created to help in making smart, instant, and crucial decisions based on extensive and in-depth quantitative information, supported by extensive analysis and industry insights.
Our dedicated in-house team ensures the reports satisfy the requirement of the client. We aim at providing value service to our clients. Our reports are backed by extensive industry coverage and is made sure to give importance to the specific needs of our clients. The main idea is to enable our clients to make an informed decision, by keeping them and ourselves up to date with the latest trends in the market.
Contact Us:
Carl Allison (Head of Business Development)
Market Reports
phone: +44 141 628 5998
Email: sales@marketreports.info
Website: www.marketreports.info
Distributed NoSQL database Aerospike adds support for JSON • The Register – TheRegister
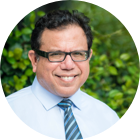
MMS • RSS
Posted on mongodb google news. Visit mongodb google news
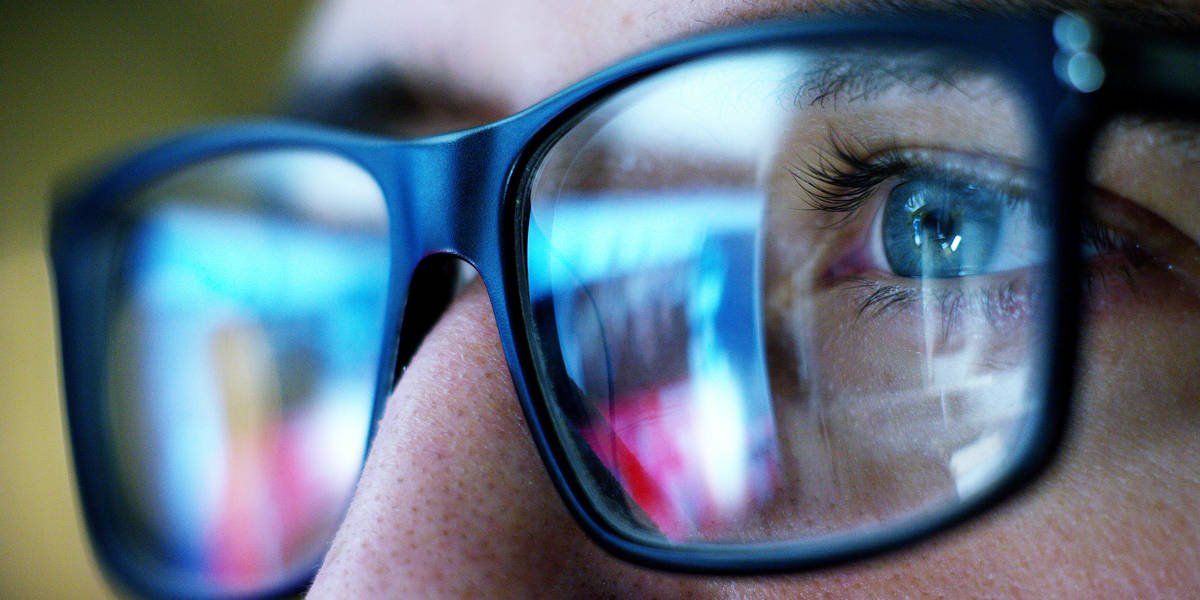
Distributed NoSQL database Aerospike has added support for JSON documents to a slew of new features included in its Database 6 release.
The value-key database has established its niche by touting high throughput, low latency and global scalability. It is adding support for the document format in a bid to broaden use cases and take on document database specialists Couchbase and MongoDB.
Lenley Hensarling, chief product officer, said some customers were supporting as many as 13 billion transactions per day on Aerospike. Support for JSON document models, the Java programming models, and JSONPath query would help users store, search, and better manage complex data sets and workloads.
“Many times, we replace the standard solutions in this space due to their inability to maintain consistent predictable performance as the data scales, but perhaps more importantly the number of concurrent transactions scales.
Hensarling added: “There starts to be a wider variance in the latency as one scales across one or more of these vectors. That variance winds up affecting the Service Level Agreements in response times, decisioning windows, and transactions.
“While there are a number of ‘document’ data stores, there are very few that can deal with the true variance in loads that the internet presents. Aerospike handles that. Now enterprises needn’t rewrite their JSON / JSONPath applications to gain that advantage,” he told The Register.
Holger Mueller, vice president, Constellation Research, said some enterprises would commonly use Aerospike and a separate document DB, such as MongoDB or Couchbase. “If both are needed in one application, it gets complicated. So having one database, one license, and one performance to manage leads to simplifications.
“Aerospike has become bigger and better: it has an impressive innovation track record and it has become a multimodal database player while keeping its high performance,” he said.
However, the company was “still small”, Mueller said. “They will need to deliver to become the trusted partner of more large corporations – this is an important step.”
While Aerospike focuses on low latency — and is used in adtech and fraud detection — document databases have been focused on the type of data users can store.
They have not stood still either. Last year Couchbase launched its 7.0 release which offers multi-statement SQL transactions and an approach to building schema-like structures into the database, allowing it to support multiple applications from the same data.
Aerospike can support SQL — the more or less universal query language. Users have to employ the Aerospike Connect for Presto, which works with Presto/Trino data mesh SQL solutions, to give SQL access to the data in Aerospike Clusters. ®
Article originally posted on mongodb google news. Visit mongodb google news
Distributed NoSQL database Aerospike adds support for JSON • The Register – TheRegister
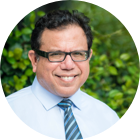
MMS • RSS
Posted on nosqlgooglealerts. Visit nosqlgooglealerts
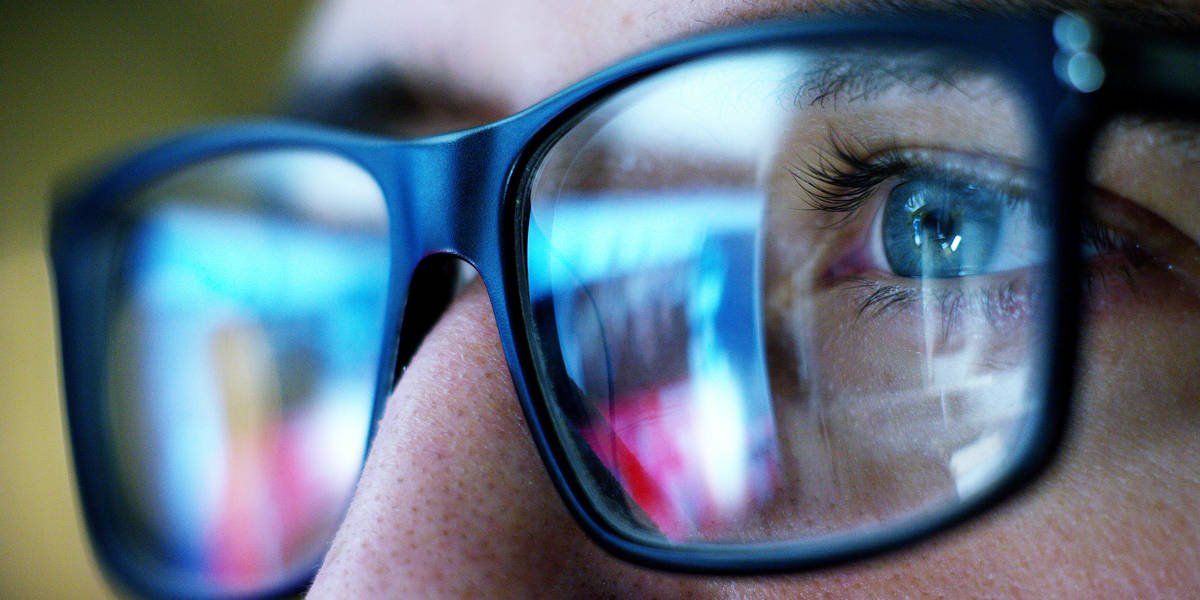
Distributed NoSQL database Aerospike has added support for JSON documents to a slew of new features included in its Database 6 release.
The value-key database has established its niche by touting high throughput, low latency and global scalability. It is adding support for the document format in a bid to broaden use cases and take on document database specialists Couchbase and MongoDB.
Lenley Hensarling, chief product officer, said some customers were supporting as many as 13 billion transactions per day on Aerospike. Support for JSON document models, the Java programming models, and JSONPath query would help users store, search, and better manage complex data sets and workloads.
“Many times, we replace the standard solutions in this space due to their inability to maintain consistent predictable performance as the data scales, but perhaps more importantly the number of concurrent transactions scales.
Hensarling added: “There starts to be a wider variance in the latency as one scales across one or more of these vectors. That variance winds up affecting the Service Level Agreements in response times, decisioning windows, and transactions.
“While there are a number of ‘document’ data stores, there are very few that can deal with the true variance in loads that the internet presents. Aerospike handles that. Now enterprises needn’t rewrite their JSON / JSONPath applications to gain that advantage,” he told The Register.
Holger Mueller, vice president, Constellation Research, said some enterprises would commonly use Aerospike and a separate document DB, such as MongoDB or Couchbase. “If both are needed in one application, it gets complicated. So having one database, one license, and one performance to manage leads to simplifications.
“Aerospike has become bigger and better: it has an impressive innovation track record and it has become a multimodal database player while keeping its high performance,” he said.
However, the company was “still small”, Mueller said. “They will need to deliver to become the trusted partner of more large corporations – this is an important step.”
While Aerospike focuses on low latency — and is used in adtech and fraud detection — document databases have been focused on the type of data users can store.
They have not stood still either. Last year Couchbase launched its 7.0 release which offers multi-statement SQL transactions and an approach to building schema-like structures into the database, allowing it to support multiple applications from the same data.
Aerospike can support SQL — the more or less universal query language. Users have to employ the Aerospike Connect for Presto, which works with Presto/Trino data mesh SQL solutions, to give SQL access to the data in Aerospike Clusters. ®
Article: Design-First Approach to API Development: How to Implement and Why It Works and How to Implement It
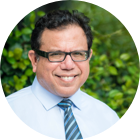
MMS • Steve Rodda
Article originally posted on InfoQ. Visit InfoQ
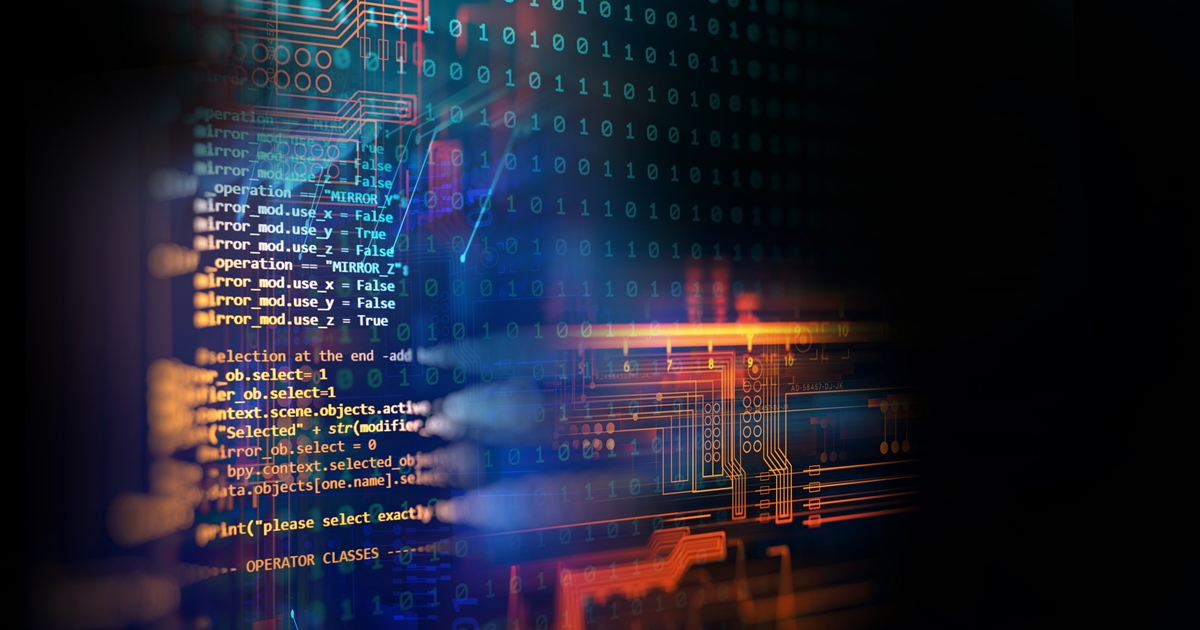
Key Takeaways
- Identify which approach to your API program makes the most sense for your team and the benefits/drawbacks of each approach.
- The most important thing is to ensure positive experiences for all stakeholders including the end-user, the developer and the company (a design-first approach and customer-centric language is key to this).
- Viewing your API as a product saves time, expands revenue opportunities, increases collaboration and innovation.
- A focus on decentralized governance, increased automation and consistency through style guides will improve your API program by minimizing complexity for API consumers.
- There are guidelines and patterns for taking a design-first approach to API development
Let’s think about the world’s greatest masterpieces for a second.
Shakespeare’s “Hamlet.” DaVinci’s “Mona Lisa.” Frank Lloyd Wright’s architectural beauty that is “Fallingwater.” Vivaldi’s “Four Seasons” concerto. I could go on and on, and if it were up to me, I would include the world’s best pick-up truck ever created, as well. But, I digress.
All of these masterpieces have one thing in common: they were built with the design first in mind. You can’t build a house without a blueprint, and you can bet DaVinci didn’t just wing it on the “Mona Lisa.”
Starting with a design isn’t a new idea, and the world’s greatest creators have practiced it throughout generations, but having a “design-first” approach to Application Programming Interfaces (API) is.
Huh? You may ask yourself. Let me explain!
Thanks to the explosion of the digital economy, there are currently 27 million software developers in the world, and that number is expected to reach well over 45 million by 2030. Today, 19 million of those developers are API developers, and the Application Development Software Market Size is projected to grow 196% by 2027. Now, I don’t know about you, but I would consider that a significant market.
With this rapid growth of the API industry, it follows that more developers and technology leaders both need to know how to make these efforts successful. Increasingly, folks in these essential roles need to understand what it takes to build out a solid, successful, scalable API program that will drive business value.
Where do you start? What are the most important factors to consider?
There is complexity for sure, but I’m here to tell you that the most important factor to consider is getting started with a design-first approach to your API strategy. As developers, we can have the next API masterpiece at our fingertips; we just have to design it first.
Approaches to Your API Strategy
Okay, so you have an excellent idea for an API that will benefit the business, but what next?
You could start by writing out the business requirements for the API and then proceed to code it. Or, perhaps you decide first to describe your API in a specification language, such as OpenAPI. You could even convince your organization to take your idea and turn it into an API-first product. Which is it?
Let’s go through the most common approaches — Code-First, API-First, or Design-First — and review some pros and cons of each:
- Code-First: When a developer needs to deploy an API fast, going right to coding after receiving the business requirements will speed up API deployment. Sometimes, this is the quickest option if the API has only a few endpoints. Code-first, in a nutshell, is completely oriented to developers and unconcerned about other potential API users.
The problem with a code-first approach is that even well-designed APIs developed this way are predestined to fail because the success of an API is based on its adoption and use by more and more users. As adoption trends up, most of your new users won’t be developers or technically-minded people — they will just be people trying to solve problems as quickly and efficiently as possible. If an API is not intuitive and applicable to their context, the average person simply won’t use it.
- API-First: This is an increasingly promoted approach. Basically, it means that your organization treats APIs as the core focus with the understanding that they are critical business assets upon which the organization operates. This process is initiated with a contract written in an API description language such as OpenAPI. There’s nothing wrong with this way, and it’s wise to standardize early across a platform or set of products. The problem is that the chosen language and its particulars often dominate and even limit a company’s ability to scale and build for the future. If your API is the most important thing, what does that say about all the people developing it and/or consuming it?
- Design-First: This approach fundamentally means any API effort — whether one or many in a program — starts with a design process. In this model, APIs are defined in an iterative way that both humans and computers can understand — before any code is ever written. The goal is that every team speaks the same language, and every tool they use leverages the same API design. The crucial difference here compared to an API-first approach is that, while the API is incredibly important, the design process is what ensures all stakeholders are involved, and their needs are satisfied in the creation.
Design-First begins with both technical and non-technical individuals from each of the functions involved participating in the process of writing a contract that defines the purpose and function of the API (or set of APIs). Obviously, this approach requires some time upfront spent on planning. This phase aims to ensure that when it comes time to start coding, developers are writing code that won’t need to be scrapped and rewritten later down the line. This helps create iterative, useful APIs that, in turn, lead to a better, more scalable API program — and value to your business — as a whole.
Regardless of which approach you choose, the most critical thing to think about is how to deliver positive experiences for stakeholders, including end-users, third-party or in-house developers, and even folks from the rest of the company who may have a role. I think of APIs like technology ambassadors — the digital face of a brand — as they form a network of internal and external connections. And as such, they should be designed and crafted with care, just like any other product or service that your company offers.
Successful API programs engage users early and keep them involved in the design process to ensure development tracks with users’ expectations. This step is equally important for internal and external APIs. The users involved in the process are likely to become the early adopters who will initially drive acceptance and use of the product.
The Benefits of a Design-First Approach to API Development
‘But, Steve! (you may be asking) How can this approach benefit developers, end users, internal partners, and more?’ Great question. Let’s start with the most valuable API development asset: developers.
- Improved Developer Experience: There are numerous benefits to the Design-First approach but by far one the most important, and (dare I say ‘buzziest’), is creating a positive developer experience. As someone who came up through the developer path myself, I can tell you that being tasked with fixing poorly written APIs is a nightmare.
Without intentional design, the development process can become chaotic, clunky, and disjointed. It creates disconnects between the developers, security, governance, and documentation teams. The end result is vast amounts of pressure heaped on the developers’ shoulders to push an API all the way to the end.
The API specs, governance, design, development, and documentation start from the same page and are developed and maintained simultaneously by taking a design-first approach. This built-in coordination keeps developers focused on developing solutions and prevents them from slowing down due to API clean-up work. Design-first is developer-friendly, keeping them engaged and focused on the end result, not distracted or delayed by poorly-written and inconsistent APIs. Ultimately, happy developers = a happy API program.
- Engineering Efficiency and Cost Savings: When quality components are developed and maintained using a design-first approach, they can be reused for future APIs. You only need to build each component once, saving a ton of time and money for your technology team. Reusability for all components allows for significant cost savings in development time while also getting new APIs to market faster.
- Improved API Security: Although APIs have blossomed in the past few years, that also makes them a prime target for hackers and malware due to their visibility and reputation for having design flaws and vulnerabilities. Without a proper design in place, exposed API endpoints can be easily exploited by hackers. As a technology leader, security is always a top concern of mine, and intentional design ensures that security is built into your API strategy from the ground up.
- Better Coordination Among Internal Teams: Large and cross-functional teams are notoriously hard to coordinate, and it’s even more of a challenge to bring additional stakeholders in midstream and keep everyone on the same page. With this approach, relevant stakeholders are involved from the outset and can build their input into developing the API. Including all stakeholders, even those who may be non-technical users of that API ensures that the API is designed to be inclusive and meet all possible needs.
- Increased Innovation & Growth Opportunities: APIs are catalysts for growth, increased innovation, and improved user experiences. It’s easier than you may expect to go from an expensive, inefficient process to something that works better for your developers, customers, and bottom line. Over 40% of large companies today report utilizing 250 or more APIs, according to the Developer Survey 2020 report, and that opportunity for continued growth will only rise. It is estimated that 2000 new public APIs are published daily.
With how prolific and widespread APIs have become, they now touch virtually every industry, sector, and location in the world. As the designated integration point between technologies (by name!) APIs are prevalent in all kinds of companies, from car manufacturers to healthcare. For example, one of our largest customers is a global beer manufacturer. I’m going to “go out on a limb” and say: that’s not the first company you would think of as a leader in API design and development.
We know that it’s no joke that many of the most significant opportunities for innovation — across industries and the public and private sector — are through APIs. That is why it’s critical they be designed correctly to scale with that growth.
Okay, But does Design-First Actually Work?
I get it; you may not want to take my word that design-first is a game-changer for your API program, but here are a few stories that speak for themselves.
Transact, is a campus services technology company. They cut 80% of their API development time by adopting the design-First approach to their API program. (Remember that time efficiency and cost savings I mentioned previously? Voila.)
“Even better than the reduced development time is the increased collaboration with other teams when we use the design-first approach, we get feedback earlier and the result ends up being more polished and professional,” shared Paul Trevino, Senior Engineering Manager at Transact.
Another, Calendly, the meeting scheduling software (which I’m not shy to admit I use constantly), has an API team that built an entirely new API platform using a design-first approach.
“The design-first approach, for us, leads to a higher-quality implementation, consistency, governance, and documentation that’s never out of date. That’s something that definitely speaks to developers both producing and consuming the API; documentation that’s never out of date and that’s high quality, detailed, all that good stuff that you expect from a mature platform,” shared Calendly’s Dmitry Pashkevich, an Application Architect.
How to Implement a Design-First Approach
Alright, so you’ve stuck with me this long, which means you’re probably ready to hear how you can implement one of these fancy Design-First approaches to your own organization’s API strategy. Focusing on decentralized governance, increased automation, and consistency through a tool such as style guides will lead you down a design-first path of success and improve your API program by minimizing complexity for API consumers.
Start With Consistency & Good Governance
They say if you have nothing else to your name or reputation, at least you can have consistency. There’s a reason a company like Starbucks does so well: it is because we know what to expect. There is comfort in having a predictable experience, no matter where in the world you are when you walk into one.
What is true for real-world human interfaces such as Starbucks is also true for APIs. It is essential to keep the design of an API consistent — to make it a predictable experience. As we say here at Stoplight, consistency is the primary difference between a bunch of APIs and something that feels like one cohesive platform.
To enforce consistency and keep that thoughtful design going, implementing guidelines into your API strategy will make it possible to enforce standards. API style guides are easily consumable instructions for all relevant information that a developer or team of developers will need to create or work with APIs. Style guide references typically provide a basic understanding of the API(s), what they are, and best practices for how to create, use, and enforce them at your organization.
Along with that consistency comes a need for strong governance of your API program. Standardization will soon fall to the wayside if your program is not designed with API governance in mind. API governance is all about applying rules and policies to your APIs, such as descriptions, contracts, designs, protocols, reviews, etc. This standardization in the designing of your API helps ensure that all your APIs remain consistent even when different developers design and build them. An effective governance program helps organizations ensure that every API is high-quality and discoverable — whether they create a few APIs or a few thousand.
View Your APIs as Products
The next step in applying the design-first approach is to make sure you are viewing your APIs as products. API-as-a-Product is an established and increasingly popular software development concept, which essentially just means that you’re operating your API with a lens that it’s just like any other product someone consumes or uses. APIs, like products, should be easy-to-use and actively maintained and supported.
The process of API design includes the planning and architectural decisions you’d make for a product. Just like product design, API design informs the user experience, and good design principles meet initial expectations and continue to behave consistently and predictably.
Gaining Stakeholder Buy-In
One of the most important parts of implementing a design-first approach is gaining the favor of all relevant stakeholders (which is, again, the primary point of operating from a design-first approach). Creating the foundation for your API program means that you need developer buy-in, executive buy-in, feedback from early end-users, and the opinions of any other partners or internal folks that may interact with the API.
My recommendation to woo the business leaders is to focus on (probably not surprisingly) the business value, ideally, including solid metrics and proof-of-concept. Even if you only have minimal data to show off at first, take them up the chain and show constant improvement over time. The momentum will speak for itself as you get the ball rolling. Once you have that buy-in, think about how to parlay this mandate into transforming the organization and what key players are needed to make it happen.
To woo the developers, create a safe space that they feel comfortable voicing their opinions in and a space to test out what will work (with a set of guidelines, of course). Find stakeholders with relational clout within a development organization and establish a way to collaborate with them on your API strategy (best enabled through an API Design tool, I might shamelessly add). Your API program team must be a multidisciplinary one, with a wide range of experiences, use cases, and skills.
Lastly, be sure and get feedback from early adopters and end-users (throughout) about the actual experience of consuming the API. Keep in mind that if it’s not used, it’s not useful, so it needs to be a positive experience. Developers can sometimes lose sight of that end-user experience, but a design-first approach ensures that this is always top of mind.
So, Did I Convince You?
I appreciate you joining me in delving into the design-first discovery. I hope you can now see how it is a linchpin for ensuring a sound, robust, and easily scalable – or, heck, your masterpiece – API and even API program. (And, if you choose not to heed my warning, here’s what happens when you don’t follow a design-first approach.)
So, if you need a guide to get you going, I strongly recommend (though I am biased) that you check out Stoplight’s resources around API design.
Overall, we know APIs are taking the world by storm, and nearly everyone is looking for a way to build successful programs to innovate and grow their business. By now, I hope you can see that you won’t create an API masterpiece if you don’t design first.
Personally, I like to think that developers are some of the world’s best designers and creators, and I can’t wait to see what gets created next with the design-first approach in mind.
Exclusive Interview with Akshay Soam, Chief Technology Officer, Seracle – Analytics Insight
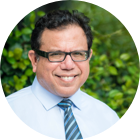
MMS • RSS
Posted on mongodb google news. Visit mongodb google news
With lots of fans and critics, blockchain, with its apps and blockchain development tools are growing in popularity to unimaginable heights. It is becoming the buzzword where even a normal company changing its name and business model according to the blockchain hype is leading to higher profits. Founded in 2018, Seracle enables developers to develop and create apps on blockchain very fast, with the full support of the Seracle blockchain experts. Analytics Insight has engaged in an exclusive interview with Akshay Soam, Chief Technology Officer, Seracle.
1. What was the business problem? How was it identified and by whom?
Founded in 2018, Seracle enables developers to develop and create apps on blockchain very fast, with the full support of the Seracle blockchain experts. Seracle has quickly grown to become more than a USD $100 million business employing over 100 staff and with offices across India, Singapore, Thailand, and Canada. We work with a wide range of industries, in banking, agriculture, and travel which are increasingly reliant on blockchain technology. Over the last two years, during the pandemic, we have seen a remarkable uptake in the number of people investing in crypto, as well as the use of blockchain tech by other organizations hence keeping pace with this newfound demand, was an important element. This is where our technical team identified some significant issues.
As an early-stage start-up, we built a lot of our crypto and web 3.0 infrastructure on our own, using the services of a cloud provider that offered basic capabilities. We knew that we would need to handle and prioritize a wide variety of data types and hence, chose MongoDB as our core database which we managed ourselves. But our team of developers quickly needed extra scalability to support the business’ strong growth. We also needed to overcome the challenges that came with having to manage growing data complexity so we could continue to improve performance and reliability.
While an average day would see about 3,000 users on the Seracle platform, this number could go up to between 12,000 and 18,000 new registrations per day following marketing campaigns. It was important our developers could scale up and down on-demand to manage transaction volumes. The development team also had to continually make changes and release updates to keep up with the quickly evolving Crypto landscape. While this was all possible with our existing infrastructure, it required a large investment in developer time and more capital resources.
We also had an important analytics and visualization challenge: getting a holistic picture of all up-to-date data was a pain. We were using Kibana and had to sync all our different data sources into ElasticSearch, just to generate reports. This was time-consuming and created other problems like multiple copies of data and the risk the data would be out of date by the time it was shared. The syncing issues often caused downtime and would make queries inefficient.
Finally, Seracle’s initial data infrastructure didn’t allow for different sets of data from different projects, each having different privacy and security requirements based on each country or region, to be aggregated in a single database. As more customers from a wide range of countries were onboarded, we required extra security capabilities and built-in compliance for every conceivable geographic region, but it couldn’t compromise the customer experience.
2. How did you and your team settle on this solution to the business problem?
Once we had identified the problems, we started figuring out ways to resolve these and make our system much more reliable and efficient.
In our quest to be able to support scaling up of clients, we upgraded to MongoDB Atlas, the fully managed cloud database service. With MongoDB Atlas, our developers no longer had to worry about the infrastructure and management of the database – think scaling, security, optimization, etc. MongoDB manages all of that in the backend and enables our developers to focus on building and releasing applications. It saves time and cost for us as a company. We migrated away from Digital Ocean to host MongoDB Atlas on AWS, which provided the company with extra flexibility and scalability. We currently heavily rely on AWS, and the ability to have MongoDB via AWS was a big selling point.
MongoDB Charts have helped us solve the BI problem we were facing. Charts are built on a drag and drop interface, so users can perform custom queries and doesn’t require data movement or the use of a querying language. Once a data source is selected in Charts, updates happen in real-time, so syncing is a non-issue even across lots of different data sets.
3. What was the build/creation process like? How long did it take? Were there any hiccups or surprises?
We recently migrated one of our marquee customers- to Atlas. In all, the entire migration process took about 40-50 days. The migration was a perfect opportunity for us to revisit its cloud layer and overcome the flexibility and scalability limitations of our cloud provider and we also moved to AWS. The second stage of the migration included the move to MongoDB Charts for analytics.
We were handheld by MongoDB through the entire build /creation process and this made the whole process of migration as well as implementation seamless. The team at MongoDB, hosted one-on-one sessions with our technical team to better understand our architecture and gave us suggestions on how to improve our platform. The always-on support from people who know the database inside and out gave us a lot of added value. Developers can now focus on what matters the most: driving innovation and supporting customers instead of just managing the database and issues such as queries, sync, security, compliance, and more. Together, the MongoDB platform, MongoDB Atlas and Charts are helping drive forward one of the most modern and exciting areas of technology, pushing the boundaries of what our platform can offer.
4. What was the most challenging aspect for you (as CIO) and how did you address that challenge?
Working with data has always been the hardest part of building and evolving applications. And for us, performance and reliability are key.
MongoDB has provided us with the flexibility to move fast and simplify how we build data for any application. With MongoDB’s application data platform, our team can use one interface, for any application, and run it anywhere. This provides us with resilience, nearly infinite scalability, and best-in-class security, with multi-cloud deployment flexibility. The MongoDB Atlas platform has helped us immensely in operational tooling and management while freeing up developer time. Another important aspect is the charts, which have added huge value for us by enabling better and easier analytics and access by having everything in one place.
5. What were the results? Has the project transformed your business in unexpected ways? Has it led to identifying additional opportunities?
We now have a more efficient trading mechanism and can now go beyond 15000 trades per sec with no trouble. This directly translates to better performance and cost savings for Seracle’s clients. Customers are able to make more money because they can process more transactions. The analytics element also results in the creation of more targeted products and a better understanding of customer needs. Compared to Kibana, MongoDB Atlas’s Charts can more easily and quickly handle a large – and exponentially growing – number of data sets. Atlas on AWS provides us with extra flexibility and scalability. That means the company can scale up and down to respond to any promotional activity or anything in a particular region without having any issues or downside.
We can also now more easily control where and how data is accessed. This could be stored on local servers or separated data into distinct clusters and we can do this with a few clicks. So now we can comply with different regional rules more easily, whether that’s European customers that need to comply with GDPR or with APAC customers that also have their own specific compliance requirements.
6. Any advice or best practices you’d like to share with other CIOs that may be facing similar business challenges or undertaking similar projects?
Well, first I have to say to tech leaders if you’re looking for crypto or blockchain services, there are world-class solutions out there that can radically transform how you do business. Just call me! Working with MongoDB helps ensure we can offer industry-leading reliability, robustness, and security.
It makes more sense to plug into an already built solution like Seracle, than build the whole tech stack from the ground up. Companies can reduce costs as well as save precious time in terms of Go-to-Market by using an already existing solution. Doing what we have done from scratch, building your own infrastructure would take at least 3 years and over 40 developers. Thanks to MongoDB we were able to build what we’ve built much faster, and with fewer developers. We plan to onboard more customers in 2022 and will continue relying on MongoDB to power our growth as well as sustain its innovation efforts in an industry that is fast growing.
It is better to focus on your areas of expertise and strengths and leave the rest to the other experts as we have done with databases and MongoDB.
Article originally posted on mongodb google news. Visit mongodb google news